


Explore training techniques for open-world test segments using self-training methods with dynamic prototyping extensions
Improving the generalization ability of the model is an important basis for promoting the implementation of vision-based perception methods. Test-Time Training/Adaptation (Test-Time Training/Adaptation) generalizes the model to Unknown target domain data distribution segment. Existing TTT/TTA methods usually focus on improving test segment training performance under target domain data in the closed-loop world.
However, in many application scenarios, the target domain is easily contaminated by strong out-of-domain data (Strong OOD), such as data unrelated to semantic categories. This scenario is also known as Open World Test Segment Training (OWTTT). In this case, existing TTT/TTA usually forces the classification of strong out-of-domain data into known categories, thereby ultimately interfering with the ability to resolve weak out-of-domain data (Weak OOD) such as images affected by noise
Recently, South China University of Technology and the A*STAR team proposed the setting of open world test segment training for the first time, and launched corresponding training methods
- Paper: https://arxiv.org/abs/2308.09942
- Code: https://github.com/Yushu-Li/OWTTT
This paper first proposes a strong out-of-domain data sample filtering method with adaptive threshold to improve the robustness of the self-training TTT method in the open world. The method further proposes a method to characterize strong out-of-domain samples based on dynamically extended prototypes to improve the weak/strong out-of-domain data separation effect. Finally, self-training is constrained by distribution alignment
The method in this study achieved the best performance on 5 different OWTTT benchmarks, and opened up new directions for subsequent research on TTT. New directions for more robust TTT methods. This research has been accepted as an oral presentation paper at ICCV 2023
Introduction
Test segment training (TTT) can access the target domain only during the inference phase data and perform on-the-fly inference on test data with distribution shifts. The success of TTT has been demonstrated on a number of artificially selected synthetically corrupted target domain data. However, the capability boundaries of existing TTT methods have not been fully explored.
To promote TTT applications in open scenarios, the focus of research has shifted to investigating scenarios where TTT methods may fail. Many efforts have been made to develop stable and robust TTT methods in more realistic open-world environments. In this work, we delve into a common but overlooked open-world scenario, where the target domain may contain test data distributions drawn from significantly different environments, such as different semantic categories than the source domain, or simply random noise.
We call the above test data strong out-of-distribution data (strong OOD). What is called weak OOD data in this work is test data with distribution shifts, such as common synthetic damage. Therefore, the lack of existing work on this realistic environment motivates us to explore improving the robustness of Open World Test Segment Training (OWTTT), where the test data is contaminated by strong OOD samples.
Figure 1: Evaluation results of the existing TTT method under OWTTT settings
As shown As shown in Figure 1, we first evaluate existing TTT methods under the OWTTT setting and find that TTT methods through self-training and distribution alignment will be affected by strong OOD samples. These results demonstrate that safe test-time training in the open world cannot be achieved by applying existing TTT techniques. We attribute their failure to the following two reasons.
- Self-training-based TTT has difficulty handling strong OOD samples because it must assign test samples to known classes. Although some low-confidence samples can be filtered out by applying the threshold employed in semi-supervised learning, there is still no guarantee that all strong OOD samples will be filtered out.
- Methods based on distribution alignment will be affected when strong OOD samples are calculated to estimate the target domain distribution. Both global distribution alignment [1] and class distribution alignment [2] can be affected and lead to inaccurate feature distribution alignment.
In order to solve the potential reasons for the failure of existing TTT methods, we propose a method that combines two technologies to improve the robustness of open-world TTT under a self-training framework.
First, we construct the baseline of TTT on the self-trained variant, that is, clustering in the target domain with the source domain prototype as the cluster center. To mitigate the impact of self-training on strong OOD with incorrect pseudo-labels, we design a hyperparameter-free method to reject strong OOD samples.
To further separate the characteristics of weak OOD samples and strong OOD samples, we allow the prototype pool to expand by selecting isolated strong OOD samples. Therefore, self-training will allow strong OOD samples to form tight clusters around the newly expanded strong OOD prototype. This will facilitate distribution alignment between source and target domains. We further propose to regularize self-training through global distribution alignment to reduce the risk of confirmation bias.
Finally, in order to synthesize the open-world TTT scenario, we use CIFAR10-C, CIFAR100-C, ImageNet-C, VisDA-C, ImageNet-R, Tiny-ImageNet, MNIST and SVHN dataset, and establish a benchmark dataset by utilizing one dataset as weak OOD and the other as strong OOD. We refer to this benchmark as the Open World Test Segment Training Benchmark and hope that this encourages more future work to focus on the robustness of test segment training in more realistic scenarios.
Method
The paper divides the proposed method into four parts to introduce it
1) Overview of the settings of the training tasks in the test segment under the open world.
#2) Describes how to implement TTT by rewriting the content as: cluster analysis and how to extend the prototype for open world test-time training.
3) Introduces how to use target domain data for dynamic prototype expansion.
4) Introduce Distribution alignment combined with rewritten content: cluster analysis to achieve powerful open-world test-time training.
Figure 2: Method overview diagram
Task setting
The purpose of TTT is to adapt the source domain pre-trained model to the target domain, where the target domain may have distributed migration relative to the source domain. In standard closed-world TTT, the label spaces of the source and target domains are the same. However, in the open world TTT, the label space of the target domain contains the target space of the source domain, which means that the target domain has unseen new semantic categories
In order to avoid the gap between TTT definitions For confusion, we adopt the sequential test time training (sTTT) protocol proposed in TTAC [2] for evaluation. Under the sTTT protocol, test samples are tested sequentially, and model updates are performed after observing small batches of test samples. The prediction for any test sample arriving at timestamp t is not affected by any test sample arriving at t k (whose k is greater than 0).
Rewritten content as: Cluster analysis
Inspired by work using clustering in domain adaptation tasks [ 3, 4], we treat test segment training as discovering cluster structures in the target domain data. By identifying representative prototypes as cluster centers, cluster structures are identified in the target domain and test samples are encouraged to embed near one of the prototypes. The rewritten content is: The goal of cluster analysis is defined as minimizing the negative log-likelihood loss of the cosine similarity between the sample and the cluster center, as shown in the following formula.
We developed a hyperparameter-free method to filter out strong OOD samples to avoid the negative impact of adjusting model weights. Specifically, we define a strong OOD score os for each test sample as the highest similarity to the source domain prototype, as shown in the following equation.
Figure 3 Outliers present a bimodal distribution
We observe that the outliers follow a bimodal distribution, as shown in Figure 3. Therefore, instead of specifying a fixed threshold, we define the optimal threshold as the best value that separates the two distributions. Specifically, the problem can be formulated as dividing the outliers into two clusters, and the optimal threshold will minimize the within-cluster variance in . Optimizing the following equation can be efficiently achieved by exhaustively searching all possible thresholds from 0 to 1 in steps of 0.01.
Dynamic prototype expansion
Expanding the strong OOD prototype pool needs to be considered at the same time Source domain and strong OOD prototypes to evaluate test samples. To dynamically estimate the number of clusters from data, previous studies have investigated similar problems. The deterministic hard clustering algorithm DP-means [5] was developed by measuring the distance of data points to known cluster centers, and a new cluster is initialized when the distance is above a threshold. DP-means is shown to be equivalent to optimizing the K-means objective but with an additional penalty on the number of clusters, providing a feasible solution for dynamic prototype expansion.
To alleviate the difficulty of estimating additional hyperparameters, we first define a test sample with an extended strong OOD score as the closest distance to the existing source domain prototype and the strong OOD prototype, As follows. Therefore, testing samples above this threshold will build a new prototype. To avoid adding nearby test samples, we incrementally repeat this prototype expansion process.
As other strong OOD prototypes are identified, we define the rewrite for the test sample as: cluster analysis loss, and consider two factors. First, test samples classified into known classes should be embedded closer to prototypes and farther away from other prototypes, which defines the K-class classification task. Second, test samples classified as strong OOD prototypes should be far away from any source domain prototypes, which defines the K 1 class classification task. With these goals in mind, we will rewrite the content as: The cluster analysis loss is defined as follows.
Distributed alignment constraints mean that elements in a design or layout are required to be arranged and aligned in a specific way. This constraint can be applied to a variety of different scenarios, including web design, graphic design, and space layout. By using distributed alignment constraints, the relationship between elements can be made clearer and more unified, improving the aesthetics and readability of the overall design
It is well known that self-training is susceptible to errors The impact of pseudo-labeling. The situation is worsened when the target domain consists of OOD samples. To reduce the risk of failure, we further use distribution alignment [1] as a regularization for self-training, as follows.
Experiments
We tested on 5 different OWTTT benchmark datasets, including Synthetically corrupted datasets and style-varying datasets. The experiment mainly uses three evaluation indicators: weak OOD classification accuracy ACCS, strong OOD classification accuracy ACCN and the harmonic mean of the two ACCH
# #The content that needs to be rewritten is: Cifar10-C The performance of different methods in the data set is shown in the table below
The content that needs to be rewritten is: Cifar100 The performance of different methods in the -C data set is shown in the following table:
The content that needs to be rewritten is: on the ImageNet-C data set, different The performance of the method is shown in the following table
Table 4 Performance of different methods on the ImageNet-R data set
Table 5 The performance of different methods on the VisDA-C data set
Our method has significantly improved compared to the current best methods on almost all data sets, as shown in the table above . It can effectively identify strong OOD samples and reduce the impact on the classification of weak OOD samples. Therefore, in an open world scenario, our method can achieve a more robust TTT
Summary
This paper proposes an open world for the first time Problems and settings of test segment training (OWTTT), pointing out that existing methods will encounter difficulties when processing target domain data containing strong OOD samples that have semantic offsets from source domain samples, and proposes a dynamic prototype extension-based The self-training method solves the above problems. We hope that this work can provide new directions for subsequent research on TTT to explore more robust TTT methods
The above is the detailed content of Explore training techniques for open-world test segments using self-training methods with dynamic prototyping extensions. For more information, please follow other related articles on the PHP Chinese website!

Hot AI Tools

Undresser.AI Undress
AI-powered app for creating realistic nude photos

AI Clothes Remover
Online AI tool for removing clothes from photos.

Undress AI Tool
Undress images for free

Clothoff.io
AI clothes remover

Video Face Swap
Swap faces in any video effortlessly with our completely free AI face swap tool!

Hot Article

Hot Tools

Notepad++7.3.1
Easy-to-use and free code editor

SublimeText3 Chinese version
Chinese version, very easy to use

Zend Studio 13.0.1
Powerful PHP integrated development environment

Dreamweaver CS6
Visual web development tools

SublimeText3 Mac version
God-level code editing software (SublimeText3)

Hot Topics
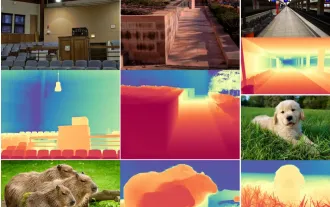
0.What does this article do? We propose DepthFM: a versatile and fast state-of-the-art generative monocular depth estimation model. In addition to traditional depth estimation tasks, DepthFM also demonstrates state-of-the-art capabilities in downstream tasks such as depth inpainting. DepthFM is efficient and can synthesize depth maps within a few inference steps. Let’s read about this work together ~ 1. Paper information title: DepthFM: FastMonocularDepthEstimationwithFlowMatching Author: MingGui, JohannesS.Fischer, UlrichPrestel, PingchuanMa, Dmytr
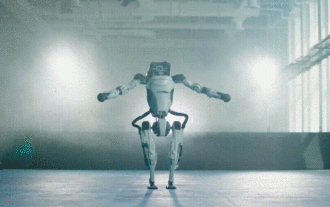
Boston Dynamics Atlas officially enters the era of electric robots! Yesterday, the hydraulic Atlas just "tearfully" withdrew from the stage of history. Today, Boston Dynamics announced that the electric Atlas is on the job. It seems that in the field of commercial humanoid robots, Boston Dynamics is determined to compete with Tesla. After the new video was released, it had already been viewed by more than one million people in just ten hours. The old people leave and new roles appear. This is a historical necessity. There is no doubt that this year is the explosive year of humanoid robots. Netizens commented: The advancement of robots has made this year's opening ceremony look like a human, and the degree of freedom is far greater than that of humans. But is this really not a horror movie? At the beginning of the video, Atlas is lying calmly on the ground, seemingly on his back. What follows is jaw-dropping
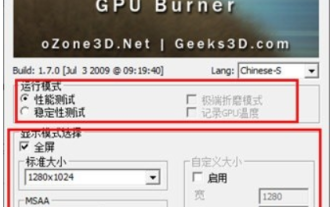
What do you think of furmark? 1. Set the "Run Mode" and "Display Mode" in the main interface, and also adjust the "Test Mode" and click the "Start" button. 2. After waiting for a while, you will see the test results, including various parameters of the graphics card. How is furmark qualified? 1. Use a furmark baking machine and check the results for about half an hour. It basically hovers around 85 degrees, with a peak value of 87 degrees and room temperature of 19 degrees. Large chassis, 5 chassis fan ports, two on the front, two on the top, and one on the rear, but only one fan is installed. All accessories are not overclocked. 2. Under normal circumstances, the normal temperature of the graphics card should be between "30-85℃". 3. Even in summer when the ambient temperature is too high, the normal temperature is "50-85℃
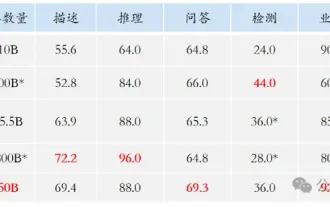
I cry to death. The world is madly building big models. The data on the Internet is not enough. It is not enough at all. The training model looks like "The Hunger Games", and AI researchers around the world are worrying about how to feed these data voracious eaters. This problem is particularly prominent in multi-modal tasks. At a time when nothing could be done, a start-up team from the Department of Renmin University of China used its own new model to become the first in China to make "model-generated data feed itself" a reality. Moreover, it is a two-pronged approach on the understanding side and the generation side. Both sides can generate high-quality, multi-modal new data and provide data feedback to the model itself. What is a model? Awaker 1.0, a large multi-modal model that just appeared on the Zhongguancun Forum. Who is the team? Sophon engine. Founded by Gao Yizhao, a doctoral student at Renmin University’s Hillhouse School of Artificial Intelligence.
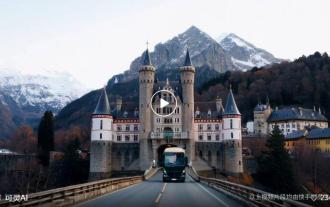
What? Is Zootopia brought into reality by domestic AI? Exposed together with the video is a new large-scale domestic video generation model called "Keling". Sora uses a similar technical route and combines a number of self-developed technological innovations to produce videos that not only have large and reasonable movements, but also simulate the characteristics of the physical world and have strong conceptual combination capabilities and imagination. According to the data, Keling supports the generation of ultra-long videos of up to 2 minutes at 30fps, with resolutions up to 1080p, and supports multiple aspect ratios. Another important point is that Keling is not a demo or video result demonstration released by the laboratory, but a product-level application launched by Kuaishou, a leading player in the short video field. Moreover, the main focus is to be pragmatic, not to write blank checks, and to go online as soon as it is released. The large model of Ke Ling is already available in Kuaiying.
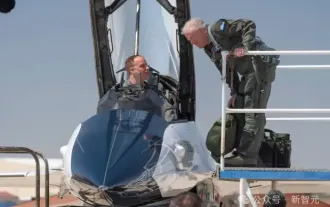
Recently, the military circle has been overwhelmed by the news: US military fighter jets can now complete fully automatic air combat using AI. Yes, just recently, the US military’s AI fighter jet was made public for the first time and the mystery was unveiled. The full name of this fighter is the Variable Stability Simulator Test Aircraft (VISTA). It was personally flown by the Secretary of the US Air Force to simulate a one-on-one air battle. On May 2, U.S. Air Force Secretary Frank Kendall took off in an X-62AVISTA at Edwards Air Force Base. Note that during the one-hour flight, all flight actions were completed autonomously by AI! Kendall said - "For the past few decades, we have been thinking about the unlimited potential of autonomous air-to-air combat, but it has always seemed out of reach." However now,
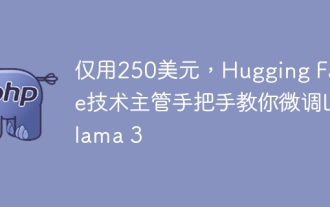
The familiar open source large language models such as Llama3 launched by Meta, Mistral and Mixtral models launched by MistralAI, and Jamba launched by AI21 Lab have become competitors of OpenAI. In most cases, users need to fine-tune these open source models based on their own data to fully unleash the model's potential. It is not difficult to fine-tune a large language model (such as Mistral) compared to a small one using Q-Learning on a single GPU, but efficient fine-tuning of a large model like Llama370b or Mixtral has remained a challenge until now. Therefore, Philipp Sch, technical director of HuggingFace
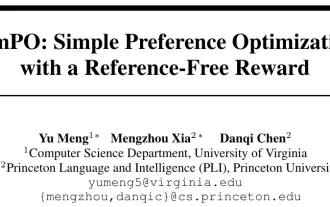
In order to align large language models (LLMs) with human values and intentions, it is critical to learn human feedback to ensure that they are useful, honest, and harmless. In terms of aligning LLM, an effective method is reinforcement learning based on human feedback (RLHF). Although the results of the RLHF method are excellent, there are some optimization challenges involved. This involves training a reward model and then optimizing a policy model to maximize that reward. Recently, some researchers have explored simpler offline algorithms, one of which is direct preference optimization (DPO). DPO learns the policy model directly based on preference data by parameterizing the reward function in RLHF, thus eliminating the need for an explicit reward model. This method is simple and stable
