Is python artificial intelligence difficult?
Artificial intelligence is an interdisciplinary subject with a history of more than 60 years since it was proposed. It is still in the initial stage of AI. An important reason for the slow development is that artificial intelligence is technically difficult, involving computers, psychology, philosophy, etc., and has high requirements for practitioners. Currently, many domestic engineers engaged in the AI industry have a master's degree or above.
Artificial intelligence technology can be applied to various industries such as security, medical care, home furnishing, transportation, smart cities, etc. Its prospects are beyond doubt, and the future is definitely a Trillion-level market. (Recommended learning: Python video tutorial)
According to different application fields, the technologies of artificial intelligence research are also different. Currently, machine learning, computer vision, etc. have become popular AI technology directions. , explore the development and future of artificial intelligence together.
Machine learning is the core of artificial intelligence
Machine learning is also called the core of artificial intelligence. It mainly studies how computers can simulate or realize human learning behavior. Acquire new knowledge or skills and help the computer reorganize the existing knowledge structure to continuously improve its performance.
Machine learning is a branch of artificial intelligence research, and people have been studying machine learning for many years. Its development process can be roughly divided into several periods. The first is from the mid-1950s to the mid-1960s, which is an enthusiastic period; the second is from the mid-1960s to the mid-1970s, which is called machine learning. The calming period; the third is from the mid-1970s to the mid-1980s, called the renaissance period; the fourth stage of machine learning began in 1986, and we are still in this period.
Machine learning can now be seen in many application fields, such as data mining, natural language processing, biometric identification, search engines, medical diagnosis, securities, games, robots, etc.
Learning is a very complex process. Learning and reasoning are inseparable. According to the amount of reasoning used in learning, the strategies used in machine learning can be divided into four types: mechanical learning, teaching learning, and analogy learning. and learning by example. The more reasoning used in learning, the stronger the system's capabilities.
What is the difficulty of machine learning?
For machine learning developers, in addition to being very proficient in mathematical knowledge, the tools they choose are also very important. On the one hand, machine learning research requires innovation, experimentation and persistence, and many people give up halfway; on the other hand, it is also difficult to apply machine learning models to actual work.
In addition to engineering factors, machine learning system design is also difficult. The most important factor affecting the design of the learning system is the information provided by the environment to the system. The quality of the information directly affects the performance of the system. The knowledge base stores general principles that guide the execution of some actions, but the information provided by the environment to the learning system is various. All kinds.
If the quality of the information is high and the differences from general principles are relatively small, machine learning is easier to process. If irregular instruction information is provided to the learning system, the learning system needs to obtain enough data, delete unnecessary details, summarize it, form guidance actions, and put it into the knowledge base; in this way, the task of machine learning will be relatively heavy. , it is also more difficult to design.
For machine learning, another technical difficulty is that the debugging of machine learning is very complicated. For example, when doing conventional software design, if the written problem does not work as expected, there may be problems with the algorithm and implementation; but In machine learning, the actual model and data are two key factors. The randomness of these two is very strong, which doubles the difficulty of debugging. In addition to complexity, the debugging cycle of machine learning is generally very long, because it usually takes more than ten hours or even days for the machine to receive instructions to implement corrections and changes.
For more Python related technical articles, please visit the Python Tutorial column to learn!
The above is the detailed content of Is python artificial intelligence difficult?. For more information, please follow other related articles on the PHP Chinese website!

Hot AI Tools

Undresser.AI Undress
AI-powered app for creating realistic nude photos

AI Clothes Remover
Online AI tool for removing clothes from photos.

Undress AI Tool
Undress images for free

Clothoff.io
AI clothes remover

Video Face Swap
Swap faces in any video effortlessly with our completely free AI face swap tool!

Hot Article

Hot Tools

Notepad++7.3.1
Easy-to-use and free code editor

SublimeText3 Chinese version
Chinese version, very easy to use

Zend Studio 13.0.1
Powerful PHP integrated development environment

Dreamweaver CS6
Visual web development tools

SublimeText3 Mac version
God-level code editing software (SublimeText3)

Hot Topics
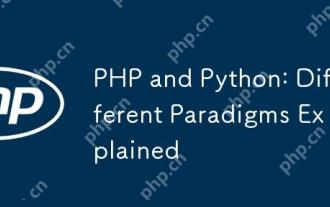
PHP is mainly procedural programming, but also supports object-oriented programming (OOP); Python supports a variety of paradigms, including OOP, functional and procedural programming. PHP is suitable for web development, and Python is suitable for a variety of applications such as data analysis and machine learning.
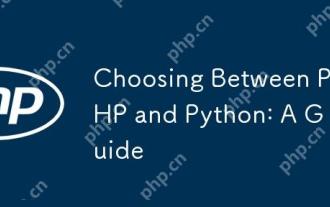
PHP is suitable for web development and rapid prototyping, and Python is suitable for data science and machine learning. 1.PHP is used for dynamic web development, with simple syntax and suitable for rapid development. 2. Python has concise syntax, is suitable for multiple fields, and has a strong library ecosystem.
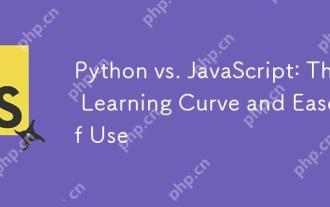
Python is more suitable for beginners, with a smooth learning curve and concise syntax; JavaScript is suitable for front-end development, with a steep learning curve and flexible syntax. 1. Python syntax is intuitive and suitable for data science and back-end development. 2. JavaScript is flexible and widely used in front-end and server-side programming.
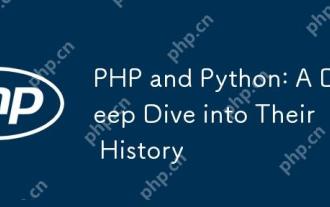
PHP originated in 1994 and was developed by RasmusLerdorf. It was originally used to track website visitors and gradually evolved into a server-side scripting language and was widely used in web development. Python was developed by Guidovan Rossum in the late 1980s and was first released in 1991. It emphasizes code readability and simplicity, and is suitable for scientific computing, data analysis and other fields.
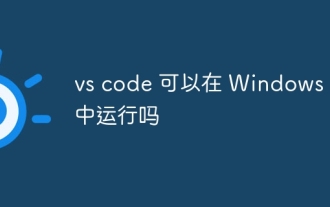
VS Code can run on Windows 8, but the experience may not be great. First make sure the system has been updated to the latest patch, then download the VS Code installation package that matches the system architecture and install it as prompted. After installation, be aware that some extensions may be incompatible with Windows 8 and need to look for alternative extensions or use newer Windows systems in a virtual machine. Install the necessary extensions to check whether they work properly. Although VS Code is feasible on Windows 8, it is recommended to upgrade to a newer Windows system for a better development experience and security.
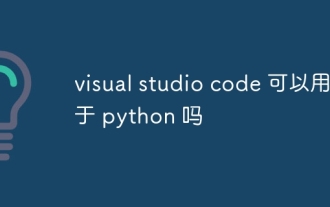
VS Code can be used to write Python and provides many features that make it an ideal tool for developing Python applications. It allows users to: install Python extensions to get functions such as code completion, syntax highlighting, and debugging. Use the debugger to track code step by step, find and fix errors. Integrate Git for version control. Use code formatting tools to maintain code consistency. Use the Linting tool to spot potential problems ahead of time.
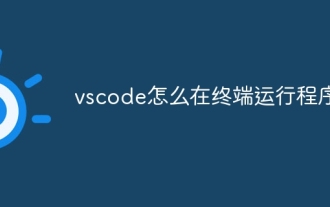
In VS Code, you can run the program in the terminal through the following steps: Prepare the code and open the integrated terminal to ensure that the code directory is consistent with the terminal working directory. Select the run command according to the programming language (such as Python's python your_file_name.py) to check whether it runs successfully and resolve errors. Use the debugger to improve debugging efficiency.
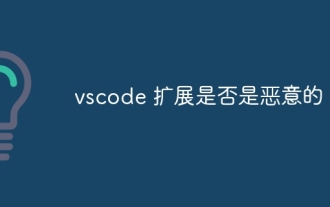
VS Code extensions pose malicious risks, such as hiding malicious code, exploiting vulnerabilities, and masturbating as legitimate extensions. Methods to identify malicious extensions include: checking publishers, reading comments, checking code, and installing with caution. Security measures also include: security awareness, good habits, regular updates and antivirus software.
