Exciting! A preliminary study of GPT-4V in autonomous driving
Update: Added a new example, the self-driving delivery vehicle drove into the Xinpu cement floor
Under the spotlight, GPT4 finally launched vision-related functions today. This afternoon I quickly tested GPT's image perception capabilities with my friends. Although we had expectations, we were still greatly shocked. TL;DR isI think that the semantic-related issues in autonomous driving should have been solved very well by large models, but the credibility and spatial perception capabilities of large models are still not satisfactory. It should be more than enough to solve some so-called efficiency-related corner cases, but it is still very far away to completely rely on large models to independently complete driving and ensure safety.
1 Example1: Some unknown obstacles appeared on the road
GPT4's description
Accurate part: 3 trucks were detected, the license plate number of the front truck is basically correct (ignore if there are Chinese characters), the weather and environment are correct, accurate without prompts Unknown obstacles ahead were identified
Inaccurate part: The position of the third truck is indistinguishable from left to right, and the text on the top of the second truck is a random guess (because of insufficient resolution?)
This is not enough, let us continue to give a little hint to ask what this object is and whether it can be pressed over.
Impressive! We have tested multiple similar scenarios, and the performance on unknown obstacles can be said to be very amazing.
2 Example2: Understanding road water accumulation
There is no prompt to automatically recognize the sign. This should It was gay and we continued to give some hints
We were shocked again. . . He could automatically tell the fog behind the truck and also mentioned the puddle, but once again said the direction was to the left. . . I feel that some prompt engineering may be needed here to better enable GPT to output the position and direction.
3 Example3: A vehicle turned around and hit the guardrail directly
Enter the first frame , because there is no timing information, the truck on the right is just regarded as parked. So here’s another frame:
can be said automatically. These two broke through the guardrail and hovered at the edge of the highway. It’s great. . . But instead the road signs that looked easier were wrong. . . All I can say is that this is a huge model. It will always shock you and you never know when it will make you cry. . . Another frame:
#This time, it talks directly about the debris on the road, and I admire it again. . . But once I named the arrow on the road wrong. . . Generally speaking, the information that needs special attention in this scene is covered. For issues like road signs, the flaws are not hidden
4 Example4: Let’s have a funny
It can only be said that it is very accurate. Compared with the previous cases that seemed extremely difficult, such as "someone waved his hand at you", it is like pediatrics. The semantics The corner case above can be solved.
5 Example5 Let’s have a famous scene. . . The delivery truck mistakenly entered the newly constructed road
##
I was relatively conservative at the beginning and did not directly guess the reason. I gave a variety of guesses. This is in line with the goal of alignment. After using CoT, it was discovered that the problem was that the car was not understood to be a self-driving vehicle, so giving this information through prompt can give more accurate information. Finally, through a bunch of prompts, the conclusion can be output that the newly laid asphalt is not suitable for driving. The final result is still OK, but the process is more tortuous and requires more prompt engineering and careful design. This reason may also be because it is not a first-person perspective picture and can only be speculated from a third-person perspective. So this example is not very precise.
6 Summary
Some quick attempts have fully proved the power and generalization performance of GPT4V. Appropriate prompts should be able to fully demonstrate The strength of GPT4V. Solving the semantic corner case should be very promising, but the problem of illusion will still plague some applications in security-related scenarios. Very exciting. I personally think that the rational use of such large models can greatly accelerate the development of L4 and even L5 autonomous driving. However, does LLM have to drive directly? End-to-end driving, in particular, remains a debatable issue. I have been thinking a lot recently, so I will find time to write an article and chat with you all~
Original link: https://mp.weixin.qq.com/s/RtEek6HadErxXLSdtsMWHQ
The above is the detailed content of Exciting! A preliminary study of GPT-4V in autonomous driving. For more information, please follow other related articles on the PHP Chinese website!

Hot AI Tools

Undresser.AI Undress
AI-powered app for creating realistic nude photos

AI Clothes Remover
Online AI tool for removing clothes from photos.

Undress AI Tool
Undress images for free

Clothoff.io
AI clothes remover

Video Face Swap
Swap faces in any video effortlessly with our completely free AI face swap tool!

Hot Article

Hot Tools

Notepad++7.3.1
Easy-to-use and free code editor

SublimeText3 Chinese version
Chinese version, very easy to use

Zend Studio 13.0.1
Powerful PHP integrated development environment

Dreamweaver CS6
Visual web development tools

SublimeText3 Mac version
God-level code editing software (SublimeText3)

Hot Topics
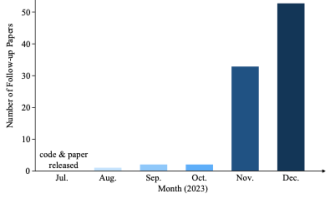
Written above & the author’s personal understanding Three-dimensional Gaussiansplatting (3DGS) is a transformative technology that has emerged in the fields of explicit radiation fields and computer graphics in recent years. This innovative method is characterized by the use of millions of 3D Gaussians, which is very different from the neural radiation field (NeRF) method, which mainly uses an implicit coordinate-based model to map spatial coordinates to pixel values. With its explicit scene representation and differentiable rendering algorithms, 3DGS not only guarantees real-time rendering capabilities, but also introduces an unprecedented level of control and scene editing. This positions 3DGS as a potential game-changer for next-generation 3D reconstruction and representation. To this end, we provide a systematic overview of the latest developments and concerns in the field of 3DGS for the first time.
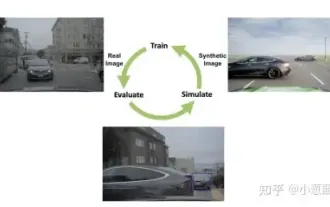
Yesterday during the interview, I was asked whether I had done any long-tail related questions, so I thought I would give a brief summary. The long-tail problem of autonomous driving refers to edge cases in autonomous vehicles, that is, possible scenarios with a low probability of occurrence. The perceived long-tail problem is one of the main reasons currently limiting the operational design domain of single-vehicle intelligent autonomous vehicles. The underlying architecture and most technical issues of autonomous driving have been solved, and the remaining 5% of long-tail problems have gradually become the key to restricting the development of autonomous driving. These problems include a variety of fragmented scenarios, extreme situations, and unpredictable human behavior. The "long tail" of edge scenarios in autonomous driving refers to edge cases in autonomous vehicles (AVs). Edge cases are possible scenarios with a low probability of occurrence. these rare events
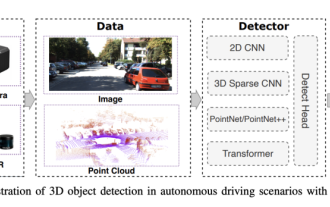
0.Written in front&& Personal understanding that autonomous driving systems rely on advanced perception, decision-making and control technologies, by using various sensors (such as cameras, lidar, radar, etc.) to perceive the surrounding environment, and using algorithms and models for real-time analysis and decision-making. This enables vehicles to recognize road signs, detect and track other vehicles, predict pedestrian behavior, etc., thereby safely operating and adapting to complex traffic environments. This technology is currently attracting widespread attention and is considered an important development area in the future of transportation. one. But what makes autonomous driving difficult is figuring out how to make the car understand what's going on around it. This requires that the three-dimensional object detection algorithm in the autonomous driving system can accurately perceive and describe objects in the surrounding environment, including their locations,
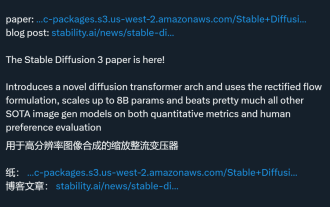
StableDiffusion3’s paper is finally here! This model was released two weeks ago and uses the same DiT (DiffusionTransformer) architecture as Sora. It caused quite a stir once it was released. Compared with the previous version, the quality of the images generated by StableDiffusion3 has been significantly improved. It now supports multi-theme prompts, and the text writing effect has also been improved, and garbled characters no longer appear. StabilityAI pointed out that StableDiffusion3 is a series of models with parameter sizes ranging from 800M to 8B. This parameter range means that the model can be run directly on many portable devices, significantly reducing the use of AI
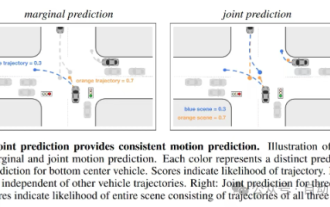
Trajectory prediction plays an important role in autonomous driving. Autonomous driving trajectory prediction refers to predicting the future driving trajectory of the vehicle by analyzing various data during the vehicle's driving process. As the core module of autonomous driving, the quality of trajectory prediction is crucial to downstream planning control. The trajectory prediction task has a rich technology stack and requires familiarity with autonomous driving dynamic/static perception, high-precision maps, lane lines, neural network architecture (CNN&GNN&Transformer) skills, etc. It is very difficult to get started! Many fans hope to get started with trajectory prediction as soon as possible and avoid pitfalls. Today I will take stock of some common problems and introductory learning methods for trajectory prediction! Introductory related knowledge 1. Are the preview papers in order? A: Look at the survey first, p

Original title: SIMPL: ASimpleandEfficientMulti-agentMotionPredictionBaselineforAutonomousDriving Paper link: https://arxiv.org/pdf/2402.02519.pdf Code link: https://github.com/HKUST-Aerial-Robotics/SIMPL Author unit: Hong Kong University of Science and Technology DJI Paper idea: This paper proposes a simple and efficient motion prediction baseline (SIMPL) for autonomous vehicles. Compared with traditional agent-cent
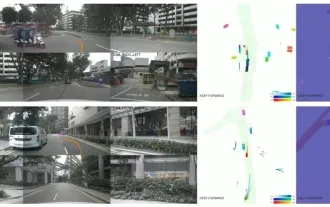
In the past month, due to some well-known reasons, I have had very intensive exchanges with various teachers and classmates in the industry. An inevitable topic in the exchange is naturally end-to-end and the popular Tesla FSDV12. I would like to take this opportunity to sort out some of my thoughts and opinions at this moment for your reference and discussion. How to define an end-to-end autonomous driving system, and what problems should be expected to be solved end-to-end? According to the most traditional definition, an end-to-end system refers to a system that inputs raw information from sensors and directly outputs variables of concern to the task. For example, in image recognition, CNN can be called end-to-end compared to the traditional feature extractor + classifier method. In autonomous driving tasks, input data from various sensors (camera/LiDAR
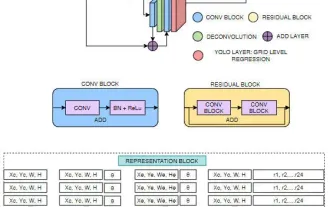
Target detection is a relatively mature problem in autonomous driving systems, among which pedestrian detection is one of the earliest algorithms to be deployed. Very comprehensive research has been carried out in most papers. However, distance perception using fisheye cameras for surround view is relatively less studied. Due to large radial distortion, standard bounding box representation is difficult to implement in fisheye cameras. To alleviate the above description, we explore extended bounding box, ellipse, and general polygon designs into polar/angular representations and define an instance segmentation mIOU metric to analyze these representations. The proposed model fisheyeDetNet with polygonal shape outperforms other models and simultaneously achieves 49.5% mAP on the Valeo fisheye camera dataset for autonomous driving
