


Breaking through the resolution limit: Byte and the University of Science and Technology of China reveal a large multi-modal document model
Now there are even large multi-modal high-resolution documents!
This technology can not only accurately identify the information in the image, but also call its own knowledge base to answer questions according to user needs
For example, when you see Mario’s interface in the picture, you can directly The answer is that this is a work of Nintendo.
This model was jointly researched by ByteDance and the University of Science and Technology of China, and was uploaded to arXiv on November 24, 2023
Study here , the author team proposed DocPedia, a unified high-resolution multi-modal document large model DocPedia.
In this study, the author used a new way to solve the shortcoming of existing models that cannot parse high-resolution document images.
DocPedia has a resolution of up to 2560×2560, but currently the industry’s advanced multi-modal large models such as LLaVA and MiniGPT-4 have an upper limit of image resolution of 336×336, which makes them unable to parse high-resolution document images.
So, how does this model perform and what kind of optimization method is used?
Significant improvement in various evaluation scores
In this paper, the author shows an example of DocPedia high-resolution image and text understanding. It can be observed that DocPedia has the ability to understand the content of instructions and accurately extract relevant graphic and text information from high-resolution document images and natural scene images
For example, in this set of pictures, DocPedia easily mines from the pictures With text information such as license plate number and computer configuration, even handwritten text can be accurately judged.
Combined with the text information in the image, DocPedia can also use large model reasoning capabilities to analyze problems based on context.
After reading the image information, DocPedia will also answer the extended content not shown in the image based on its rich world knowledge base
The following table quantitatively compares some existing multi-modal large models with DocPedia’s key information extraction (KIE) and visual question answering (VQA) capabilities.
By increasing the resolution and adopting effective training methods, we can see that DocPedia has achieved significant improvements on various test benchmarks
So, how does DocPedia achieve such an effect?
Solving the resolution problem from the frequency domain
DocPedia’s training is divided into two stages: pre-training and fine-tuning. In order to train DocPedia, the author team collected a large amount of graphic data containing various types of documents and built an instruction fine-tuning data set.
In the pre-training stage, the large language model will be frozen, and only the part of the visual encoder is optimized so that its output token representation space is consistent with the large language model
At this stage , the author team proposed to mainly train DocPedia’s perception capabilities, including the perception of text and natural scenes
The pre-training tasks include text detection, text recognition, end-to-end OCR, paragraph reading, full-text reading, and image text description .
In the fine-tuning phase, the large language model is unfrozen and end-to-end overall optimization is performed.
The author team proposed a perception-understanding joint training strategy: based on the original low-level perception tasks, add This joint perception-understanding training strategy further improves the performance of DocPedia.
In terms of strategy for resolution issues, unlike existing methods, DocPedia solves it from the
perspective. When processing high-resolution document images, DocPedia will first extract its DCT coefficient matrix. This matrix can downsample the spatial resolution by 8 times without losing the textual information of the original image.
After this step, we will use the cascaded frequency domain adapter (Frequency Adapter) Pass the input signal to the Vision Encoder for deeper resolution compression and feature extraction
With this method, a 2560×2560 image can be represented by 1600 tokens.
Compared with directly inputting the original image into a visual encoder (such as Swin Transformer), this method reduces the number of tokens by 4 times.
Finally, these tokens are spliced with the tokens converted from the instructions in the sequence dimension and input into the large model for answer.
The results of the ablation experiment show that improving resolution and performing joint perception-understanding fine-tuning are two important factors to improve DocPedia performance
The following figure compares DocPedia's answer to a paper image and the same command under different input scales. It can be seen that DocPedia answers correctly if and only if the resolution is increased to 2560×2560.
The figure below compares DocPedia’s model responses to the same scene text image and the same instruction under different fine-tuning strategies.
It can be seen from this example that the model that has been jointly fine-tuned by perception and understanding can accurately perform text recognition and semantic question answering
##Please Click the following link to view the paper: https://arxiv.org/abs/2311.11810
The above is the detailed content of Breaking through the resolution limit: Byte and the University of Science and Technology of China reveal a large multi-modal document model. For more information, please follow other related articles on the PHP Chinese website!

Hot AI Tools

Undresser.AI Undress
AI-powered app for creating realistic nude photos

AI Clothes Remover
Online AI tool for removing clothes from photos.

Undress AI Tool
Undress images for free

Clothoff.io
AI clothes remover

Video Face Swap
Swap faces in any video effortlessly with our completely free AI face swap tool!

Hot Article

Hot Tools

Notepad++7.3.1
Easy-to-use and free code editor

SublimeText3 Chinese version
Chinese version, very easy to use

Zend Studio 13.0.1
Powerful PHP integrated development environment

Dreamweaver CS6
Visual web development tools

SublimeText3 Mac version
God-level code editing software (SublimeText3)

Hot Topics
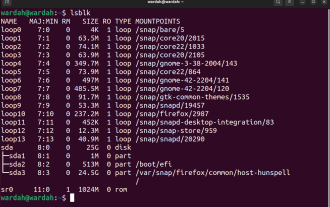
DDREASE is a tool for recovering data from file or block devices such as hard drives, SSDs, RAM disks, CDs, DVDs and USB storage devices. It copies data from one block device to another, leaving corrupted data blocks behind and moving only good data blocks. ddreasue is a powerful recovery tool that is fully automated as it does not require any interference during recovery operations. Additionally, thanks to the ddasue map file, it can be stopped and resumed at any time. Other key features of DDREASE are as follows: It does not overwrite recovered data but fills the gaps in case of iterative recovery. However, it can be truncated if the tool is instructed to do so explicitly. Recover data from multiple files or blocks to a single
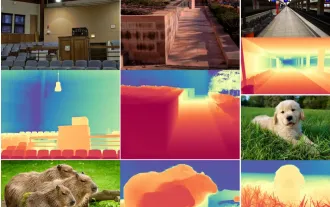
0.What does this article do? We propose DepthFM: a versatile and fast state-of-the-art generative monocular depth estimation model. In addition to traditional depth estimation tasks, DepthFM also demonstrates state-of-the-art capabilities in downstream tasks such as depth inpainting. DepthFM is efficient and can synthesize depth maps within a few inference steps. Let’s read about this work together ~ 1. Paper information title: DepthFM: FastMonocularDepthEstimationwithFlowMatching Author: MingGui, JohannesS.Fischer, UlrichPrestel, PingchuanMa, Dmytr
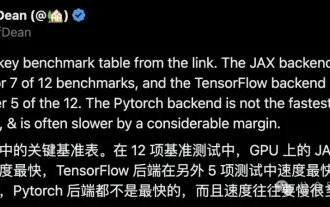
The performance of JAX, promoted by Google, has surpassed that of Pytorch and TensorFlow in recent benchmark tests, ranking first in 7 indicators. And the test was not done on the TPU with the best JAX performance. Although among developers, Pytorch is still more popular than Tensorflow. But in the future, perhaps more large models will be trained and run based on the JAX platform. Models Recently, the Keras team benchmarked three backends (TensorFlow, JAX, PyTorch) with the native PyTorch implementation and Keras2 with TensorFlow. First, they select a set of mainstream
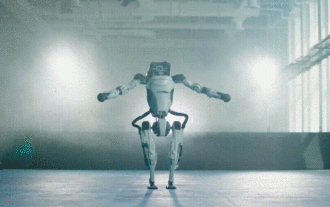
Boston Dynamics Atlas officially enters the era of electric robots! Yesterday, the hydraulic Atlas just "tearfully" withdrew from the stage of history. Today, Boston Dynamics announced that the electric Atlas is on the job. It seems that in the field of commercial humanoid robots, Boston Dynamics is determined to compete with Tesla. After the new video was released, it had already been viewed by more than one million people in just ten hours. The old people leave and new roles appear. This is a historical necessity. There is no doubt that this year is the explosive year of humanoid robots. Netizens commented: The advancement of robots has made this year's opening ceremony look like a human, and the degree of freedom is far greater than that of humans. But is this really not a horror movie? At the beginning of the video, Atlas is lying calmly on the ground, seemingly on his back. What follows is jaw-dropping
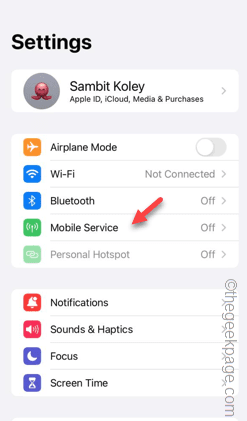
Facing lag, slow mobile data connection on iPhone? Typically, the strength of cellular internet on your phone depends on several factors such as region, cellular network type, roaming type, etc. There are some things you can do to get a faster, more reliable cellular Internet connection. Fix 1 – Force Restart iPhone Sometimes, force restarting your device just resets a lot of things, including the cellular connection. Step 1 – Just press the volume up key once and release. Next, press the Volume Down key and release it again. Step 2 – The next part of the process is to hold the button on the right side. Let the iPhone finish restarting. Enable cellular data and check network speed. Check again Fix 2 – Change data mode While 5G offers better network speeds, it works better when the signal is weaker
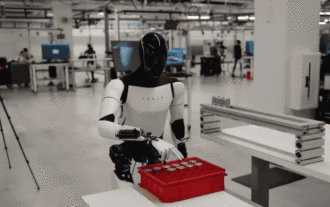
The latest video of Tesla's robot Optimus is released, and it can already work in the factory. At normal speed, it sorts batteries (Tesla's 4680 batteries) like this: The official also released what it looks like at 20x speed - on a small "workstation", picking and picking and picking: This time it is released One of the highlights of the video is that Optimus completes this work in the factory, completely autonomously, without human intervention throughout the process. And from the perspective of Optimus, it can also pick up and place the crooked battery, focusing on automatic error correction: Regarding Optimus's hand, NVIDIA scientist Jim Fan gave a high evaluation: Optimus's hand is the world's five-fingered robot. One of the most dexterous. Its hands are not only tactile
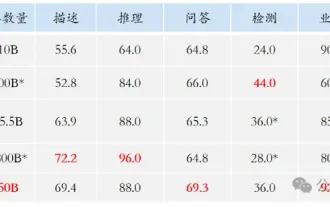
I cry to death. The world is madly building big models. The data on the Internet is not enough. It is not enough at all. The training model looks like "The Hunger Games", and AI researchers around the world are worrying about how to feed these data voracious eaters. This problem is particularly prominent in multi-modal tasks. At a time when nothing could be done, a start-up team from the Department of Renmin University of China used its own new model to become the first in China to make "model-generated data feed itself" a reality. Moreover, it is a two-pronged approach on the understanding side and the generation side. Both sides can generate high-quality, multi-modal new data and provide data feedback to the model itself. What is a model? Awaker 1.0, a large multi-modal model that just appeared on the Zhongguancun Forum. Who is the team? Sophon engine. Founded by Gao Yizhao, a doctoral student at Renmin University’s Hillhouse School of Artificial Intelligence.
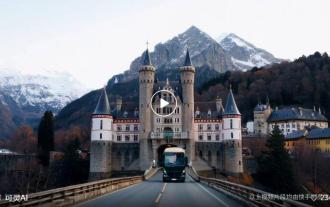
What? Is Zootopia brought into reality by domestic AI? Exposed together with the video is a new large-scale domestic video generation model called "Keling". Sora uses a similar technical route and combines a number of self-developed technological innovations to produce videos that not only have large and reasonable movements, but also simulate the characteristics of the physical world and have strong conceptual combination capabilities and imagination. According to the data, Keling supports the generation of ultra-long videos of up to 2 minutes at 30fps, with resolutions up to 1080p, and supports multiple aspect ratios. Another important point is that Keling is not a demo or video result demonstration released by the laboratory, but a product-level application launched by Kuaishou, a leading player in the short video field. Moreover, the main focus is to be pragmatic, not to write blank checks, and to go online as soon as it is released. The large model of Ke Ling is already available in Kuaiying.
