


3D scene generation: Generate diverse results from a single sample without any neural network training
Diverse and high-quality three-dimensional scene generation results
- Paper address: https://arxiv.org/abs/2304.12670
- ## Project homepage: http://weiyuli.xyz/Sin3DGen/
Using artificial intelligence-assisted content generation (AIGC), a large amount of work has emerged in the field of image generation, from early variational autoencoders (VAE), to generation From the adversarial network (GAN) to the recently popular diffusion model (Diffusion Model), the model generation capabilities have improved rapidly. Models represented by Stable Diffusion, Midjourney, etc. have achieved unprecedented results in generating highly realistic images. At the same time, in the field of video generation, a lot of excellent work has emerged recently. For example, Runway's generative model can generate imaginative video clips. These applications have greatly lowered the threshold for content creation, making it easy for everyone to turn their wild ideas into reality.
However, as the media carrying content become more and more abundant, people are gradually no longer satisfied with two-dimensional graphic content such as pictures, texts and videos. With the continuous development of interactive electronic game technology, especially the gradual maturity of applications such as virtual and augmented reality, people increasingly hope to interact with scenes and objects from a three-dimensional perspective, which brings about the need for three-dimensional content. Generate greater demands.
How to quickly generate high-quality three-dimensional content with fine geometric structure and highly realistic appearance has always been a key issue explored by researchers in the computer graphics community. The intelligent generation of three-dimensional content through computers can assist in the production of important digital assets in games, film and television production in actual production applications, greatly reducing the development time of art production personnel, significantly reducing asset acquisition costs, and shortening the overall production The cycle also provides technical possibilities for users to bring thousands of personalized visual experiences. For ordinary users, the emergence of fast and convenient 3D content creation tools, combined with applications such as desktop 3D printers, will bring more unlimited imagination to the entertainment life of ordinary consumers in the future.
Currently, although ordinary users can easily create two-dimensional content such as images and videos through devices such as portable cameras, and can even model and scan three-dimensional scenes, in general, high The creation of quality 3D content often requires manual modeling and rendering by experienced professionals using software such as 3ds Max, Maya, Blender, etc., but these have high learning costs and steep growth curves.
One of the main reasons is that the expression of three-dimensional content is very complex, such as geometric models, texture maps or character skeleton animations. Even in terms of geometric expression, it can be in various forms such as point clouds, voxels, and meshes. The complexity of three-dimensional expression greatly limits subsequent data acquisition and algorithm design.
On the other hand, 3D data is naturally scarce, and the cost of data acquisition is high. It often requires expensive equipment and complex acquisition processes, and it is difficult to collect a large number of 3D data in a unified format. data. This makes most data-driven deep generative models difficult to use.
At the algorithm level, how to feed the collected three-dimensional data into the calculation model is also a difficult problem to solve. The computing power overhead of three-dimensional data processing is exponentially higher than that of two-dimensional data. Violently extending the two-dimensional generation algorithm to three dimensions is difficult for even the most advanced parallel computing processors to process within an acceptable time.
For the above reasons, most of the current 3D content generation work is limited to a specific category or can only generate lower resolution content, making it difficult to apply it to real production processes.
In order to solve the above problems, Peking University Chen Baoquan’s team teamed up with researchers from Shandong University and Tencent AI Lab to propose the first single-sample scenario without training. A method that can generate a variety of high-quality 3D scenes. This algorithm has the following advantages:
1. It does not require large-scale similar training data and long-term training, and can quickly generate high-quality three-dimensional scenes using only a single sample;
2, using Plenoxels based on neural radiation fields as a three-dimensional expression, the scene has a highly realistic appearance and can render photo-realistic multi-view images. The generated scene also perfectly retains all the characteristics of the sample, such as the effect of the reflection on the water surface changing with the viewing angle;
3, supports a variety of application production scenarios, such as three-dimensional scenes Editing, size redirection, scene structure analogy, changing scene appearance, etc.
Method introduction
The researchers proposed a multi-scale progressive generation framework, as shown in the figure below. The core idea of the algorithm is to dismantle the sample scene into multiple blocks, introduce Gaussian noise, and then reassemble them into similar new scenes in a manner similar to building blocks.
The author uses the coordinate mapping field, an expression heterogeneous with the sample, to represent the generated scene, making high-quality generation feasible. In order to make the optimization process of the algorithm more robust, this study also proposes an optimization method based on a mixture of values and coordinates. At the same time, in order to solve the problem of massive resource consumption in three-dimensional calculations, this research uses an accurate to approximate optimization strategy, which enables the generation of high-quality new scenes in minutes without any training. Please refer to the original paper for more technical details.
Random scene generation
##With a single 3D sample scene like the one in the box on the left, new scenes with complex geometry and realistic appearance can be quickly generated. This method can handle objects with complex topology, such as cacti, arches, and stone benches, etc., and the generated scenes perfectly retain the fine geometry and high-quality appearance of the sample scenes. No current generative model based on neural networks achieves similar quality and diversity.
High resolution large scene generation
This method can efficiently generate extremely high resolution of 3D content. As shown above, we can generate a 1328 x 512 x 200 resolution "Thousand Miles of Rivers and Mountains" part by inputting a single part of the three-dimensional "Thousands of Miles of Rivers and Mountains" with a resolution of 512 x 512 x 200 in the upper left corner, and render it 4096 x 1024 2D multi-view images with high resolution.
Real world borderless scene generation
The author also verified the proposed generation method on real natural scenes. By adopting a processing method similar to NeRF, after explicitly separating the foreground and the background such as the sky, and generating the foreground content separately, new scenes can be generated in borderless scenes in the real world.
Other application scenarios
Scenario editing
Using the same generation algorithm framework, by adding artificially specified restrictions, editing operations such as deletion, copying and modification of objects in the three-dimensional scene can be performed. As shown in the picture, you can remove the mountain from the scene and automatically fill in the holes, duplicate it to create three peaks, or make the mountain larger.
Size Redirect
This method can also stretch or compress a three-dimensional object while maintaining its local shape. The green frame in the picture shows the original sample scene, which elongates a three-dimensional train while maintaining the local size of the window.
Structural analogy generation
#Similar to image style transfer, given two scenes A and B, we can create a new scene that has the appearance and geometric characteristics of A, but has a similar structure to B. For example, we can refer to a snow mountain to turn another mountain into a three-dimensional snow mountain.
Change the sample scene
Summary
This work is oriented to the field of three-dimensional content generation. It proposes a three-dimensional natural scene generation model based on a single sample for the first time, trying to solve the problems of current three-dimensional generation methods. There are problems such as large data requirements, high computing power overhead, and poor generation quality. This work focuses on more general natural scenes with weak semantic information, paying more attention to the diversity and quality of generated content. The algorithm is mainly inspired by technologies related to texture image generation in traditional computer graphics, and combined with the recent neural radiation field, it can quickly generate high-quality three-dimensional scenes and has demonstrated a variety of practical applications.Future Outlook
This work has strong versatility. It can not only be combined with current neural expressions, but also suitable for traditional rendering pipeline geometric expressions, such as polygon meshes. Mesh. While we focus on large data and models, we should also review traditional graphics tools from time to time. Researchers believe that in the near future, in the field of 3D AIGC, traditional graphics tools combined with high-quality neural expressions and powerful generation models will create more brilliant sparks, further promoting the quality and speed of 3D content generation, and liberating People's creativity.This research has been discussed by the majority of netizens:
Some netizens said: (This research) is very good for game development. , you only need to model a single model to generate many new versions.
The above is the detailed content of 3D scene generation: Generate diverse results from a single sample without any neural network training. For more information, please follow other related articles on the PHP Chinese website!

Hot AI Tools

Undresser.AI Undress
AI-powered app for creating realistic nude photos

AI Clothes Remover
Online AI tool for removing clothes from photos.

Undress AI Tool
Undress images for free

Clothoff.io
AI clothes remover

Video Face Swap
Swap faces in any video effortlessly with our completely free AI face swap tool!

Hot Article

Hot Tools

Notepad++7.3.1
Easy-to-use and free code editor

SublimeText3 Chinese version
Chinese version, very easy to use

Zend Studio 13.0.1
Powerful PHP integrated development environment

Dreamweaver CS6
Visual web development tools

SublimeText3 Mac version
God-level code editing software (SublimeText3)

Hot Topics










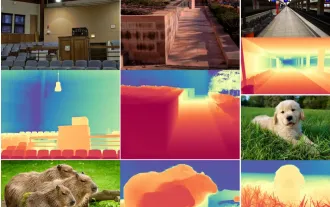
0.What does this article do? We propose DepthFM: a versatile and fast state-of-the-art generative monocular depth estimation model. In addition to traditional depth estimation tasks, DepthFM also demonstrates state-of-the-art capabilities in downstream tasks such as depth inpainting. DepthFM is efficient and can synthesize depth maps within a few inference steps. Let’s read about this work together ~ 1. Paper information title: DepthFM: FastMonocularDepthEstimationwithFlowMatching Author: MingGui, JohannesS.Fischer, UlrichPrestel, PingchuanMa, Dmytr
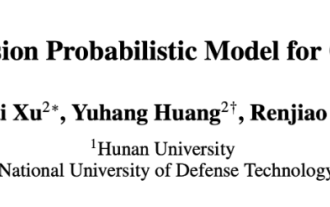
Current deep edge detection networks usually adopt an encoder-decoder architecture, which contains up and down sampling modules to better extract multi-level features. However, this structure limits the network to output accurate and detailed edge detection results. In response to this problem, a paper on AAAI2024 provides a new solution. Thesis title: DiffusionEdge:DiffusionProbabilisticModelforCrispEdgeDetection Authors: Ye Yunfan (National University of Defense Technology), Xu Kai (National University of Defense Technology), Huang Yuxing (National University of Defense Technology), Yi Renjiao (National University of Defense Technology), Cai Zhiping (National University of Defense Technology) Paper link: https ://ar
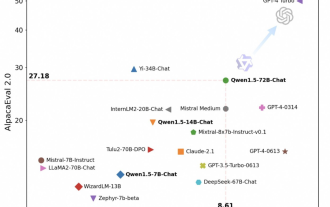
In time for the Spring Festival, version 1.5 of Tongyi Qianwen Model (Qwen) is online. This morning, the news of the new version attracted the attention of the AI community. The new version of the large model includes six model sizes: 0.5B, 1.8B, 4B, 7B, 14B and 72B. Among them, the performance of the strongest version surpasses GPT3.5 and Mistral-Medium. This version includes Base model and Chat model, and provides multi-language support. Alibaba’s Tongyi Qianwen team stated that the relevant technology has also been launched on the Tongyi Qianwen official website and Tongyi Qianwen App. In addition, today's release of Qwen 1.5 also has the following highlights: supports 32K context length; opens the checkpoint of the Base+Chat model;
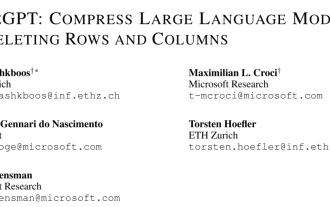
Large language models (LLMs) typically have billions of parameters and are trained on trillions of tokens. However, such models are very expensive to train and deploy. In order to reduce computational requirements, various model compression techniques are often used. These model compression techniques can generally be divided into four categories: distillation, tensor decomposition (including low-rank factorization), pruning, and quantization. Pruning methods have been around for some time, but many require recovery fine-tuning (RFT) after pruning to maintain performance, making the entire process costly and difficult to scale. Researchers from ETH Zurich and Microsoft have proposed a solution to this problem called SliceGPT. The core idea of this method is to reduce the embedding of the network by deleting rows and columns in the weight matrix.
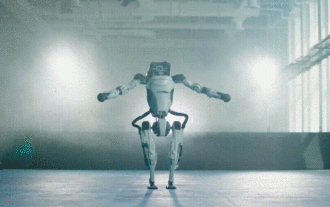
Boston Dynamics Atlas officially enters the era of electric robots! Yesterday, the hydraulic Atlas just "tearfully" withdrew from the stage of history. Today, Boston Dynamics announced that the electric Atlas is on the job. It seems that in the field of commercial humanoid robots, Boston Dynamics is determined to compete with Tesla. After the new video was released, it had already been viewed by more than one million people in just ten hours. The old people leave and new roles appear. This is a historical necessity. There is no doubt that this year is the explosive year of humanoid robots. Netizens commented: The advancement of robots has made this year's opening ceremony look like a human, and the degree of freedom is far greater than that of humans. But is this really not a horror movie? At the beginning of the video, Atlas is lying calmly on the ground, seemingly on his back. What follows is jaw-dropping
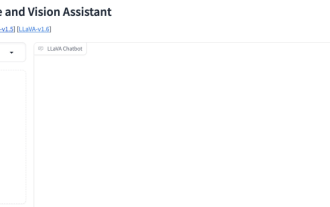
In April last year, researchers from the University of Wisconsin-Madison, Microsoft Research, and Columbia University jointly released LLaVA (Large Language and Vision Assistant). Although LLaVA is only trained with a small multi-modal instruction data set, it shows very similar inference results to GPT-4 on some samples. Then in October, they launched LLaVA-1.5, which refreshed the SOTA in 11 benchmarks with simple modifications to the original LLaVA. The results of this upgrade are very exciting, bringing new breakthroughs to the field of multi-modal AI assistants. The research team announced the launch of LLaVA-1.6 version, targeting reasoning, OCR and
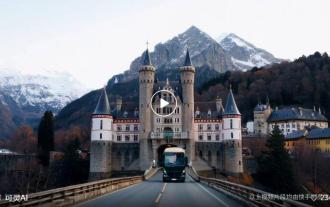
What? Is Zootopia brought into reality by domestic AI? Exposed together with the video is a new large-scale domestic video generation model called "Keling". Sora uses a similar technical route and combines a number of self-developed technological innovations to produce videos that not only have large and reasonable movements, but also simulate the characteristics of the physical world and have strong conceptual combination capabilities and imagination. According to the data, Keling supports the generation of ultra-long videos of up to 2 minutes at 30fps, with resolutions up to 1080p, and supports multiple aspect ratios. Another important point is that Keling is not a demo or video result demonstration released by the laboratory, but a product-level application launched by Kuaishou, a leading player in the short video field. Moreover, the main focus is to be pragmatic, not to write blank checks, and to go online as soon as it is released. The large model of Ke Ling is already available in Kuaiying.
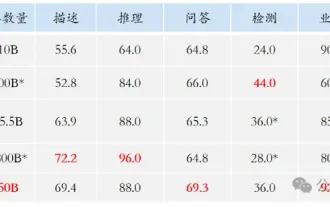
I cry to death. The world is madly building big models. The data on the Internet is not enough. It is not enough at all. The training model looks like "The Hunger Games", and AI researchers around the world are worrying about how to feed these data voracious eaters. This problem is particularly prominent in multi-modal tasks. At a time when nothing could be done, a start-up team from the Department of Renmin University of China used its own new model to become the first in China to make "model-generated data feed itself" a reality. Moreover, it is a two-pronged approach on the understanding side and the generation side. Both sides can generate high-quality, multi-modal new data and provide data feedback to the model itself. What is a model? Awaker 1.0, a large multi-modal model that just appeared on the Zhongguancun Forum. Who is the team? Sophon engine. Founded by Gao Yizhao, a doctoral student at Renmin University’s Hillhouse School of Artificial Intelligence.
