


Released Chinese paragraph sorting benchmark data set: based on 300,000 real queries and 2 million Internet paragraphs.
Paragraph sorting is a very important and challenging topic in the field of information retrieval, and has received widespread attention from academia and industry. The effectiveness of the paragraph ranking model can improve search engine user satisfaction and help information retrieval-related applications such as question and answer systems, reading comprehension, etc. In this context, some benchmark datasets such as MS-MARCO, DuReader_retrieval, etc. were constructed to support related research work on paragraph sorting. However, most of the commonly used data sets focus on English scenes. For Chinese scenes, existing data sets have limitations in data scale, fine-grained user annotation, and solution to the problem of false negative examples. In this context, we constructed a new Chinese paragraph ranking benchmark data set based on real search logs: T2Ranking.
##T2Ranking consists of more than 300,000 real queries and 2 million Internet paragraphs, and includes information provided by professional annotators Level 4 fine-grained correlation annotation. The current data and some baseline models have been published on Github, and the relevant research work has been accepted by SIGIR 2023 as a Resource paper.
- Paper information: Xiaohui Xie, Qian Dong, Bingning Wang, Feiyang Lv , Ting Yao, Weinan Gan, Zhijing Wu, Xiangsheng Li, Haitao Li, Yiqun Liu, and Jin Ma. T2Ranking: A large-scale Chinese Benchmark . SIGIR 2023.
- ##Paper address: https://arxiv.org/abs/2304.03679
- Github address: https://github.com/THUIR/T2Ranking Background and Related Work
To support the paragraph sorting task, multiple data sets are constructed for training and testing paragraph sorting algorithms. Most of the widely used datasets focus on English scenes. For example, the most commonly used dataset is the MS-MARCO dataset, which contains more than 500,000 query terms and more than 8 million paragraphs. Each query term has a question attribute. For each query term, the MS-MARCO data release team recruited annotators to provide standard answers. Based on whether a given paragraph contains the manually provided standard answers, it is judged whether this paragraph is related to the query term.
In the Chinese scenario, there are also some data sets built to support paragraph sorting tasks. For example, mMarco-Chinese is the Chinese translation version of the MS-MARCO data set, and the DuReader_retrieval data set uses the same paradigm as MS-MARCO to generate paragraph labels, that is, the correlation of the query word-paragraph pair is given from the standard answers provided by humans. score. The Multi-CPR model contains paragraph retrieval data from three different domains (e-commerce, entertainment videos, and medicine). Based on the log data of Sogou search, data sets such as Sogou-SRR, Sogou-QCL and Tiangong-PDR have also been proposed.
Figure 1: Statistics of commonly used data sets in paragraph sorting tasks
Although existing data sets have promoted the development of paragraph sorting applications, we also need to pay attention to several limitations:1) These data sets are not large-scale The or relevance labels are not manually annotated, especially in the Chinese scenario. Sogou-SRR and Tiangong-PDR only contain a small amount of query data. Although mMarco-Chinese and Sogou-QCL are larger in scale, the former is based on machine translation, and the latter uses relevance labels as user click data. Recently, two relatively large datasets, Multi-CPR and DuReader_retrieval, have been constructed and released.
2) Existing data sets lack fine-grained correlation annotation information. Most data sets use binary correlation annotation (coarse-grained), that is, relevant or irrelevant. Existing work has shown that fine-grained correlation annotation information can help mine the relationships between different entities and build more accurate ranking algorithms. Then there are existing datasets that do not provide or only provide a small amount of multi-level fine-grained annotations. For example, Sogou-SRR or Tiangong-PDR only provide fine-grained annotations no more than 100,000. 3) The problem of false negative examples affects the accuracy of the evaluation. Existing datasets are affected by the false negative example problem, where a large number of relevant documents are marked as irrelevant. This problem is caused by the small number of manual annotations in large-scale data, which will significantly affect the accuracy of the evaluation. For example, in Multi-CPR, only one paragraph will be marked as relevant for each query term, while the others will be marked as irrelevant. DuReader_retrieval attempts to alleviate the false negative problem by letting the annotator manually inspect and re-annotate the top paragraph set. In order to better support paragraph ranking models for high-quality training and evaluation, we constructed and released a new Chinese paragraph retrieval benchmark data set - T2Ranking. The data set construction process includes query word sampling, document recall, paragraph extraction and fine-grained correlation annotation. At the same time, we have also designed multiple methods to improve the quality of the data set, including using model-based paragraph segmentation methods and clustering-based paragraph deduplication methods to ensure the semantic integrity and diversity of paragraphs, and using active learning-based annotation. Methods to improve the efficiency and quality of annotation, etc. 1) Overall process Figure 2: Example of a Wikipedia page. The document presented contains clearly defined paragraphs. 2) Model-based paragraph segmentation method In existing data sets, Paragraphs are usually split from the document based on natural paragraphs (line breaks) or by fixed-length sliding windows. However, both methods may result in paragraphs that are semantically incomplete or that are too long and contain multiple different topics. In this work, we adopted a model-based paragraph segmentation method. Specifically, we used Sogou Encyclopedia, Baidu Encyclopedia and Chinese Wikipedia as training data, because the structure of this part of the document is relatively clear, and the natural paragraphs are also got a better definition. We trained a segmentation model to determine whether a given word needs to be a segmentation point. We used the idea of sequence labeling tasks and used the last word of each natural segment as a positive example to train the model. 3) Clustering-based paragraph deduplication method It is redundant to annotate highly similar paragraphs and meaningless. For the paragraph ranking model, the information gain brought by highly similar paragraph content is limited, so we designed a clustering-based paragraph deduplication method to improve the efficiency of annotation. Specifically, we employ Ward, a hierarchical clustering algorithm, to perform unsupervised clustering of similar documents. Paragraphs in the same class are considered to be highly similar, and we sample one paragraph from each class for relevance annotation. It should be noted that we only perform this operation on the training set. For the test set, we will fully annotate all extracted paragraphs to reduce the impact of false negative examples. Figure 3: Sampling annotation process based on active learning 4) Data sampling annotation method based on active learning In practice, we have observed that not all training samples can be further improved Performance of ranking models. For training samples that the model can accurately predict, the training help for subsequent models is limited. Therefore, we borrowed the idea of active learning to enable the model to select more informative training samples for further annotation. Specifically, we first trained a query word-paragraph reordering model based on the cross-encoder framework based on the existing training data. Then we used this model to predict other data and remove excessive confidence scores (information content). (low) and too low confidence score (noisy data), further annotate the retained paragraphs, and iterate this process. T2Ranking consists of over 300,000 real queries and 2 million Internet paragraphs. Among them, the training set contains about 250,000 query words, and the test set contains about 50,000 query words. Query terms can be up to 40 characters long, with an average length of around 11 characters. At the same time, the query words in the data set cover multiple fields, including medicine, education, e-commerce, etc. We also calculated the diversity score (ILS) of the query words. Compared with existing data sets, our query diversity is more high. More than 2.3 million paragraphs were sampled from 1.75 million documents, and each document was divided into 1.3 paragraphs on average. In the training set, an average of 6.25 paragraphs per query term were manually annotated, while in the test set, an average of 15.75 paragraphs per query term were manually annotated. Figure 4: Domain distribution of query words in the data set Figure 5: Distribution of correlation annotations We tested the performance of some commonly used paragraph ranking models on the obtained data set. We also evaluated the existing methods in paragraph recall and paragraph emphasis. Performance on both stages of sorting. 1) Paragraph recall experiment Existing paragraph recall models can be roughly divided into sparse recall models and dense recall models. We tested the performance of the following recall models: Among these models, QL and BM25 are sparse recall models, and the other models are dense recall models. We use common indicators such as MRR and Recall to evaluate the performance of these models. The experimental results are shown in the following table: Figure 6: Paragraph Performance of the recall model on the test set It can be seen from the experimental results that the dense retrieval model achieves better performance than the traditional sparse sorting model. At the same time, the introduction of hard-to-negative examples is also helpful to improve the performance of the model. It is worth mentioning that the recall performance of these experimental models on our dataset is worse than that on other datasets. For example, the Recall@50 of BM25 on our dataset is 0.492, while in MS-Marco and Dureader_retrieval Above are 0.601 and 0.700. This may be due to the fact that we have more paragraphs that have been manually annotated. In the test set, we have an average of 4.74 relevant documents per query term, which makes the recall task more challenging and reduces false negatives to a certain extent. The problem. This also shows that T2Ranking is a challenging benchmark data set and has large room for improvement for future recall models. 2) Paragraph reordering experiment ##Compared with the paragraph recall stage, the reordering stage needs to be considered The paragraph size is small, so most methods tend to use an interactive encoder (Cross-Encoder) as the model framework. In this work, we test the performance of the interactive encoder model on the paragraph reordering task. We adopt MRR and nDCG As an evaluation index, the experimental results are as follows: Dataset construction process
Dataset Statistics
Experimental results of commonly used models
## Figure 7: Interactive encoder on paragraph reordering task The performance of It can achieve better results, which is consistent with the experimental conclusions of existing work. Similar to the recall experiment, the performance of the reranking model on our dataset is worse than that on other datasets, which may be due to the fine-grained annotation and higher query word diversity of our dataset, and further It illustrates that our data set is challenging and can more accurately reflect model performance. This data set was jointly released by the Information Retrieval Research Group (THUIR) of the Department of Computer Science of Tsinghua University and the QQ Browser Search Technology Center team of Tencent. It was approved by Tsinghua University Tian Supported by the Institute of Artificial Intelligence Computing. The THUIR research group focuses on research on search and recommendation methods, and has achieved typical results in user behavior modeling and explainable learning methods. The research group's results have won the WSDM2022 Best Paper Award, SIGIR2020 Best Paper Nomination Award and CIKM2018 Best Paper Award. He has won a number of academic awards including the 2020 Chinese Information Society "Qian Weichang Chinese Information Processing Science and Technology Award" first prize. The QQ Browser Search Technology Center team is the team responsible for search technology research and development of Tencent PCG information platform and service line. Relying on Tencent's content ecosystem and driving product innovation through user research, it provides users with graphics, information, novels, long and short videos, services, etc. The orientation information needs are met. Introduction to the data set publishing team
The above is the detailed content of Released Chinese paragraph sorting benchmark data set: based on 300,000 real queries and 2 million Internet paragraphs.. For more information, please follow other related articles on the PHP Chinese website!

Hot AI Tools

Undresser.AI Undress
AI-powered app for creating realistic nude photos

AI Clothes Remover
Online AI tool for removing clothes from photos.

Undress AI Tool
Undress images for free

Clothoff.io
AI clothes remover

Video Face Swap
Swap faces in any video effortlessly with our completely free AI face swap tool!

Hot Article

Hot Tools

Notepad++7.3.1
Easy-to-use and free code editor

SublimeText3 Chinese version
Chinese version, very easy to use

Zend Studio 13.0.1
Powerful PHP integrated development environment

Dreamweaver CS6
Visual web development tools

SublimeText3 Mac version
God-level code editing software (SublimeText3)

Hot Topics
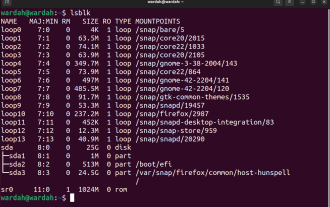
DDREASE is a tool for recovering data from file or block devices such as hard drives, SSDs, RAM disks, CDs, DVDs and USB storage devices. It copies data from one block device to another, leaving corrupted data blocks behind and moving only good data blocks. ddreasue is a powerful recovery tool that is fully automated as it does not require any interference during recovery operations. Additionally, thanks to the ddasue map file, it can be stopped and resumed at any time. Other key features of DDREASE are as follows: It does not overwrite recovered data but fills the gaps in case of iterative recovery. However, it can be truncated if the tool is instructed to do so explicitly. Recover data from multiple files or blocks to a single
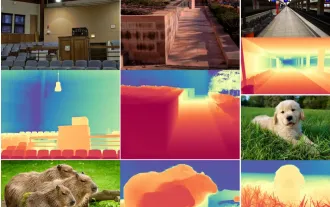
0.What does this article do? We propose DepthFM: a versatile and fast state-of-the-art generative monocular depth estimation model. In addition to traditional depth estimation tasks, DepthFM also demonstrates state-of-the-art capabilities in downstream tasks such as depth inpainting. DepthFM is efficient and can synthesize depth maps within a few inference steps. Let’s read about this work together ~ 1. Paper information title: DepthFM: FastMonocularDepthEstimationwithFlowMatching Author: MingGui, JohannesS.Fischer, UlrichPrestel, PingchuanMa, Dmytr
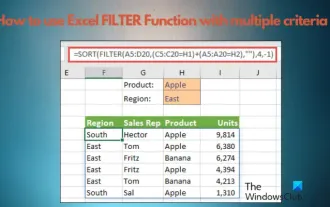
If you need to know how to use filtering with multiple criteria in Excel, the following tutorial will guide you through the steps to ensure you can filter and sort your data effectively. Excel's filtering function is very powerful and can help you extract the information you need from large amounts of data. This function can filter data according to the conditions you set and display only the parts that meet the conditions, making data management more efficient. By using the filter function, you can quickly find target data, saving time in finding and organizing data. This function can not only be applied to simple data lists, but can also be filtered based on multiple conditions to help you locate the information you need more accurately. Overall, Excel’s filtering function is a very practical
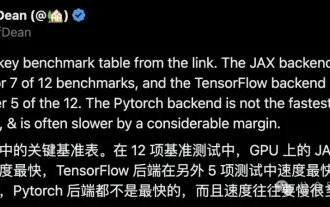
The performance of JAX, promoted by Google, has surpassed that of Pytorch and TensorFlow in recent benchmark tests, ranking first in 7 indicators. And the test was not done on the TPU with the best JAX performance. Although among developers, Pytorch is still more popular than Tensorflow. But in the future, perhaps more large models will be trained and run based on the JAX platform. Models Recently, the Keras team benchmarked three backends (TensorFlow, JAX, PyTorch) with the native PyTorch implementation and Keras2 with TensorFlow. First, they select a set of mainstream
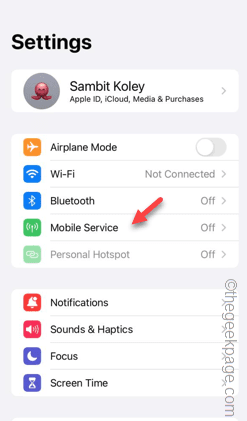
Facing lag, slow mobile data connection on iPhone? Typically, the strength of cellular internet on your phone depends on several factors such as region, cellular network type, roaming type, etc. There are some things you can do to get a faster, more reliable cellular Internet connection. Fix 1 – Force Restart iPhone Sometimes, force restarting your device just resets a lot of things, including the cellular connection. Step 1 – Just press the volume up key once and release. Next, press the Volume Down key and release it again. Step 2 – The next part of the process is to hold the button on the right side. Let the iPhone finish restarting. Enable cellular data and check network speed. Check again Fix 2 – Change data mode While 5G offers better network speeds, it works better when the signal is weaker
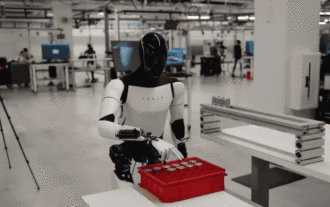
The latest video of Tesla's robot Optimus is released, and it can already work in the factory. At normal speed, it sorts batteries (Tesla's 4680 batteries) like this: The official also released what it looks like at 20x speed - on a small "workstation", picking and picking and picking: This time it is released One of the highlights of the video is that Optimus completes this work in the factory, completely autonomously, without human intervention throughout the process. And from the perspective of Optimus, it can also pick up and place the crooked battery, focusing on automatic error correction: Regarding Optimus's hand, NVIDIA scientist Jim Fan gave a high evaluation: Optimus's hand is the world's five-fingered robot. One of the most dexterous. Its hands are not only tactile
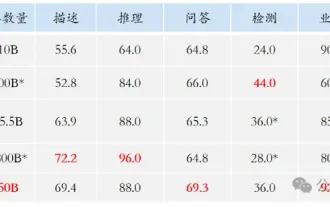
I cry to death. The world is madly building big models. The data on the Internet is not enough. It is not enough at all. The training model looks like "The Hunger Games", and AI researchers around the world are worrying about how to feed these data voracious eaters. This problem is particularly prominent in multi-modal tasks. At a time when nothing could be done, a start-up team from the Department of Renmin University of China used its own new model to become the first in China to make "model-generated data feed itself" a reality. Moreover, it is a two-pronged approach on the understanding side and the generation side. Both sides can generate high-quality, multi-modal new data and provide data feedback to the model itself. What is a model? Awaker 1.0, a large multi-modal model that just appeared on the Zhongguancun Forum. Who is the team? Sophon engine. Founded by Gao Yizhao, a doctoral student at Renmin University’s Hillhouse School of Artificial Intelligence.
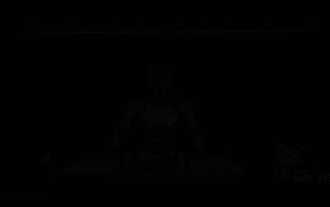
This week, FigureAI, a robotics company invested by OpenAI, Microsoft, Bezos, and Nvidia, announced that it has received nearly $700 million in financing and plans to develop a humanoid robot that can walk independently within the next year. And Tesla’s Optimus Prime has repeatedly received good news. No one doubts that this year will be the year when humanoid robots explode. SanctuaryAI, a Canadian-based robotics company, recently released a new humanoid robot, Phoenix. Officials claim that it can complete many tasks autonomously at the same speed as humans. Pheonix, the world's first robot that can autonomously complete tasks at human speeds, can gently grab, move and elegantly place each object to its left and right sides. It can autonomously identify objects
