


How does the partition tool diskgenius detect bad sectors on a disk - How does the partition tool diskgenius detect bad sectors on a disk
php editor Baicao introduces to you how the partition tool DiskGenius detects bad sectors on the disk. DiskGenius has a powerful disk detection function that can quickly discover and repair disk bad sector problems. Through simple operation steps, users can easily detect the disk, understand the health status of the disk, deal with disk bad sector problems in a timely manner, and ensure data security. Next, let's take a detailed look at DiskGenius's method of detecting disk bad sectors.
Step 1: Open the DiskGenius software and select the disk you want to detect.
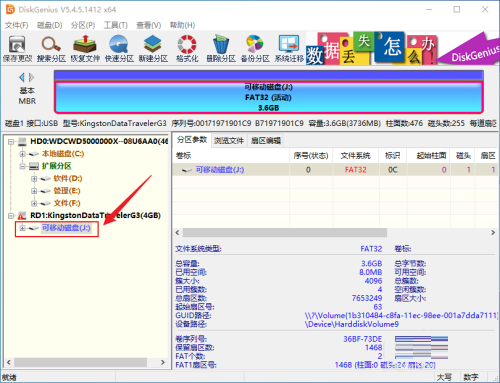
Step 2: Click "Bad Sector Detection and Repair" under the "Disk" menu.
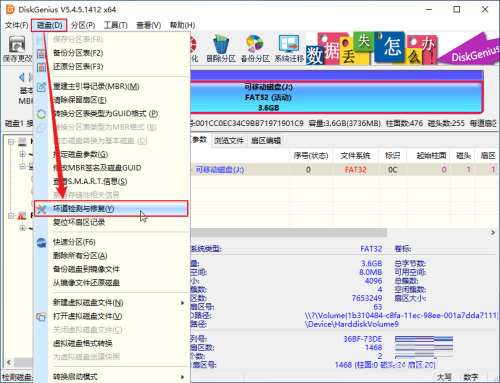
Step 3: Confirm whether the selected disk is correct, and then click "Start Detection" to detect the bad sectors of the U disk.
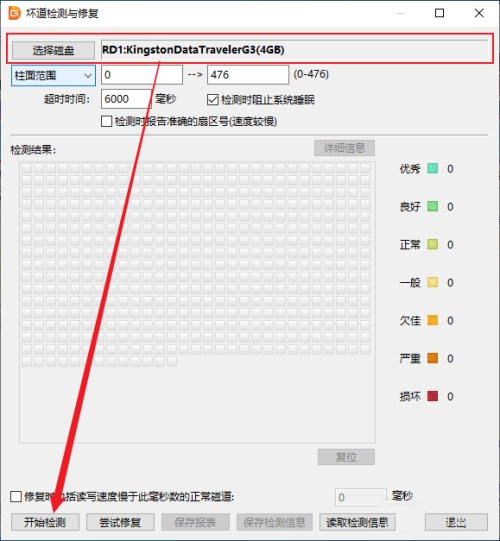
Step 4: Enter the bad sector detection interface until the detection is completed.
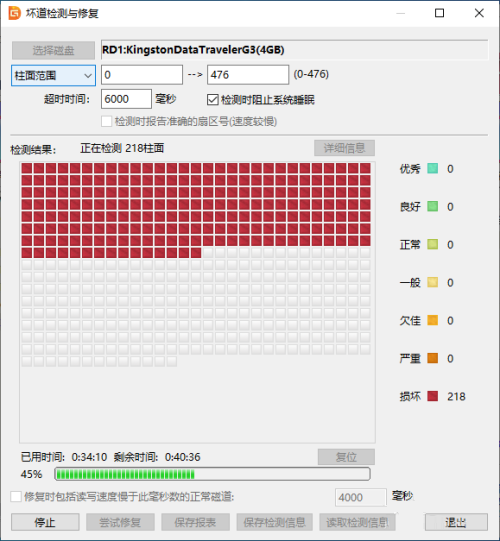
The above is the detailed content of How does the partition tool diskgenius detect bad sectors on a disk - How does the partition tool diskgenius detect bad sectors on a disk. For more information, please follow other related articles on the PHP Chinese website!

Hot AI Tools

Undresser.AI Undress
AI-powered app for creating realistic nude photos

AI Clothes Remover
Online AI tool for removing clothes from photos.

Undress AI Tool
Undress images for free

Clothoff.io
AI clothes remover

Video Face Swap
Swap faces in any video effortlessly with our completely free AI face swap tool!

Hot Article

Hot Tools

Notepad++7.3.1
Easy-to-use and free code editor

SublimeText3 Chinese version
Chinese version, very easy to use

Zend Studio 13.0.1
Powerful PHP integrated development environment

Dreamweaver CS6
Visual web development tools

SublimeText3 Mac version
God-level code editing software (SublimeText3)

Hot Topics










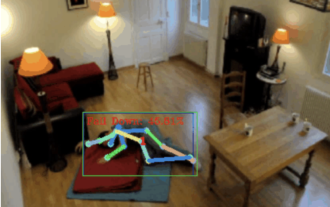
Hello everyone. Today I would like to share with you a fall detection project, to be precise, it is human movement recognition based on skeletal points. It is roughly divided into three steps: human body recognition, human skeleton point action classification project source code has been packaged, see the end of the article for how to obtain it. 0. chatgpt First, we need to obtain the monitored video stream. This code is relatively fixed. We can directly let chatgpt complete the code written by chatgpt. There is no problem and can be used directly. But when it comes to business tasks later, such as using mediapipe to identify human skeleton points, the code given by chatgpt is incorrect. I think chatgpt can be used as a toolbox that is independent of business logic. You can try to hand it over to c
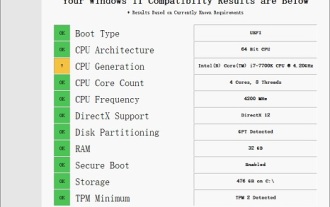
The performance of i77700 is completely sufficient to run win11, but users find that their i77700 cannot be upgraded to win11. This is mainly due to restrictions imposed by Microsoft, so they can install it as long as they skip this restriction. i77700 cannot be upgraded to win11: 1. Because Microsoft limits the CPU version. 2. Only the eighth generation and above versions of Intel can directly upgrade to win11. 3. As the 7th generation, i77700 cannot meet the upgrade needs of win11. 4. However, i77700 is completely capable of using win11 smoothly in terms of performance. 5. So you can use the win11 direct installation system of this site. 6. After the download is complete, right-click the file and "load" it. 7. Double-click to run the "One-click
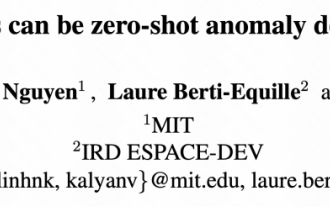
Today I would like to introduce to you an article published by MIT last week, using GPT-3.5-turbo to solve the problem of time series anomaly detection, and initially verifying the effectiveness of LLM in time series anomaly detection. There is no finetune in the whole process, and GPT-3.5-turbo is used directly for anomaly detection. The core of this article is how to convert time series into input that can be recognized by GPT-3.5-turbo, and how to design prompts or pipelines to let LLM solve the anomaly detection task. Let me introduce this work to you in detail. Image paper title: Largelanguagemodelscanbezero-shotanomalydete

01 Outlook Summary Currently, it is difficult to achieve an appropriate balance between detection efficiency and detection results. We have developed an enhanced YOLOv5 algorithm for target detection in high-resolution optical remote sensing images, using multi-layer feature pyramids, multi-detection head strategies and hybrid attention modules to improve the effect of the target detection network in optical remote sensing images. According to the SIMD data set, the mAP of the new algorithm is 2.2% better than YOLOv5 and 8.48% better than YOLOX, achieving a better balance between detection results and speed. 02 Background & Motivation With the rapid development of remote sensing technology, high-resolution optical remote sensing images have been used to describe many objects on the earth’s surface, including aircraft, cars, buildings, etc. Object detection in the interpretation of remote sensing images
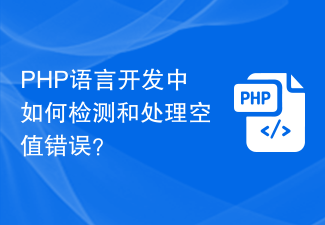
With the continuous development of modern web applications, PHP, as one of the most popular programming languages, is widely used in website development. However, during the development process, null value errors are often encountered, and these errors can cause the application to throw exceptions, thus affecting the user experience. Therefore, in the PHP development process, how to detect and deal with null errors is an important skill that programmers need to master. 1. What is a null value error? In the PHP development process, null value errors usually refer to two situations: uninitialized variables and variable variables.

Recently, I read a latest research on pure visual surround perception on Arxiv. This research is based on the PETR series of methods and focuses on solving the pure visual perception problem of long-distance target detection, extending the perception range to 150 meters. The methods and results of this paper have great reference value for us, so I tried to interpret it. Original title: Far3D: Expanding the Horizon for Surround-view3DObject Detection Paper link: https://arxiv.org/abs/2308.09616 Author affiliation :Beijing Institute of Technology & Megvii Technology Task Background 3D Object Detection in Understanding Autonomous Driving
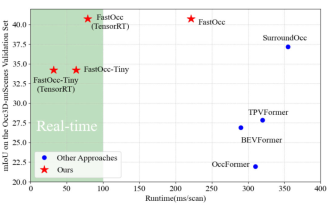
Written above & The author’s personal understanding is that in the autonomous driving system, the perception task is a crucial component of the entire autonomous driving system. The main goal of the perception task is to enable autonomous vehicles to understand and perceive surrounding environmental elements, such as vehicles driving on the road, pedestrians on the roadside, obstacles encountered during driving, traffic signs on the road, etc., thereby helping downstream modules Make correct and reasonable decisions and actions. A vehicle with self-driving capabilities is usually equipped with different types of information collection sensors, such as surround-view camera sensors, lidar sensors, millimeter-wave radar sensors, etc., to ensure that the self-driving vehicle can accurately perceive and understand surrounding environment elements. , enabling autonomous vehicles to make correct decisions during autonomous driving. Head
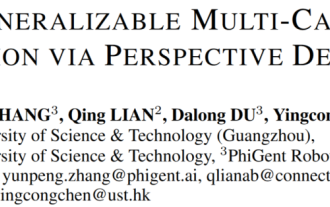
Written above & the author's personal summary Birdeye'sview (BEV) detection is a method of detection by fusing multiple surround-view cameras. Most of the current algorithms are trained and evaluated on the same data set, which causes these algorithms to overfit to the unchanged camera internal parameters (camera type) and external parameters (camera placement). This paper proposes a BEV detection framework based on implicit rendering, which can solve the problem of object detection in unknown domains. The framework uses implicit rendering to establish the relationship between the 3D position of the object and the perspective position of a single view, which can be used to correct perspective bias. This method achieves significant performance improvements in domain generalization (DG) and unsupervised domain adaptation (UDA). This method was first tried using only virtual numbers
