


New work by the author of ControlNet: AI painting can be divided into layers! The project earned 660 stars without being open source
"It is by no means a simple cutout."
ControlNet authorThe latest study has received a wave of high attention——
Give me a prompt, you can use Stable Diffusion to directly generate single or multiple transparent layers (PNG) !
For example:
A woman with messy hair is in the bedroom.
Woman with messy hair, in the bedroom.
As you can see, AI not only generated a complete image that conforms to the prompt, but even the background Can also be separated from characters.
And if you zoom in on the character PNG image and take a closer look, you will see that the hair strands are clearly defined.
Look at another example:
Burning firewood, on a table, in the countryside.
Burning firewood, on a table, in the countryside.
Similarly, zoom in on the PNG of the "burning match", even the black smoke around the flame can all be separated:
This is the new method proposed by the author of ControlNet - LayerDiffusion, which allows large-scale pre-training of latent diffusion models (Latent Diffusion Model) generates transparent images.
It is worth emphasizing again that LayerDiffusion is by no means as simple as cutout, the focus is on generating.
As netizens said:
This is one of the core processes in animation and video production now. If this step can be passed, it can be said that SD consistency is no longer a problem.
# Some netizens thought that a job like this was not difficult, just a matter of "adding an alpha channel by the way", but what surprised him was:
It took so long for the results to come out.
#So how is LayerDiffusion implemented?
PNG, now starting to take the generation route
The core of LayerDiffusion is a method called latent transparency(latent transparency).
Simply put, it allows adding transparency to the model without destroying the latent distribution of the pre-trained latent diffusion model (such as Stable Diffusion) .
In terms of specific implementation, it can be understood as adding a carefully designed small perturbation (offset) to the latent image. This perturbation is encoded as an additional channel, which together with the RGB channel constitutes a complete potential image.
In order to achieve encoding and decoding of transparency, the author trained two independent neural network models: one is the latent transparency encoder(latent transparency encoder), and the other One is latent transparency decoder(latent transparency decoder).
The encoder receives the RGB channel and alpha channel of the original image as input and converts the transparency information into an offset in the latent space.
The decoder receives the adjusted latent image and the reconstructed RGB image, and extracts the transparency information from the latent space to reconstruct the original transparent image.
To ensure that the added potential transparency does not destroy the underlying distribution of the pre-trained model, the authors propose a measure of "harmlessness" .
This metric evaluates the impact of latent transparency by comparing the decoding results of the adjusted latent image by the original pre-trained model's decoder to the original image.(joint loss function), which combines the reconstruction loss( reconstruction loss), identity loss (identity loss) and discriminator loss. Their functions are:
Reconstruction loss: used to ensure that the decoded image is as similar as possible to the original image;- Identity loss: used to ensure The adjusted latent image can be correctly decoded by the pre-trained decoder;
- discriminator loss: is used to improve the realism of the generated image.
- With this approach, any latent diffusion model can be converted into a transparent image generator by simply fine-tuning it to fit the adjusted latent space.
The concept of latent transparency can also be extended to generate multiple transparent layers, as well as combined with other conditional control systems to achieve more complex image generation tasks such as foreground/background Conditional generation, joint layer generation, structural control of layer content, etc.
##It is worth mentioning that the author also shows how to introduce ControlNet to enrich the functions of LayerDiffusion:
The difference with traditional cutout
Native generation vs. post-processing
LayerDiffusion is a native transparent image generation method that considers and encodes transparency information directly during the generation process. This means that the model creates an alpha channel when generating the image, thus producing an image with transparency.
Traditional matting methods usually involve first generating or obtaining an image, and then using image editing techniques(such as chroma key, edge detection, user-specified masks, etc.) to separate the foreground and background. This approach often requires additional steps to handle transparency and can produce unnatural transitions on complex backgrounds or edges.
Latent space operations vs. pixel space operations
LayerDiffusion operates in latent space (latent space) , which is an intermediate representation that allows the model to learn and generate more complex image features. By encoding transparency in the latent space, the model can naturally handle transparency during generation without the need for complex calculations at the pixel level.
Traditional cutout technology is usually performed in pixel space, which may involve direct editing of the original image, such as color replacement, edge smoothing, etc. These methods may have difficulty handling translucent effects (such as fire, smoke) or complex edges.
Dataset and training
LayerDiffusion uses a large-scale data set for training. This data set contains transparent image pairs, allowing the model to learn to generate high-definition images. Complex distribution required for quality transparent images.
Traditional matting methods may rely on smaller data sets or specific training sets, which may limit their ability to handle diverse scenarios.
Flexibility and control
LayerDiffusion provides greater flexibility and control because it allows users to prompt via text (text prompts) to guide the generation of images and can generate multiple layers that can be blended and combined to create complex scenes.
Traditional cutout methods may be more limited in control, especially when dealing with complex image content and transparency.
Quality Comparison
User research shows that transparent images generated by LayerDiffusion are preferred by users in most cases (97%) , indicating The transparent content it generates is visually equivalent to, or perhaps even superior to, commercial transparent assets.
Traditional cutout methods may not achieve the same quality in some cases, especially when dealing with challenging transparency and edges.
In short, LayerDiffusion provides a more advanced and flexible method to generate and process transparent images.
It encodes transparency directly during the generation process and is able to produce high-quality results that are difficult to achieve in traditional matting methods.
About the author
As we just mentioned, one of the authors of this study is the inventor of the famous ControlNet-Zhang Lumin.
He graduated from Suzhou University with an undergraduate degree. He published a paper related to AI painting when he was a freshman. During his undergraduate period, he published 10 top-level works.
Zhang Lumin is currently studying for a PhD at Stanford University, but he can be said to be very low-key and has not even registered for Google Scholar.
As of now, LayerDiffusion is not open source in GitHub, but even so it cannot stop everyone's attention and has already gained 660 stars.
After all, Zhang Lumin has also been ridiculed by netizens as a "time management master". Friends who are interested in LayerDiffusion can mark it in advance.
The above is the detailed content of New work by the author of ControlNet: AI painting can be divided into layers! The project earned 660 stars without being open source. For more information, please follow other related articles on the PHP Chinese website!

Hot AI Tools

Undresser.AI Undress
AI-powered app for creating realistic nude photos

AI Clothes Remover
Online AI tool for removing clothes from photos.

Undress AI Tool
Undress images for free

Clothoff.io
AI clothes remover

Video Face Swap
Swap faces in any video effortlessly with our completely free AI face swap tool!

Hot Article

Hot Tools

Notepad++7.3.1
Easy-to-use and free code editor

SublimeText3 Chinese version
Chinese version, very easy to use

Zend Studio 13.0.1
Powerful PHP integrated development environment

Dreamweaver CS6
Visual web development tools

SublimeText3 Mac version
God-level code editing software (SublimeText3)

Hot Topics
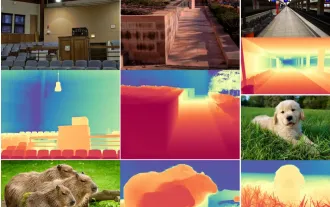
0.What does this article do? We propose DepthFM: a versatile and fast state-of-the-art generative monocular depth estimation model. In addition to traditional depth estimation tasks, DepthFM also demonstrates state-of-the-art capabilities in downstream tasks such as depth inpainting. DepthFM is efficient and can synthesize depth maps within a few inference steps. Let’s read about this work together ~ 1. Paper information title: DepthFM: FastMonocularDepthEstimationwithFlowMatching Author: MingGui, JohannesS.Fischer, UlrichPrestel, PingchuanMa, Dmytr
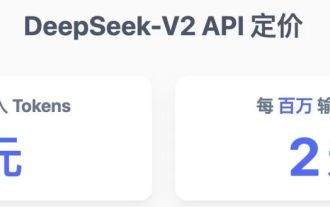
Imagine an artificial intelligence model that not only has the ability to surpass traditional computing, but also achieves more efficient performance at a lower cost. This is not science fiction, DeepSeek-V2[1], the world’s most powerful open source MoE model is here. DeepSeek-V2 is a powerful mixture of experts (MoE) language model with the characteristics of economical training and efficient inference. It consists of 236B parameters, 21B of which are used to activate each marker. Compared with DeepSeek67B, DeepSeek-V2 has stronger performance, while saving 42.5% of training costs, reducing KV cache by 93.3%, and increasing the maximum generation throughput to 5.76 times. DeepSeek is a company exploring general artificial intelligence
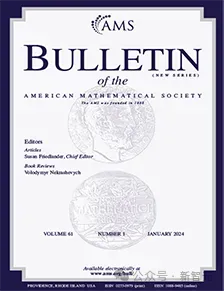
AI is indeed changing mathematics. Recently, Tao Zhexuan, who has been paying close attention to this issue, forwarded the latest issue of "Bulletin of the American Mathematical Society" (Bulletin of the American Mathematical Society). Focusing on the topic "Will machines change mathematics?", many mathematicians expressed their opinions. The whole process was full of sparks, hardcore and exciting. The author has a strong lineup, including Fields Medal winner Akshay Venkatesh, Chinese mathematician Zheng Lejun, NYU computer scientist Ernest Davis and many other well-known scholars in the industry. The world of AI has changed dramatically. You know, many of these articles were submitted a year ago.
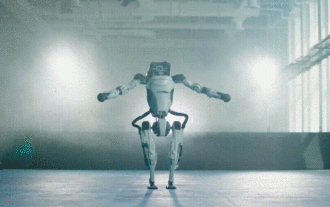
Boston Dynamics Atlas officially enters the era of electric robots! Yesterday, the hydraulic Atlas just "tearfully" withdrew from the stage of history. Today, Boston Dynamics announced that the electric Atlas is on the job. It seems that in the field of commercial humanoid robots, Boston Dynamics is determined to compete with Tesla. After the new video was released, it had already been viewed by more than one million people in just ten hours. The old people leave and new roles appear. This is a historical necessity. There is no doubt that this year is the explosive year of humanoid robots. Netizens commented: The advancement of robots has made this year's opening ceremony look like a human, and the degree of freedom is far greater than that of humans. But is this really not a horror movie? At the beginning of the video, Atlas is lying calmly on the ground, seemingly on his back. What follows is jaw-dropping
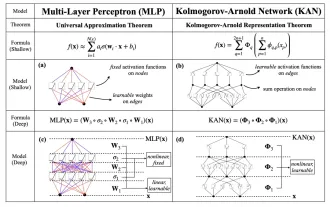
Earlier this month, researchers from MIT and other institutions proposed a very promising alternative to MLP - KAN. KAN outperforms MLP in terms of accuracy and interpretability. And it can outperform MLP running with a larger number of parameters with a very small number of parameters. For example, the authors stated that they used KAN to reproduce DeepMind's results with a smaller network and a higher degree of automation. Specifically, DeepMind's MLP has about 300,000 parameters, while KAN only has about 200 parameters. KAN has a strong mathematical foundation like MLP. MLP is based on the universal approximation theorem, while KAN is based on the Kolmogorov-Arnold representation theorem. As shown in the figure below, KAN has
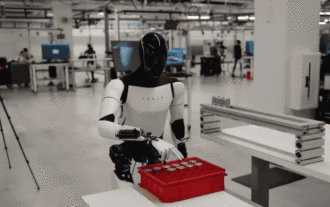
The latest video of Tesla's robot Optimus is released, and it can already work in the factory. At normal speed, it sorts batteries (Tesla's 4680 batteries) like this: The official also released what it looks like at 20x speed - on a small "workstation", picking and picking and picking: This time it is released One of the highlights of the video is that Optimus completes this work in the factory, completely autonomously, without human intervention throughout the process. And from the perspective of Optimus, it can also pick up and place the crooked battery, focusing on automatic error correction: Regarding Optimus's hand, NVIDIA scientist Jim Fan gave a high evaluation: Optimus's hand is the world's five-fingered robot. One of the most dexterous. Its hands are not only tactile
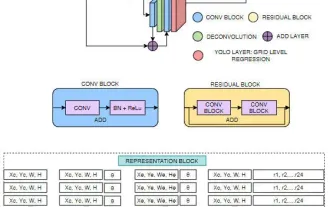
Target detection is a relatively mature problem in autonomous driving systems, among which pedestrian detection is one of the earliest algorithms to be deployed. Very comprehensive research has been carried out in most papers. However, distance perception using fisheye cameras for surround view is relatively less studied. Due to large radial distortion, standard bounding box representation is difficult to implement in fisheye cameras. To alleviate the above description, we explore extended bounding box, ellipse, and general polygon designs into polar/angular representations and define an instance segmentation mIOU metric to analyze these representations. The proposed model fisheyeDetNet with polygonal shape outperforms other models and simultaneously achieves 49.5% mAP on the Valeo fisheye camera dataset for autonomous driving
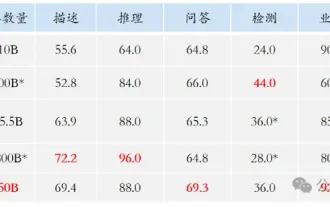
I cry to death. The world is madly building big models. The data on the Internet is not enough. It is not enough at all. The training model looks like "The Hunger Games", and AI researchers around the world are worrying about how to feed these data voracious eaters. This problem is particularly prominent in multi-modal tasks. At a time when nothing could be done, a start-up team from the Department of Renmin University of China used its own new model to become the first in China to make "model-generated data feed itself" a reality. Moreover, it is a two-pronged approach on the understanding side and the generation side. Both sides can generate high-quality, multi-modal new data and provide data feedback to the model itself. What is a model? Awaker 1.0, a large multi-modal model that just appeared on the Zhongguancun Forum. Who is the team? Sophon engine. Founded by Gao Yizhao, a doctoral student at Renmin University’s Hillhouse School of Artificial Intelligence.
