


Introduction to the method of reading custom data for Tensorflow classifier project (code example)
This article brings you an introduction to the method of reading custom data for the Tensorflow classifier project (code example). It has certain reference value. Friends in need can refer to it. , hope it helps you.
Tensorflow classifier project custom data reading
After typing the code of the classifier project according to the demo on the Tensorflow official website, the operation was successful. The result Not bad. But in the end, I still have to train my own data, so I tried to prepare to load custom data. However, fashion_mnist.load_data() only appeared in the demo without a detailed reading process. Then I found some information and explained the reading process. Recorded here.
First mention the modules you need to use:
import os import keras import matplotlib.pyplot as plt from PIL import Image from keras.preprocessing.image import ImageDataGenerator from sklearn.model_selection import train_test_split
Image classifier project, first determine what the resolution of the image you want to process will be, the example here is 30 pixels:
IMG_SIZE_X = 30 IMG_SIZE_Y = 30
Secondly determine the directory of your pictures:
image_path = r'D:\Projects\ImageClassifier\data\set' path = ".\data" # 你也可以使用相对路径的方式 # image_path =os.path.join(path, "set")
The structure under the directory is as follows:
The corresponding label.txt is as follows:
动漫 风景 美女 物语 樱花
Next is connected to labels.txt, as follows:
label_name = "labels.txt" label_path = os.path.join(path, label_name) class_names = np.loadtxt(label_path, type(""))
For the sake of simplicity, numpy's loadtxt function is directly used to load directly.
After that, the image data is officially processed, and the comments are written inside:
re_load = False re_build = False # re_load = True re_build = True data_name = "data.npz" data_path = os.path.join(path, data_name) model_name = "model.h5" model_path = os.path.join(path, model_name) count = 0 # 这里判断是否存在序列化之后的数据,re_load是一个开关,是否强制重新处理,测试用,可以去除。 if not os.path.exists(data_path) or re_load: labels = [] images = [] print('Handle images') # 由于label.txt是和图片防止目录的分类目录一一对应的,即每个子目录的目录名就是labels.txt里的一个label,所以这里可以通过读取class_names的每一项去拼接path后读取 for index, name in enumerate(class_names): # 这里是拼接后的子目录path classpath = os.path.join(image_path, name) # 先判断一下是否是目录 if not os.path.isdir(classpath): continue # limit是测试时候用的这里可以去除 limit = 0 for image_name in os.listdir(classpath): if limit >= max_size: break # 这里是拼接后的待处理的图片path imagepath = os.path.join(classpath, image_name) count = count + 1 limit = limit + 1 # 利用Image打开图片 img = Image.open(imagepath) # 缩放到你最初确定要处理的图片分辨率大小 img = img.resize((IMG_SIZE_X, IMG_SIZE_Y)) # 转为灰度图片,这里彩色通道会干扰结果,并且会加大计算量 img = img.convert("L") # 转为numpy数组 img = np.array(img) # 由(30,30)转为(1,30,30)(即`channels_first`),当然你也可以转换为(30,30,1)(即`channels_last`)但为了之后预览处理后的图片方便这里采用了(1,30,30)的格式存放 img = np.reshape(img, (1, IMG_SIZE_X, IMG_SIZE_Y)) # 这里利用循环生成labels数据,其中存放的实际是class_names中对应元素的索引 labels.append([index]) # 添加到images中,最后统一处理 images.append(img) # 循环中一些状态的输出,可以去除 print("{} class: {} {} limit: {} {}" .format(count, index + 1, class_names[index], limit, imagepath)) # 最后一次性将images和labels都转换成numpy数组 npy_data = np.array(images) npy_labels = np.array(labels) # 处理数据只需要一次,所以我们选择在这里利用numpy自带的方法将处理之后的数据序列化存储 np.savez(data_path, x=npy_data, y=npy_labels) print("Save images by npz") else: # 如果存在序列化号的数据,便直接读取,提高速度 npy_data = np.load(data_path)["x"] npy_labels = np.load(data_path)["y"] print("Load images by npz") image_data = npy_data labels_data = npy_labels
At this point, the processing and preprocessing of the original data has been completed. Only the last step is needed, just like in the demo fashion_mnist.load_data()
The results returned are the same. The code is as follows:
# 最后一步就是将原始数据分成训练数据和测试数据 train_images, test_images, train_labels, test_labels = \ train_test_split(image_data, labels_data, test_size=0.2, random_state=6)
The method of printing relevant information is also attached here:
print("_________________________________________________________________") print("%-28s %-s" % ("Name", "Shape")) print("=================================================================") print("%-28s %-s" % ("Image Data", image_data.shape)) print("%-28s %-s" % ("Labels Data", labels_data.shape)) print("=================================================================") print('Split train and test data,p=%') print("_________________________________________________________________") print("%-28s %-s" % ("Name", "Shape")) print("=================================================================") print("%-28s %-s" % ("Train Images", train_images.shape)) print("%-28s %-s" % ("Test Images", test_images.shape)) print("%-28s %-s" % ("Train Labels", train_labels.shape)) print("%-28s %-s" % ("Test Labels", test_labels.shape)) print("=================================================================")
Don’t forget to normalize after that:
print("Normalize images") train_images = train_images / 255.0 test_images = test_images / 255.0
Finally, the method of printing the relevant information is attached: Complete code defining data:
import os import keras import matplotlib.pyplot as plt from PIL import Image from keras.layers import * from keras.models import * from keras.optimizers import Adam from keras.preprocessing.image import ImageDataGenerator from sklearn.model_selection import train_test_split os.environ['TF_CPP_MIN_LOG_LEVEL'] = '2' # 支持中文 plt.rcParams['font.sans-serif'] = ['SimHei'] # 用来正常显示中文标签 plt.rcParams['axes.unicode_minus'] = False # 用来正常显示负号 re_load = False re_build = False # re_load = True re_build = True epochs = 50 batch_size = 5 count = 0 max_size = 2000000000 IMG_SIZE_X = 30 IMG_SIZE_Y = 30 np.random.seed(9277) image_path = r'D:\Projects\ImageClassifier\data\set' path = ".\data" data_name = "data.npz" data_path = os.path.join(path, data_name) model_name = "model.h5" model_path = os.path.join(path, model_name) label_name = "labels.txt" label_path = os.path.join(path, label_name) class_names = np.loadtxt(label_path, type("")) print('Load class names') if not os.path.exists(data_path) or re_load: labels = [] images = [] print('Handle images') for index, name in enumerate(class_names): classpath = os.path.join(image_path, name) if not os.path.isdir(classpath): continue limit = 0 for image_name in os.listdir(classpath): if limit >= max_size: break imagepath = os.path.join(classpath, image_name) count = count + 1 limit = limit + 1 img = Image.open(imagepath) img = img.resize((30, 30)) img = img.convert("L") img = np.array(img) img = np.reshape(img, (1, 30, 30)) # img = skimage.io.imread(imagepath, as_grey=True) # if img.shape[2] != 3: # print("{} shape is {}".format(image_name, img.shape)) # continue # data = transform.resize(img, (IMG_SIZE_X, IMG_SIZE_Y)) labels.append([index]) images.append(img) print("{} class: {} {} limit: {} {}" .format(count, index + 1, class_names[index], limit, imagepath)) npy_data = np.array(images) npy_labels = np.array(labels) np.savez(data_path, x=npy_data, y=npy_labels) print("Save images by npz") else: npy_data = np.load(data_path)["x"] npy_labels = np.load(data_path)["y"] print("Load images by npz") image_data = npy_data labels_data = npy_labels print("_________________________________________________________________") print("%-28s %-s" % ("Name", "Shape")) print("=================================================================") print("%-28s %-s" % ("Image Data", image_data.shape)) print("%-28s %-s" % ("Labels Data", labels_data.shape)) print("=================================================================") train_images, test_images, train_labels, test_labels = \ train_test_split(image_data, labels_data, test_size=0.2, random_state=6) print('Split train and test data,p=%') print("_________________________________________________________________") print("%-28s %-s" % ("Name", "Shape")) print("=================================================================") print("%-28s %-s" % ("Train Images", train_images.shape)) print("%-28s %-s" % ("Test Images", test_images.shape)) print("%-28s %-s" % ("Train Labels", train_labels.shape)) print("%-28s %-s" % ("Test Labels", test_labels.shape)) print("=================================================================") # 归一化 # 我们将这些值缩小到 0 到 1 之间,然后将其馈送到神经网络模型。为此,将图像组件的数据类型从整数转换为浮点数,然后除以 255。以下是预处理图像的函数: # 务必要以相同的方式对训练集和测试集进行预处理: print("Normalize images") train_images = train_images / 255.0 test_images = test_images / 255.0
The above is the detailed content of Introduction to the method of reading custom data for Tensorflow classifier project (code example). For more information, please follow other related articles on the PHP Chinese website!

Hot AI Tools

Undresser.AI Undress
AI-powered app for creating realistic nude photos

AI Clothes Remover
Online AI tool for removing clothes from photos.

Undress AI Tool
Undress images for free

Clothoff.io
AI clothes remover

Video Face Swap
Swap faces in any video effortlessly with our completely free AI face swap tool!

Hot Article

Hot Tools

Notepad++7.3.1
Easy-to-use and free code editor

SublimeText3 Chinese version
Chinese version, very easy to use

Zend Studio 13.0.1
Powerful PHP integrated development environment

Dreamweaver CS6
Visual web development tools

SublimeText3 Mac version
God-level code editing software (SublimeText3)

Hot Topics
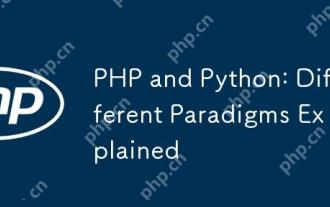
PHP is mainly procedural programming, but also supports object-oriented programming (OOP); Python supports a variety of paradigms, including OOP, functional and procedural programming. PHP is suitable for web development, and Python is suitable for a variety of applications such as data analysis and machine learning.
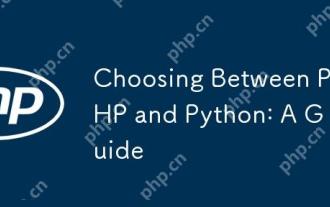
PHP is suitable for web development and rapid prototyping, and Python is suitable for data science and machine learning. 1.PHP is used for dynamic web development, with simple syntax and suitable for rapid development. 2. Python has concise syntax, is suitable for multiple fields, and has a strong library ecosystem.
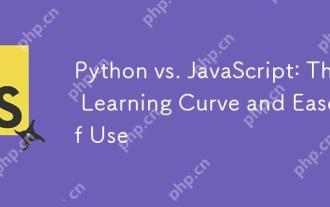
Python is more suitable for beginners, with a smooth learning curve and concise syntax; JavaScript is suitable for front-end development, with a steep learning curve and flexible syntax. 1. Python syntax is intuitive and suitable for data science and back-end development. 2. JavaScript is flexible and widely used in front-end and server-side programming.
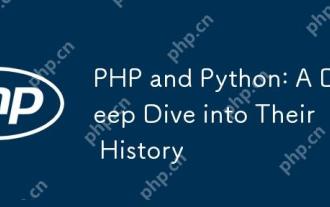
PHP originated in 1994 and was developed by RasmusLerdorf. It was originally used to track website visitors and gradually evolved into a server-side scripting language and was widely used in web development. Python was developed by Guidovan Rossum in the late 1980s and was first released in 1991. It emphasizes code readability and simplicity, and is suitable for scientific computing, data analysis and other fields.
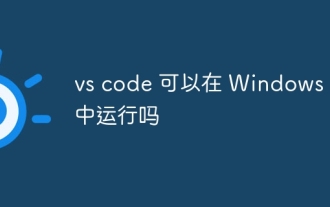
VS Code can run on Windows 8, but the experience may not be great. First make sure the system has been updated to the latest patch, then download the VS Code installation package that matches the system architecture and install it as prompted. After installation, be aware that some extensions may be incompatible with Windows 8 and need to look for alternative extensions or use newer Windows systems in a virtual machine. Install the necessary extensions to check whether they work properly. Although VS Code is feasible on Windows 8, it is recommended to upgrade to a newer Windows system for a better development experience and security.
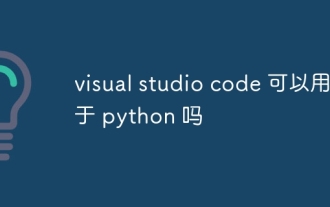
VS Code can be used to write Python and provides many features that make it an ideal tool for developing Python applications. It allows users to: install Python extensions to get functions such as code completion, syntax highlighting, and debugging. Use the debugger to track code step by step, find and fix errors. Integrate Git for version control. Use code formatting tools to maintain code consistency. Use the Linting tool to spot potential problems ahead of time.
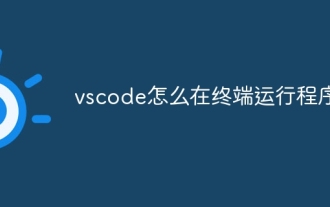
In VS Code, you can run the program in the terminal through the following steps: Prepare the code and open the integrated terminal to ensure that the code directory is consistent with the terminal working directory. Select the run command according to the programming language (such as Python's python your_file_name.py) to check whether it runs successfully and resolve errors. Use the debugger to improve debugging efficiency.
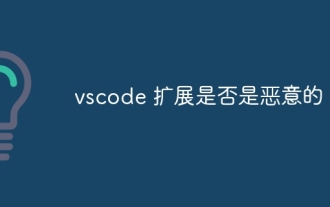
VS Code extensions pose malicious risks, such as hiding malicious code, exploiting vulnerabilities, and masturbating as legitimate extensions. Methods to identify malicious extensions include: checking publishers, reading comments, checking code, and installing with caution. Security measures also include: security awareness, good habits, regular updates and antivirus software.
