


Exploring future autonomous driving technology: 4D millimeter wave radar
The current realization of self-driving car perception is inseparable from sensing equipment such as lidar, on-board cameras, and millimeter-wave radar installed on the vehicle. By collecting data on the traffic environment around the vehicle, self-driving cars can surpass or even surpass humans. eyes to perceive the surrounding world and provide more accurate and rich environmental information for the decision-making and planning module, so that self-driving cars can drive safely.
The stacking of sensor hardware allows self-driving cars to drive more safely on the road, but on the other hand, a single hardware device cannot obtain all required data. , and in many extreme environments, hardware equipment will also have problems. For example, in extreme weather such as heavy fog and heavy rain, the measured data of lidar will have large deviations; millimeter-wave radar does not have the ability to measure altitude, and it is difficult to judge the stationary position ahead. Whether the object is on the ground or in the air; on-board cameras can only capture 2D plane images. Even with the assistance of deep learning, they still cannot accurately measure the distance between surrounding objects and self-driving cars. Therefore, self-driving cars require different hardware equipment. Working simultaneously, autonomous vehicles can achieve perception accuracy and capabilities that are no less than those of humans under any circumstances. The emergence of 4D millimeter wave radar will bring revolutionary changes to autonomous driving.
What is 4D millimeter wave radar
In autonomous driving perception, millimeter wave radar, as one of the most important sensors in autonomous driving, is a crucial one. ring. However, because it does not have the ability to measure height, it is difficult to determine whether the stationary object ahead is on the ground or in the air. When encountering ground or air objects such as manhole covers, speed bumps, overpasses, traffic signs, etc., it is impossible to accurately measure the height data of the object. . The emergence of 4D millimeter wave radar will make up for this problem. 4D millimeter wave radar is also called imaging radar. Based on the original distance, speed, and direction data, it adds a high degree of analysis of the target, and the fourth Dimensions are integrated into traditional millimeter wave radar to better understand and map the environment, making the measured traffic data more accurate.
As early as 2020, Tesla announced that it would add a 4D sensor technology to Tesla cars. Through 4D sensor technology, existing work The range is tripled for more traffic information. 4D millimeter wave radar can effectively analyze the contours, behaviors and categories of measured targets. It can adapt to more complex roads and identify more small objects, blocked objects and monitoring of stationary or horizontal objects, so that it can accurately understand the needs of vehicles. Under what circumstances should you brake? Compared with 3D millimeter wave radar, which can only measure three data: azimuth angle, elevation angle and speed, 4D millimeter wave radar can obtain more data, thus providing more reliable information for decision-making and planning.
Solution of 4D millimeter wave radar
4D millimeter wave radar was first proposed by an Israeli company in 2019. In early 2020, Waymo announced its fifth-generation autonomous driving 4D millimeter wave radar is launched in the perception suite. In the same year, Continental launched the first 4D millimeter wave radar mass production solution and stated that BMW would be the first car company to implement it. At 2021CES, 4D millimeter wave radar is also gaining momentum. Many manufacturers have unveiled their products. Texas Instruments, Mobileye and other companies have successively launched or updated 4D millimeter wave radar solutions.
Last year, Aptiv unveiled its next-generation L1~L3 autonomous driving platform, claiming that its sensor suite included 4D millimeter wave radar; ZF said it had obtained a production order for 4D millimeter wave radar from SAIC Group , will be officially supplied in 2022; Bosch launches the 5th generation radar extreme version, namely 4D millimeter wave radar, for the first time in the Chinese market.
4D millimeter wave radar, like traditional radar, will not experience great deviations when working under extreme weather conditions, and after increasing the elevation angle, it can form point cloud images, which means that 4D millimeter wave radar The radar can not only detect the distance, relative speed and azimuth of the object, but also detect the vertical height of the object in front as well as stationary and laterally moving objects in front, which will make up for the shortcomings of traditional radar in detecting static targets. At present, there are two main technical solutions for 4D millimeter wave radar:
- One is for 4D millimeter wave radar companies to independently develop multi-channel array radio frequency chipsets, radar processor chips and post-processing software algorithms based on artificial intelligence.
- The other is a solution based on traditional radar chip suppliers, which uses multi-chip extreme connection or software algorithms to achieve dense point cloud output and recognition.
The main feature of 4D millimeter wave radar is that the angular resolution is very high. The angular resolution of the front 4D millimeter wave radar can reach 1 degree in azimuth and 2 degrees in elevation. , when a self-driving car equipped with 4D millimeter wave radar detects road information, it can directly detect the outline of objects around the vehicle. For example, when road information is relatively rich, such as when pedestrians and vehicles are mixed together, 4D millimeter wave radar can directly identify pedestrians and vehicles, and can determine the movement of the corresponding objects (whether they are moving and the direction of movement).
4D millimeter wave radar can also detect geometric shapes, such as the length and width of tunnels in tunnel scenes. The emergence of 4D millimeter wave radar has made up for the performance shortcomings of traditional millimeter wave radar in a targeted manner. It not only increases the dimension of 3D, but also brings about comprehensive upgrades in detection accuracy, sensitivity, resolution and performance, giving autonomous driving more capabilities. High safety is expected to make millimeter wave radar one of the core sensors in autonomous driving systems.
The future development trend of 4D millimeter wave radar
According to analysis by industry insiders, the large-scale implementation of 4D millimeter wave radar is coming soon. In terms of marketization, the current technology is becoming mature, and there are many innovative algorithms in the process of productization. Many car manufacturers already have requirements for equipping new vehicles. Among them, automatic parking and L3 and above level autonomous driving require 4D imaging. In fact, since last year, many 4D millimeter wave radar products have been installed in vehicles for road testing. and prepare for mass production.
For example, NXP announced that the industry’s first dedicated 16nm millimeter wave radar processor S32R45 will be used for customer mass production for the first time starting in the first half of the year. Mobileye, a subsidiary of Intel, is also actively promoting the development and application of 4D millimeter wave radar. Mobileye CEO Amnon Shashua emphasized the application scenarios of 4D imaging millimeter wave radar in automobiles in this year's CES speech.
He said: "By 2025, except for the front of the car, we only want millimeter-wave radar, not lidar." In Mobileye's plan, millimeter-wave radar-based radar will be launched by 2025 /Lidar's consumer-grade autonomous vehicle solution, the car is equipped with a radar-LiDAR subsystem, and the vehicle only needs to be equipped with a forward-facing lidar and a 360-degree fully-covered millimeter-wave radar to achieve autonomous driving tasks.
Autonomous driving technology cannot rely on a single sensor to dominate the world and become an industry consensus. According to the current market understanding of autonomous driving, there is no one-size-fits-all sensor, because the market has many segments and different levels of autonomous driving. In the end, it is very likely that cameras and radars will coexist because their advantages and disadvantages are very complementary. What is special is lidar. The author believes that there is a great possibility that 4D millimeter wave radar solutions can reduce or replace the use of lidar. 4D millimeter wave radar is still in the early stages of development, but the author believes that its performance will be greatly improved in the future, and under ideal circumstances, it can eventually replace lidar. ”
The above is the detailed content of Exploring future autonomous driving technology: 4D millimeter wave radar. For more information, please follow other related articles on the PHP Chinese website!

Hot AI Tools

Undresser.AI Undress
AI-powered app for creating realistic nude photos

AI Clothes Remover
Online AI tool for removing clothes from photos.

Undress AI Tool
Undress images for free

Clothoff.io
AI clothes remover

Video Face Swap
Swap faces in any video effortlessly with our completely free AI face swap tool!

Hot Article

Hot Tools

Notepad++7.3.1
Easy-to-use and free code editor

SublimeText3 Chinese version
Chinese version, very easy to use

Zend Studio 13.0.1
Powerful PHP integrated development environment

Dreamweaver CS6
Visual web development tools

SublimeText3 Mac version
God-level code editing software (SublimeText3)

Hot Topics
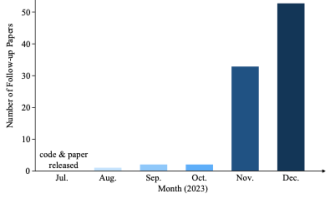
Written above & the author’s personal understanding Three-dimensional Gaussiansplatting (3DGS) is a transformative technology that has emerged in the fields of explicit radiation fields and computer graphics in recent years. This innovative method is characterized by the use of millions of 3D Gaussians, which is very different from the neural radiation field (NeRF) method, which mainly uses an implicit coordinate-based model to map spatial coordinates to pixel values. With its explicit scene representation and differentiable rendering algorithms, 3DGS not only guarantees real-time rendering capabilities, but also introduces an unprecedented level of control and scene editing. This positions 3DGS as a potential game-changer for next-generation 3D reconstruction and representation. To this end, we provide a systematic overview of the latest developments and concerns in the field of 3DGS for the first time.
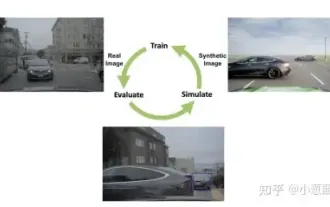
Yesterday during the interview, I was asked whether I had done any long-tail related questions, so I thought I would give a brief summary. The long-tail problem of autonomous driving refers to edge cases in autonomous vehicles, that is, possible scenarios with a low probability of occurrence. The perceived long-tail problem is one of the main reasons currently limiting the operational design domain of single-vehicle intelligent autonomous vehicles. The underlying architecture and most technical issues of autonomous driving have been solved, and the remaining 5% of long-tail problems have gradually become the key to restricting the development of autonomous driving. These problems include a variety of fragmented scenarios, extreme situations, and unpredictable human behavior. The "long tail" of edge scenarios in autonomous driving refers to edge cases in autonomous vehicles (AVs). Edge cases are possible scenarios with a low probability of occurrence. these rare events
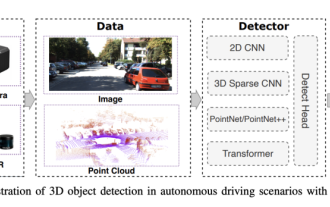
0.Written in front&& Personal understanding that autonomous driving systems rely on advanced perception, decision-making and control technologies, by using various sensors (such as cameras, lidar, radar, etc.) to perceive the surrounding environment, and using algorithms and models for real-time analysis and decision-making. This enables vehicles to recognize road signs, detect and track other vehicles, predict pedestrian behavior, etc., thereby safely operating and adapting to complex traffic environments. This technology is currently attracting widespread attention and is considered an important development area in the future of transportation. one. But what makes autonomous driving difficult is figuring out how to make the car understand what's going on around it. This requires that the three-dimensional object detection algorithm in the autonomous driving system can accurately perceive and describe objects in the surrounding environment, including their locations,
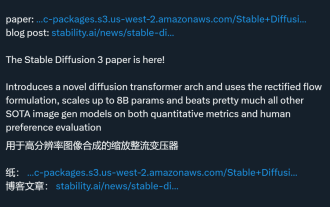
StableDiffusion3’s paper is finally here! This model was released two weeks ago and uses the same DiT (DiffusionTransformer) architecture as Sora. It caused quite a stir once it was released. Compared with the previous version, the quality of the images generated by StableDiffusion3 has been significantly improved. It now supports multi-theme prompts, and the text writing effect has also been improved, and garbled characters no longer appear. StabilityAI pointed out that StableDiffusion3 is a series of models with parameter sizes ranging from 800M to 8B. This parameter range means that the model can be run directly on many portable devices, significantly reducing the use of AI
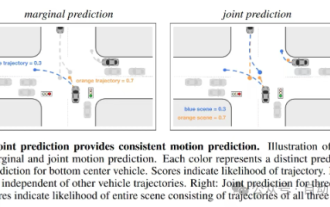
Trajectory prediction plays an important role in autonomous driving. Autonomous driving trajectory prediction refers to predicting the future driving trajectory of the vehicle by analyzing various data during the vehicle's driving process. As the core module of autonomous driving, the quality of trajectory prediction is crucial to downstream planning control. The trajectory prediction task has a rich technology stack and requires familiarity with autonomous driving dynamic/static perception, high-precision maps, lane lines, neural network architecture (CNN&GNN&Transformer) skills, etc. It is very difficult to get started! Many fans hope to get started with trajectory prediction as soon as possible and avoid pitfalls. Today I will take stock of some common problems and introductory learning methods for trajectory prediction! Introductory related knowledge 1. Are the preview papers in order? A: Look at the survey first, p

Original title: SIMPL: ASimpleandEfficientMulti-agentMotionPredictionBaselineforAutonomousDriving Paper link: https://arxiv.org/pdf/2402.02519.pdf Code link: https://github.com/HKUST-Aerial-Robotics/SIMPL Author unit: Hong Kong University of Science and Technology DJI Paper idea: This paper proposes a simple and efficient motion prediction baseline (SIMPL) for autonomous vehicles. Compared with traditional agent-cent
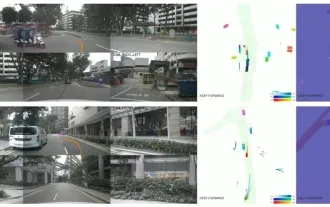
In the past month, due to some well-known reasons, I have had very intensive exchanges with various teachers and classmates in the industry. An inevitable topic in the exchange is naturally end-to-end and the popular Tesla FSDV12. I would like to take this opportunity to sort out some of my thoughts and opinions at this moment for your reference and discussion. How to define an end-to-end autonomous driving system, and what problems should be expected to be solved end-to-end? According to the most traditional definition, an end-to-end system refers to a system that inputs raw information from sensors and directly outputs variables of concern to the task. For example, in image recognition, CNN can be called end-to-end compared to the traditional feature extractor + classifier method. In autonomous driving tasks, input data from various sensors (camera/LiDAR
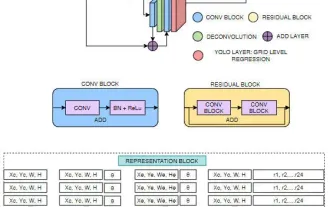
Target detection is a relatively mature problem in autonomous driving systems, among which pedestrian detection is one of the earliest algorithms to be deployed. Very comprehensive research has been carried out in most papers. However, distance perception using fisheye cameras for surround view is relatively less studied. Due to large radial distortion, standard bounding box representation is difficult to implement in fisheye cameras. To alleviate the above description, we explore extended bounding box, ellipse, and general polygon designs into polar/angular representations and define an instance segmentation mIOU metric to analyze these representations. The proposed model fisheyeDetNet with polygonal shape outperforms other models and simultaneously achieves 49.5% mAP on the Valeo fisheye camera dataset for autonomous driving
