jvm garbage collection algorithm
We all know that the biggest difference between Java language and C language is automatic memory recycling. So how does JVM control memory recycling? This article will introduce several algorithms of JVM garbage collection to understand the basics of memory recycling. principle.
stop the world
Before introducing the garbage collection algorithm, we need to first understand the word "stop the world", stop the world will Occurs when executing a certain garbage collection algorithm. In order to perform garbage collection, the JVM will temporarily execute the Java application and wait until the garbage collection is completed before continuing to run. If you have used JMeter to test a Java program, you may find that during the test, the Java program has irregular pauses. In fact, this is "stop the world". During the pause, the JVM is doing garbage collection. Therefore, reducing the time to stop the world as much as possible is our main goal in optimizing the JVM. Next, let’s take a look at what common garbage collection algorithms are currently available.
Reference counting method
The reference counting method, as the name suggests, counts the number of times an object is referenced. When a reference count is increased, the reference counting method is increased. 1. Decrementing the reference count by one reduces it by 1.
The above figure shows that three Teacher references point to the Teacher object in the heap, then the reference count of the Teacher object is 3 , and so on, the reference count of the Student object is 2.
##The above figure shows that the reference of the Teacher object is reduced to 2. The reference of the Student object is reduced to 0 (the reason for the reduction is that the reference points to null, such as teacher3=null). According to the reference counting algorithm, the memory space of the Student object will be reclaimed.
The principle of the reference counting algorithm is very simple. It is the most primitive recycling algorithm, but this algorithm is not used in Java. There are 2 reasons. 1. Frequent counting affects performance, and 2. Unable to handle circular reference issues.
For example, the Teacher object references the Student object, and the Student object references the Teacher object. In this case, the object will never be recycled.
Mark clearing
Mark clearing algorithm, which is the basis of many garbage collection algorithms. Simply put, there are two steps: marking and clearing .
Mark: Traverse all GC Roots and set objects reachable from GC Roots as living objects;
Clear: Traverse the heap All objects in , objects that are not marked as reachable will be cleared;
Pay attention to the gray objects in the above picture, because They cannot be traversed from the GC Root (although they themselves have reference relationships, they cannot be traversed from the GC Root), so they are not marked as alive objects and will be recycled during the cleanup process.
It should be noted here that during the execution of the mark clearing algorithm, "stop the world" will be generated, allowing the Java program to pause and wait to ensure that during the mark clearing process, there will be no new object is generated. Why must the java program be paused? For example, if a new object is generated after the marking process is completed, and the object has missed the marking period, then in the subsequent cleanup process, the newly generated object will be regarded as unmarked because it has not been marked. If the unreachable object is cleared, the program will error. Therefore, when the mark clearing algorithm is executed, the Java program will be suspended, resulting in "stop the world".
Next we summarize the mark clearing algorithm:
1. Because it involves a lot of memory traversal work, the execution performance is low. It will also lead to a longer "stop the world" time and reduced Java program throughput;
#2. We noticed that after the object is cleared, the cleared object leaves a vacancy in the memory. , causing memory discontinuity and waste of space.
Next let’s see if other algorithms can improve these problems?
Mark compression
Mark compression algorithm You may have thought of it. It is based on the mark removal algorithm and adds a compression process.
After the mark clearing is completed, the memory space is compressed to save memory space and solve the problem of mark clearing algorithm memory. Discontinuity problem.
Note that the mark compression algorithm will also produce "stop the world" and cannot be executed concurrently with the Java program. During the compression process, the memory addresses of some objects will change, and the Java program can only wait for the compression to complete before continuing.
Copy algorithm
The copy algorithm simply divides the memory into two parts, but only uses one of them during garbage collection. , copy the surviving objects in the memory being used to another blank memory, and finally clear the objects in the memory space being used to complete garbage collection.
Replication algorithm relative mark The compression algorithm is more concise and efficient, but its shortcomings are also obvious. It is not suitable for situations where there are many surviving objects, because there are many objects that need to be copied, and the copy performance is poor. Therefore, the copy algorithm is often used in the new generation in the memory space. Garbage collection, because there are fewer surviving objects in the new generation and the copy cost is lower. Another disadvantage is its high memory space occupation cost, because it copies objects based on two memory spaces, and only uses one memory space during the non-garbage collection cycle, resulting in low memory utilization.
Summary
Above we have introduced common garbage collection algorithms. Each of these algorithms has its own advantages and disadvantages, but they are not Instead of simply using a specific algorithm, you use something called a garbage collector. The garbage collector can be seen as a different combination of a series of algorithms. Only by using the appropriate garbage collector in different scenarios can you get twice the result with half the effort. . Our next article will introduce the garbage collector.
Reference materials: "Practical Java Virtual Machine" Ge Yiming"In-depth Understanding of Java Virtual Machine Machine (2nd Edition)》Zhou Zhiming
##
The above is the detailed content of jvm garbage collection algorithm. For more information, please follow other related articles on the PHP Chinese website!

Hot AI Tools

Undresser.AI Undress
AI-powered app for creating realistic nude photos

AI Clothes Remover
Online AI tool for removing clothes from photos.

Undress AI Tool
Undress images for free

Clothoff.io
AI clothes remover

Video Face Swap
Swap faces in any video effortlessly with our completely free AI face swap tool!

Hot Article

Hot Tools

Notepad++7.3.1
Easy-to-use and free code editor

SublimeText3 Chinese version
Chinese version, very easy to use

Zend Studio 13.0.1
Powerful PHP integrated development environment

Dreamweaver CS6
Visual web development tools

SublimeText3 Mac version
God-level code editing software (SublimeText3)

Hot Topics
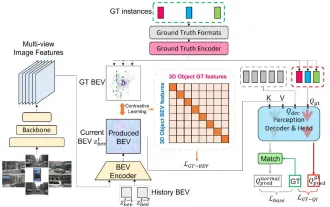
Written above & the author’s personal understanding: At present, in the entire autonomous driving system, the perception module plays a vital role. The autonomous vehicle driving on the road can only obtain accurate perception results through the perception module. The downstream regulation and control module in the autonomous driving system makes timely and correct judgments and behavioral decisions. Currently, cars with autonomous driving functions are usually equipped with a variety of data information sensors including surround-view camera sensors, lidar sensors, and millimeter-wave radar sensors to collect information in different modalities to achieve accurate perception tasks. The BEV perception algorithm based on pure vision is favored by the industry because of its low hardware cost and easy deployment, and its output results can be easily applied to various downstream tasks.
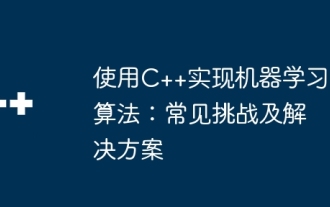
Common challenges faced by machine learning algorithms in C++ include memory management, multi-threading, performance optimization, and maintainability. Solutions include using smart pointers, modern threading libraries, SIMD instructions and third-party libraries, as well as following coding style guidelines and using automation tools. Practical cases show how to use the Eigen library to implement linear regression algorithms, effectively manage memory and use high-performance matrix operations.
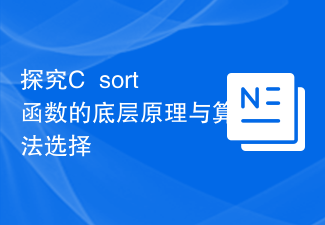
The bottom layer of the C++sort function uses merge sort, its complexity is O(nlogn), and provides different sorting algorithm choices, including quick sort, heap sort and stable sort.
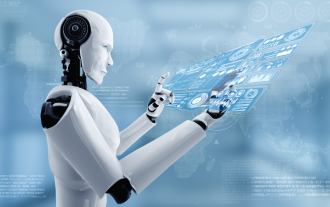
The convergence of artificial intelligence (AI) and law enforcement opens up new possibilities for crime prevention and detection. The predictive capabilities of artificial intelligence are widely used in systems such as CrimeGPT (Crime Prediction Technology) to predict criminal activities. This article explores the potential of artificial intelligence in crime prediction, its current applications, the challenges it faces, and the possible ethical implications of the technology. Artificial Intelligence and Crime Prediction: The Basics CrimeGPT uses machine learning algorithms to analyze large data sets, identifying patterns that can predict where and when crimes are likely to occur. These data sets include historical crime statistics, demographic information, economic indicators, weather patterns, and more. By identifying trends that human analysts might miss, artificial intelligence can empower law enforcement agencies

01 Outlook Summary Currently, it is difficult to achieve an appropriate balance between detection efficiency and detection results. We have developed an enhanced YOLOv5 algorithm for target detection in high-resolution optical remote sensing images, using multi-layer feature pyramids, multi-detection head strategies and hybrid attention modules to improve the effect of the target detection network in optical remote sensing images. According to the SIMD data set, the mAP of the new algorithm is 2.2% better than YOLOv5 and 8.48% better than YOLOX, achieving a better balance between detection results and speed. 02 Background & Motivation With the rapid development of remote sensing technology, high-resolution optical remote sensing images have been used to describe many objects on the earth’s surface, including aircraft, cars, buildings, etc. Object detection in the interpretation of remote sensing images
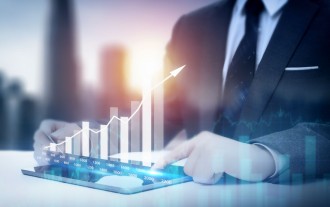
1. Background of the Construction of 58 Portraits Platform First of all, I would like to share with you the background of the construction of the 58 Portrait Platform. 1. The traditional thinking of the traditional profiling platform is no longer enough. Building a user profiling platform relies on data warehouse modeling capabilities to integrate data from multiple business lines to build accurate user portraits; it also requires data mining to understand user behavior, interests and needs, and provide algorithms. side capabilities; finally, it also needs to have data platform capabilities to efficiently store, query and share user profile data and provide profile services. The main difference between a self-built business profiling platform and a middle-office profiling platform is that the self-built profiling platform serves a single business line and can be customized on demand; the mid-office platform serves multiple business lines, has complex modeling, and provides more general capabilities. 2.58 User portraits of the background of Zhongtai portrait construction
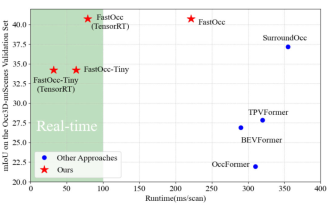
Written above & The author’s personal understanding is that in the autonomous driving system, the perception task is a crucial component of the entire autonomous driving system. The main goal of the perception task is to enable autonomous vehicles to understand and perceive surrounding environmental elements, such as vehicles driving on the road, pedestrians on the roadside, obstacles encountered during driving, traffic signs on the road, etc., thereby helping downstream modules Make correct and reasonable decisions and actions. A vehicle with self-driving capabilities is usually equipped with different types of information collection sensors, such as surround-view camera sensors, lidar sensors, millimeter-wave radar sensors, etc., to ensure that the self-driving vehicle can accurately perceive and understand surrounding environment elements. , enabling autonomous vehicles to make correct decisions during autonomous driving. Head
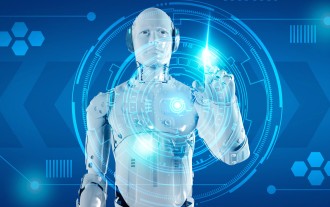
Author | Reviewed by Wang Hao | Chonglou News App is an important way for people to obtain information sources in their daily lives. Around 2010, popular foreign news apps included Zite and Flipboard, while popular domestic news apps were mainly the four major portals. With the popularity of new era news recommendation products represented by Toutiao, news apps have entered a new era. As for technology companies, no matter which one they are, as long as they master the sophisticated news recommendation algorithm technology, they will basically have the initiative and voice at the technical level. Today, let’s take a look at a RecSys2023 Best Long Paper Nomination Award paper—GoingBeyondLocal:GlobalGraph-EnhancedP
