Golang technology for interpretability tools in machine learning
The Go language is extremely advantageous for building machine learning interpretability tools due to its high speed, concurrency, and memory safety features. In a practical case, a LIME interpreter was built using Go, which can explain local model predictions. Its advantages include high performance, memory safety, and ease of use.
Application of Go language technology in machine learning interpretability tools
Introduction
The explainability of machine learning models is critical to understanding their decisions and building trust. The Go language has demonstrated strong advantages in building interpretability tools due to its speed, concurrency, and memory safety features.
Practical Case: Using Go to Build a LIME Interpreter
Local Interpretable Model Interpretability (LIME) is a popular interpretability technology that creates A locally linear approximate model to explain machine learning predictions. The following Go code shows how to use LIME to create a LIME interpreter:
import ( "github.com/martijnvg/lime" "github.com/gonum/blas" "github.com/gonum/mat" ) // Create a LIME explainer func NewLIMEExplainer(data, labels [][]float64, kernelWidth float64) *lime.Explainer { samples := mat.NewDense(len(data), len(data[0]), nil) for i, v := range data { samples.SetRow(i, v) } weights := mat.NewDense(len(labels), len(labels), nil) for i, v := range labels { weights.Set(i, i, v) } explainer := lime.NewExplainer(samples, weights, kernelWidth) explainer.SetNormalize(true) explainer.SetVerbose(true) return explainer } // Explain a prediction with LIME func ExplainPrediction(explainer *lime.Explainer, point []float64) *lime.Explanation { pointMat := mat.NewDense(1, len(point), point) return explainer.Explain(pointMat, 10) }
Use Case
The above LIME interpreter can be used for a variety of machine learning interpretability Task:
- Understand the decisions of classification models
- Identify key features that affect predictions
- Detect model biases and errors
Advantages
Using the Go language to build interpretability tools has the following advantages:
- High performance: The speed and concurrency of the Go language in processing Very effective with large amounts of data.
- Memory Safety: The memory management features of the Go language help ensure the stability of interpretability tools.
- Easy to use: The syntax of the Go language is clear and concise, making it easy to develop and maintain interpretability tools.
Conclusion
The Go language has great potential for the development of machine learning interpretability tools. It provides a powerful set of features for building efficient, stable, and easy-to-use interpretability tools to help understand and trust machine learning models.
The above is the detailed content of Golang technology for interpretability tools in machine learning. For more information, please follow other related articles on the PHP Chinese website!

Hot AI Tools

Undresser.AI Undress
AI-powered app for creating realistic nude photos

AI Clothes Remover
Online AI tool for removing clothes from photos.

Undress AI Tool
Undress images for free

Clothoff.io
AI clothes remover

Video Face Swap
Swap faces in any video effortlessly with our completely free AI face swap tool!

Hot Article

Hot Tools

Notepad++7.3.1
Easy-to-use and free code editor

SublimeText3 Chinese version
Chinese version, very easy to use

Zend Studio 13.0.1
Powerful PHP integrated development environment

Dreamweaver CS6
Visual web development tools

SublimeText3 Mac version
God-level code editing software (SublimeText3)

Hot Topics
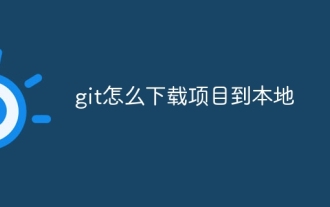
To download projects locally via Git, follow these steps: Install Git. Navigate to the project directory. cloning the remote repository using the following command: git clone https://github.com/username/repository-name.git
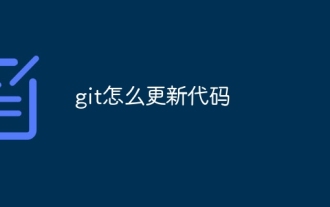
Steps to update git code: Check out code: git clone https://github.com/username/repo.git Get the latest changes: git fetch merge changes: git merge origin/master push changes (optional): git push origin master
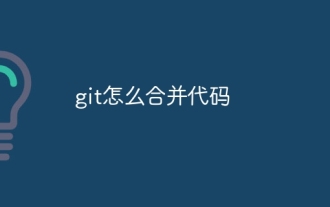
Git code merge process: Pull the latest changes to avoid conflicts. Switch to the branch you want to merge. Initiate a merge, specifying the branch to merge. Resolve merge conflicts (if any). Staging and commit merge, providing commit message.
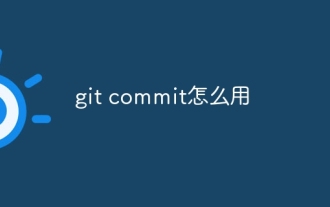
Git Commit is a command that records file changes to a Git repository to save a snapshot of the current state of the project. How to use it is as follows: Add changes to the temporary storage area Write a concise and informative submission message to save and exit the submission message to complete the submission optionally: Add a signature for the submission Use git log to view the submission content
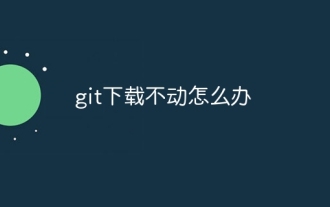
Resolve: When Git download speed is slow, you can take the following steps: Check the network connection and try to switch the connection method. Optimize Git configuration: Increase the POST buffer size (git config --global http.postBuffer 524288000), and reduce the low-speed limit (git config --global http.lowSpeedLimit 1000). Use a Git proxy (such as git-proxy or git-lfs-proxy). Try using a different Git client (such as Sourcetree or Github Desktop). Check for fire protection
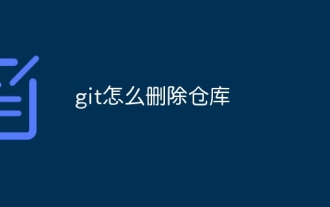
To delete a Git repository, follow these steps: Confirm the repository you want to delete. Local deletion of repository: Use the rm -rf command to delete its folder. Remotely delete a warehouse: Navigate to the warehouse settings, find the "Delete Warehouse" option, and confirm the operation.
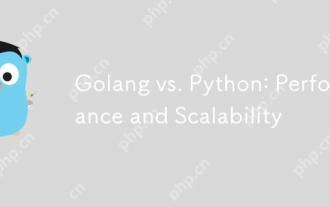
Golang is better than Python in terms of performance and scalability. 1) Golang's compilation-type characteristics and efficient concurrency model make it perform well in high concurrency scenarios. 2) Python, as an interpreted language, executes slowly, but can optimize performance through tools such as Cython.
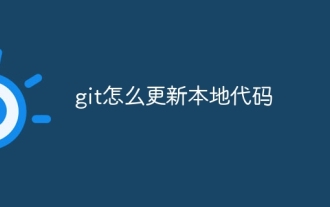
How to update local Git code? Use git fetch to pull the latest changes from the remote repository. Merge remote changes to the local branch using git merge origin/<remote branch name>. Resolve conflicts arising from mergers. Use git commit -m "Merge branch <Remote branch name>" to submit merge changes and apply updates.
