


Anonymous papers come up with surprising ideas! This can actually be done to enhance the long text capabilities of large models
When it comes to improving the long text capabilities of large models, do you think of length extrapolation or context window expansion?
No, these consume too much hardware resources.
Let’s look at a wonderful new solution:
The essence of using KV cache is different from methods such as length extrapolation. It uses parameters of the modelTo store a large amount of contextual information.
The specific method is to build a temporary Lora module , so that it can only "stream update" during the long text generation process, that is Previously generated content is continuously used as input to serve as training data to ensure that knowledge is stored in the model parameters.
ThenOnce the inference is complete, throw it away to ensure that it does not have a long-term impact on the model parameters.
This method allows us to store context information at will without expanding the context window, save as much as we want.
Experiments have proven that this method:
- can significantly improve the quality of long text tasks of the model, achieving a 29.6% reduction in confusion, and the quality of long text translation (BLUE score) increased by 53.2%;
- is also compatible with and enhances most existing long text generation methods.
- The most important thing is that it can greatly reduce computing costs.
While ensuring a slight improvement in generation quality (perplexity reduced by 3.8%), the FLOPs required for reasoning are reduced by 70.5% and the delay is reduced by 51.5%!
For the specific situation, let’s open the paper and take a look.
Create a temporary Lora module and throw it away after use
The method is called Temp-Lora, and the architecture diagram is as follows:
The core is to gradually train the temporary Lora module on the previously generated text in an autoregressive manner.
This module is very adaptable and can be continuously adjusted, so it can have a deep understanding of contexts of different distances.
The specific algorithm is as follows:
During the generation process, tokens are generated block by block. Each time a block is generated, the latest Lxtoken is used as input X to generate subsequent tokens.
Once the number of generated tokens reaches the predefined block size Δ, start the training of the Temp-Lora module using the latest block, and then start the next block generation.
In the experiment, the author sets Δ Lx to W to make full use of the model’s context window size.
For the training of the Temp-Lora module, learning to generate new blocks without any conditions may not constitute an effective training goal and lead to serious overfitting.
To solve this problem, the author incorporates the LT tags in front of each block into the training process, using them as input and the block as output.
Finally, the author also proposed a strategy called Cache Reuse (Cache Reuse) to achieve more efficient reasoning.
Generally speaking, after updating the Temp-Loramo module in the standard framework, we need to recalculate the KV status using the updated parameters.
Alternatively, reuse the existing cached KV state while using the updated model for subsequent text generation.
Specifically, we recalculate the KV state using the latest Temp-Lora module only when the model generates a maximum length (context window size W) .
Such a cache reuse method can speed up the generation without significantly affecting the generation quality.
That’s all the introduction to the Temp-Lora method. Let’s focus on the tests below.
The longer the text, the better the effect
The author tested the Temp- The Lora framework is evaluated and covers both types of long text tasks: generation and translation.
The test data set is a subset of the long text language modeling benchmark PG19, from which 40 books were randomly selected.
The other is a randomly sampled subset of the Guofeng data set from WMT 2023, which contains 20 Chinese online novels translated into English by professionals.
First let’s look at the results on PG19.
The table below shows the PPL (Perplexity, reflecting the uncertainty of the model for a given input) for various models on PG19 with and without the Temp-Lora module sex, the lower the better)Compare. Divide each document into segments ranging from 0-100K to 500K tokens.
It can be seen that the PPL of all models dropped significantly after Temp-Lora, and as the fragments become longer and longer, the impact of Temp-Lora is more obvious (1-100K only decreased by 3.6% , 500K reduced by 13.2%).
Therefore, we can simply conclude: The more text there is, the stronger the necessity to use Temp-Lora.
In addition we can also find that adjusting the block size from 1024 to 2048 and 4096 results in a slight increase in PPL.
This is not surprising, after all, the Temp-Lora module is trained on the data of the previous block.
This data mainly tells us that the choice of block size is a key trade-off between generation quality and computational efficiency (further analysis can be found in the paper) .
Finally, we can also find that cache reuse will not cause any performance loss.
The author said: This is very encouraging news.
The following are the results on the Guofeng data set.
It can be seen that Temp-Lora also has a significant impact on long text literary translation tasks.
Significant improvement in all metrics compared to the base model: PPL reduced by -29.6%, BLEU score (similarity of machine translated text to high-quality reference translation) improved 53.2%, and the COMET score (also a quality indicator) increased by 8.4%.
Finally, there is the exploration of computational efficiency and quality.
The author found through experiments that using the most "economical" Temp-Lora configuration (Δ=2K, W=4K) can While reducing PPL by 3.8%, it saves 70.5% of FLOPs and 51.5% of latency.
On the contrary, if we completely ignore the computational cost and use the most "luxurious" configuration (Δ=1K and W=24K) , It is also possible to achieve a 5.0% PPL reduction and an additional 17% FLOP and 19.6% latency.
Usage Suggestions
To summarize the above results, the author also gives three suggestions for the practical application of Temp-Lora:
1. For applications that require the highest level of long text generation , without changing any parameters, integrating Temp-Lora into the existing model can significantly improve performance at a relatively moderate cost.
2. For applications that value minimal latency or memory usage, the computational cost can be significantly reduced by reducing the input length and contextual information stored in Temp-Lora.
In this setting, we can use a fixed short window size (such as 2K or 4K) to handle almost infinitely long text (500K in the author's experiments ).
3. Finally, please note that Temp-Lora is useless in scenarios that do not contain a large amount of text, such as when the context in pre-training is smaller than the window size of the model.
The author comes from a confidential organization
It is worth mentioning that the author did not leave much source information for inventing such a simple and innovative method:
The name of the organization is directly signed "Secret Agency", the names of the three authors also only have full surnames.
#However, judging from the email information, it may be from schools such as City University of Hong Kong and Hong Kong Chinese Language School.
Finally, what do you think of this method?
Paper: https://www.php.cn/link/f74e95cf0ef6ccd85c791b5d351aa327
The above is the detailed content of Anonymous papers come up with surprising ideas! This can actually be done to enhance the long text capabilities of large models. For more information, please follow other related articles on the PHP Chinese website!

Hot AI Tools

Undresser.AI Undress
AI-powered app for creating realistic nude photos

AI Clothes Remover
Online AI tool for removing clothes from photos.

Undress AI Tool
Undress images for free

Clothoff.io
AI clothes remover

Video Face Swap
Swap faces in any video effortlessly with our completely free AI face swap tool!

Hot Article

Hot Tools

Notepad++7.3.1
Easy-to-use and free code editor

SublimeText3 Chinese version
Chinese version, very easy to use

Zend Studio 13.0.1
Powerful PHP integrated development environment

Dreamweaver CS6
Visual web development tools

SublimeText3 Mac version
God-level code editing software (SublimeText3)

Hot Topics
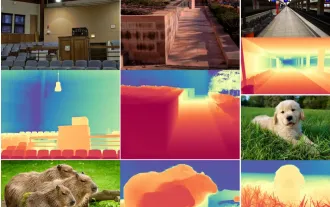
0.What does this article do? We propose DepthFM: a versatile and fast state-of-the-art generative monocular depth estimation model. In addition to traditional depth estimation tasks, DepthFM also demonstrates state-of-the-art capabilities in downstream tasks such as depth inpainting. DepthFM is efficient and can synthesize depth maps within a few inference steps. Let’s read about this work together ~ 1. Paper information title: DepthFM: FastMonocularDepthEstimationwithFlowMatching Author: MingGui, JohannesS.Fischer, UlrichPrestel, PingchuanMa, Dmytr
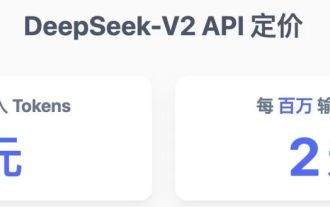
Imagine an artificial intelligence model that not only has the ability to surpass traditional computing, but also achieves more efficient performance at a lower cost. This is not science fiction, DeepSeek-V2[1], the world’s most powerful open source MoE model is here. DeepSeek-V2 is a powerful mixture of experts (MoE) language model with the characteristics of economical training and efficient inference. It consists of 236B parameters, 21B of which are used to activate each marker. Compared with DeepSeek67B, DeepSeek-V2 has stronger performance, while saving 42.5% of training costs, reducing KV cache by 93.3%, and increasing the maximum generation throughput to 5.76 times. DeepSeek is a company exploring general artificial intelligence
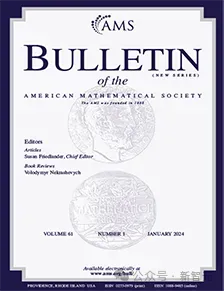
AI is indeed changing mathematics. Recently, Tao Zhexuan, who has been paying close attention to this issue, forwarded the latest issue of "Bulletin of the American Mathematical Society" (Bulletin of the American Mathematical Society). Focusing on the topic "Will machines change mathematics?", many mathematicians expressed their opinions. The whole process was full of sparks, hardcore and exciting. The author has a strong lineup, including Fields Medal winner Akshay Venkatesh, Chinese mathematician Zheng Lejun, NYU computer scientist Ernest Davis and many other well-known scholars in the industry. The world of AI has changed dramatically. You know, many of these articles were submitted a year ago.
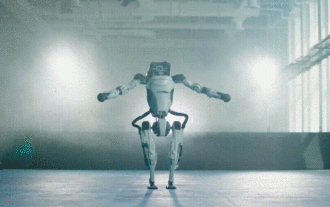
Boston Dynamics Atlas officially enters the era of electric robots! Yesterday, the hydraulic Atlas just "tearfully" withdrew from the stage of history. Today, Boston Dynamics announced that the electric Atlas is on the job. It seems that in the field of commercial humanoid robots, Boston Dynamics is determined to compete with Tesla. After the new video was released, it had already been viewed by more than one million people in just ten hours. The old people leave and new roles appear. This is a historical necessity. There is no doubt that this year is the explosive year of humanoid robots. Netizens commented: The advancement of robots has made this year's opening ceremony look like a human, and the degree of freedom is far greater than that of humans. But is this really not a horror movie? At the beginning of the video, Atlas is lying calmly on the ground, seemingly on his back. What follows is jaw-dropping
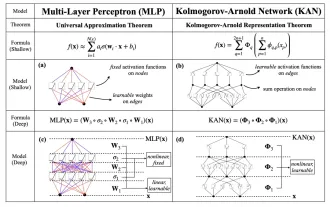
Earlier this month, researchers from MIT and other institutions proposed a very promising alternative to MLP - KAN. KAN outperforms MLP in terms of accuracy and interpretability. And it can outperform MLP running with a larger number of parameters with a very small number of parameters. For example, the authors stated that they used KAN to reproduce DeepMind's results with a smaller network and a higher degree of automation. Specifically, DeepMind's MLP has about 300,000 parameters, while KAN only has about 200 parameters. KAN has a strong mathematical foundation like MLP. MLP is based on the universal approximation theorem, while KAN is based on the Kolmogorov-Arnold representation theorem. As shown in the figure below, KAN has
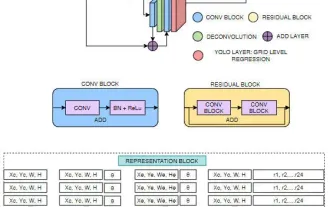
Target detection is a relatively mature problem in autonomous driving systems, among which pedestrian detection is one of the earliest algorithms to be deployed. Very comprehensive research has been carried out in most papers. However, distance perception using fisheye cameras for surround view is relatively less studied. Due to large radial distortion, standard bounding box representation is difficult to implement in fisheye cameras. To alleviate the above description, we explore extended bounding box, ellipse, and general polygon designs into polar/angular representations and define an instance segmentation mIOU metric to analyze these representations. The proposed model fisheyeDetNet with polygonal shape outperforms other models and simultaneously achieves 49.5% mAP on the Valeo fisheye camera dataset for autonomous driving
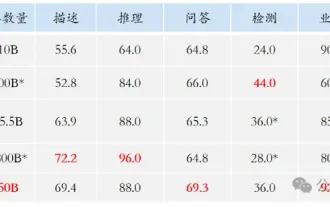
I cry to death. The world is madly building big models. The data on the Internet is not enough. It is not enough at all. The training model looks like "The Hunger Games", and AI researchers around the world are worrying about how to feed these data voracious eaters. This problem is particularly prominent in multi-modal tasks. At a time when nothing could be done, a start-up team from the Department of Renmin University of China used its own new model to become the first in China to make "model-generated data feed itself" a reality. Moreover, it is a two-pronged approach on the understanding side and the generation side. Both sides can generate high-quality, multi-modal new data and provide data feedback to the model itself. What is a model? Awaker 1.0, a large multi-modal model that just appeared on the Zhongguancun Forum. Who is the team? Sophon engine. Founded by Gao Yizhao, a doctoral student at Renmin University’s Hillhouse School of Artificial Intelligence.
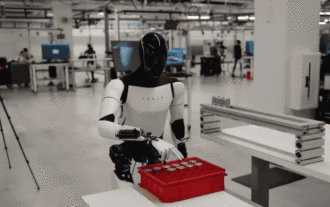
The latest video of Tesla's robot Optimus is released, and it can already work in the factory. At normal speed, it sorts batteries (Tesla's 4680 batteries) like this: The official also released what it looks like at 20x speed - on a small "workstation", picking and picking and picking: This time it is released One of the highlights of the video is that Optimus completes this work in the factory, completely autonomously, without human intervention throughout the process. And from the perspective of Optimus, it can also pick up and place the crooked battery, focusing on automatic error correction: Regarding Optimus's hand, NVIDIA scientist Jim Fan gave a high evaluation: Optimus's hand is the world's five-fingered robot. One of the most dexterous. Its hands are not only tactile
