2015.2.27
贪心算法) 一、基本概念: 所谓贪心算法是指,在对问题求解时,总是做出在当前看来是最好的选择。也就是说,不从整体最优上加以考虑,他所做出的仅是在某种意义上的局部最优解。 贪心算法没有固定的算法框架,算法设计的关键是贪心策略的选择。必须注意的是
贪心算法)
一、基本概念:
<code> 所谓贪心算法是指,在对问题求解时,总是做出在当前看来是最好的选择。也就是说,不从整体最优上加以考虑,他所做出的仅是在某种意义上的局部最优解。 贪心算法没有固定的算法框架,算法设计的关键是贪心策略的选择。必须注意的是,贪心算法不是对所有问题都能得到整体最优解,选择的贪心策略必须具备无后效性,即某个状态以后的过程不会影响以前的状态,只与当前状态有关。 所以对所采用的贪心策略一定要仔细分析其是否满足无后效性。 </code>
二、贪心算法的基本思路:
1.建立数学模型来描述问题。
2.把求解的问题分成若干个子问题。
3.对每一子问题求解,得到子问题的局部最优解。
4.把子问题的解局部最优解合成原来解问题的一个解。
三、贪心算法适用的问题
贪心策略适用的前提是:局部最优策略能导致产生全局最优解。
实际上,贪心算法适用的情况很少。一般,对一个问题分析是否适用于贪心算法,可以先选择该问题下的几个实际数据进行分析,就可做出判断。
四、贪心算法的实现框架
从问题的某一初始解出发;
<code> <span>while</span> (能朝给定总目标前进一步) <span>{ 利用可行的决策,求出可行解的一个解元素; }</span> 由所有解元素组合成问题的一个可行解; </code>
五、贪心策略的选择
因为用贪心算法只能通过解局部最优解的策略来达到全局最优解,因此,一定要注意判断问题是否适合采用贪心算法策略,找到的解是否一定是问题的最优解。
例题:
问题一、活动安排问题
问题表述:
设有n个活动的集合E = {1,2,…,n},其中每个活动都要求使用同一资源,如演讲会场等,而在同一时间内只有一个活动能使用这一资源。输入每个活动i都有一个要求使用该资源的起始时间si和一个结束时间fi,且si = fj或sj >= fi时,活动i与活动j相容。
由于输入的活动以其完成时间的非减序排列,所以算法greedySelector每次总是选择具有最早完成时间的相容活动加入集合A中。直观上,按这种方法选择相容活动为未安排活动留下尽可能多的时间。也就是说,该算法的贪心选择的意义是使剩余的可安排时间段极大化,以便安排尽可能多的相容活动。
算法greedySelector的效率极高。当输入的活动已按结束时间的非减序排列,算法只需O(n)的时间安排n个活动,使最多的活动能相容地使用公共资源。如果所给出的活动未按非减序排列,可以用O(nlogn)的时间重排。
例:设待安排的11个活动的开始时间和结束时间按结束时间的非减序排列如下:
算法greedySelector 的计算过程如下图所示。图中每行相应于算法的一次迭代。阴影长条表示的活动是已选入集合A的活动,而空白长条表示的活动是当前正在检查相容性的活动。
<code>若被检查的活动i的开始时间Si小于最近选择的活动j的结束时间fi,则不选择活动i,否则选择活动i加入集合A中。 贪心算法并不总能求得问题的整体最优解。但对于活动安排问题,贪心算法greedySelector却总能求得的整体最优解,即它最终所确定的相容活动集合A的规模最大。这个结论可以用数学归纳法证明。 </code>
<code>实现代码(我还看不懂啊~~~~~~): 代码 <span>/* 主题:活动安排问题 * 作者:chinazhangjie * 邮箱:chinajiezhang@gmail.com * 开发语言:C++ * 开发环境:Vicrosoft Visual Studio * 时间: 2010.11.21 */</span> <span>#include <iostream></iostream></span> <span>#include <vector></vector></span> <span>#include <algorithm></algorithm></span> <span>using</span> <span>namespace</span> <span>std</span> ; <span>struct</span> ActivityTime { <span>public</span>: ActivityTime (<span>int</span> nStart, <span>int</span> nEnd) : m_nStart (nStart), m_nEnd (nEnd) { } ActivityTime () : m_nStart (<span>0</span>), m_nEnd (<span>0</span>) { } <span>friend</span> <span>bool</span> <span>operator</span> const ActivityTime& lth, <span>const</span> ActivityTime& rth) { <span>return</span> lth.m_nEnd public: <span>int</span> m_nStart ; <span>int</span> m_nEnd ; } ; <span>class</span> ActivityArrange { <span>public</span>: ActivityArrange (<span>const</span> <span><span>vector</span><activitytime></activitytime></span>& vTimeList) { m_vTimeList = vTimeList ; m_nCount = vTimeList.size () ; m_bvSelectFlag.resize (m_nCount, <span>false</span>) ; } <span>// 活动安排</span> <span>void</span> greedySelector () { __sortTime () ; <span>// 第一个活动一定入内</span> m_bvSelectFlag[<span>0</span>] = <span>true</span> ; <span>int</span> j = <span>0</span> ; <span>for</span> (<span>int</span> i = <span>1</span>; i if (m_vTimeList[i].m_nStart > m_vTimeList[j].m_nEnd) { m_bvSelectFlag[i] = <span>true</span> ; j = i ; } } copy (m_bvSelectFlag.begin(), m_bvSelectFlag.end() ,ostream_iteratorbool> (<span>cout</span>, <span>" "</span>)); <span>cout</span> private: <span>// 按照活动结束时间非递减排序</span> <span>void</span> __sortTime () { sort (m_vTimeList.begin(), m_vTimeList.end()) ; <span>for</span> (<span><span>vector</span><activitytime></activitytime></span>::iterator ite = m_vTimeList.begin() ; ite != m_vTimeList.end() ; ++ ite) { <span>cout</span> m_nStart ", "m_nEnd private: <span><span>vector</span><activitytime></activitytime></span> m_vTimeList ; <span>// 活动时间安排列表</span> <span><span>vector</span>bool</span>> m_bvSelectFlag ;<span>// 是否安排活动标志</span> <span>int</span> m_nCount ; <span>// 总活动个数</span> } ; <span>int</span> main() { <span><span>vector</span><activitytime></activitytime></span> vActiTimeList ; vActiTimeList.push_back (ActivityTime(<span>1</span>, <span>4</span>)) ; vActiTimeList.push_back (ActivityTime(<span>3</span>, <span>5</span>)) ; vActiTimeList.push_back (ActivityTime(<span>0</span>, <span>6</span>)) ; vActiTimeList.push_back (ActivityTime(<span>5</span>, <span>7</span>)) ; vActiTimeList.push_back (ActivityTime(<span>3</span>, <span>8</span>)) ; vActiTimeList.push_back (ActivityTime(<span>5</span>, <span>9</span>)) ; vActiTimeList.push_back (ActivityTime(<span>6</span>, <span>10</span>)) ; vActiTimeList.push_back (ActivityTime(<span>8</span>, <span>11</span>)) ; vActiTimeList.push_back (ActivityTime(<span>8</span>, <span>12</span>)) ; vActiTimeList.push_back (ActivityTime(<span>2</span>, <span>13</span>)) ; vActiTimeList.push_back (ActivityTime(<span>12</span>, <span>14</span>)) ; ActivityArrange aa (vActiTimeList) ; aa.greedySelector () ; <span>return</span> <span>0</span> ; }</code>
2.快速幂算法
<code>Matrix qMPow(Matrix &<span>A</span>, int n) { Matrix rslt(<span>A</span>.N)<span>;</span> rslt.unit()<span>;</span> <span>if</span>(n == <span>0</span>) <span>return</span> rslt<span>;</span> <span>while</span>(n) { <span>if</span>(n & <span>1</span>) // 若幂为奇数 { rslt = rslt * <span>A</span><span>;</span> } <span>A</span> = <span>A</span> * <span>A</span><span>;</span> n >>= <span>1</span><span>; // 右位移等价于除以2</span> } <span>return</span> rslt<span>;</span> }</code>

Hot AI Tools

Undresser.AI Undress
AI-powered app for creating realistic nude photos

AI Clothes Remover
Online AI tool for removing clothes from photos.

Undress AI Tool
Undress images for free

Clothoff.io
AI clothes remover

Video Face Swap
Swap faces in any video effortlessly with our completely free AI face swap tool!

Hot Article

Hot Tools

Notepad++7.3.1
Easy-to-use and free code editor

SublimeText3 Chinese version
Chinese version, very easy to use

Zend Studio 13.0.1
Powerful PHP integrated development environment

Dreamweaver CS6
Visual web development tools

SublimeText3 Mac version
God-level code editing software (SublimeText3)

Hot Topics
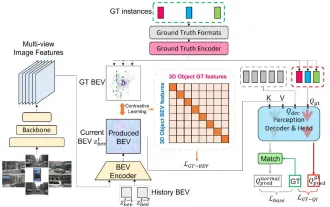
Written above & the author’s personal understanding: At present, in the entire autonomous driving system, the perception module plays a vital role. The autonomous vehicle driving on the road can only obtain accurate perception results through the perception module. The downstream regulation and control module in the autonomous driving system makes timely and correct judgments and behavioral decisions. Currently, cars with autonomous driving functions are usually equipped with a variety of data information sensors including surround-view camera sensors, lidar sensors, and millimeter-wave radar sensors to collect information in different modalities to achieve accurate perception tasks. The BEV perception algorithm based on pure vision is favored by the industry because of its low hardware cost and easy deployment, and its output results can be easily applied to various downstream tasks.
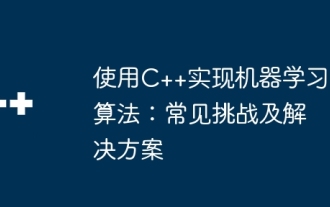
Common challenges faced by machine learning algorithms in C++ include memory management, multi-threading, performance optimization, and maintainability. Solutions include using smart pointers, modern threading libraries, SIMD instructions and third-party libraries, as well as following coding style guidelines and using automation tools. Practical cases show how to use the Eigen library to implement linear regression algorithms, effectively manage memory and use high-performance matrix operations.
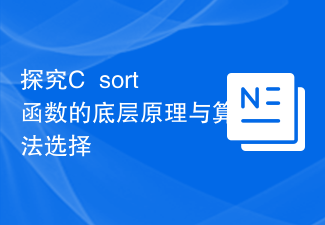
The bottom layer of the C++sort function uses merge sort, its complexity is O(nlogn), and provides different sorting algorithm choices, including quick sort, heap sort and stable sort.
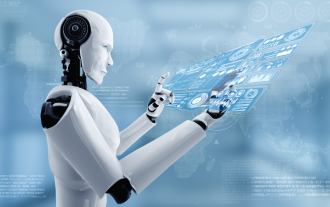
The convergence of artificial intelligence (AI) and law enforcement opens up new possibilities for crime prevention and detection. The predictive capabilities of artificial intelligence are widely used in systems such as CrimeGPT (Crime Prediction Technology) to predict criminal activities. This article explores the potential of artificial intelligence in crime prediction, its current applications, the challenges it faces, and the possible ethical implications of the technology. Artificial Intelligence and Crime Prediction: The Basics CrimeGPT uses machine learning algorithms to analyze large data sets, identifying patterns that can predict where and when crimes are likely to occur. These data sets include historical crime statistics, demographic information, economic indicators, weather patterns, and more. By identifying trends that human analysts might miss, artificial intelligence can empower law enforcement agencies

01 Outlook Summary Currently, it is difficult to achieve an appropriate balance between detection efficiency and detection results. We have developed an enhanced YOLOv5 algorithm for target detection in high-resolution optical remote sensing images, using multi-layer feature pyramids, multi-detection head strategies and hybrid attention modules to improve the effect of the target detection network in optical remote sensing images. According to the SIMD data set, the mAP of the new algorithm is 2.2% better than YOLOv5 and 8.48% better than YOLOX, achieving a better balance between detection results and speed. 02 Background & Motivation With the rapid development of remote sensing technology, high-resolution optical remote sensing images have been used to describe many objects on the earth’s surface, including aircraft, cars, buildings, etc. Object detection in the interpretation of remote sensing images
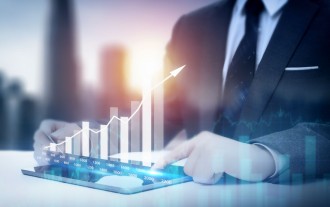
1. Background of the Construction of 58 Portraits Platform First of all, I would like to share with you the background of the construction of the 58 Portrait Platform. 1. The traditional thinking of the traditional profiling platform is no longer enough. Building a user profiling platform relies on data warehouse modeling capabilities to integrate data from multiple business lines to build accurate user portraits; it also requires data mining to understand user behavior, interests and needs, and provide algorithms. side capabilities; finally, it also needs to have data platform capabilities to efficiently store, query and share user profile data and provide profile services. The main difference between a self-built business profiling platform and a middle-office profiling platform is that the self-built profiling platform serves a single business line and can be customized on demand; the mid-office platform serves multiple business lines, has complex modeling, and provides more general capabilities. 2.58 User portraits of the background of Zhongtai portrait construction
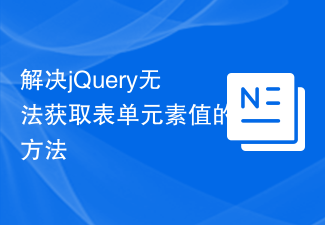
To solve the problem that jQuery.val() cannot be used, specific code examples are required. For front-end developers, using jQuery is one of the common operations. Among them, using the .val() method to get or set the value of a form element is a very common operation. However, in some specific cases, the problem of not being able to use the .val() method may arise. This article will introduce some common situations and solutions, and provide specific code examples. Problem Description When using jQuery to develop front-end pages, sometimes you will encounter
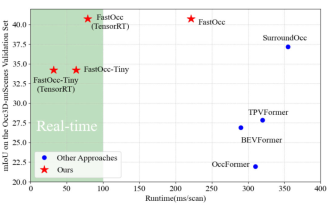
Written above & The author’s personal understanding is that in the autonomous driving system, the perception task is a crucial component of the entire autonomous driving system. The main goal of the perception task is to enable autonomous vehicles to understand and perceive surrounding environmental elements, such as vehicles driving on the road, pedestrians on the roadside, obstacles encountered during driving, traffic signs on the road, etc., thereby helping downstream modules Make correct and reasonable decisions and actions. A vehicle with self-driving capabilities is usually equipped with different types of information collection sensors, such as surround-view camera sensors, lidar sensors, millimeter-wave radar sensors, etc., to ensure that the self-driving vehicle can accurately perceive and understand surrounding environment elements. , enabling autonomous vehicles to make correct decisions during autonomous driving. Head
