


Introduction to four methods of Python data visualization (with examples)
This article brings you an introduction to four methods of Python data visualization (with examples). It has certain reference value. Friends in need can refer to it. Hope it helps.
Abstract: This article describes four Python data visualization methods: heat map, two-dimensional density map, spider map, and tree map.
Data visualization is an important part of any data science or machine learning project. People often start with exploratory data analysis (EDA) to gain a deeper understanding of their data, and creating visualizations can really help make problems clearer and easier to understand, especially for those larger, high-dimensional data sets. At the end of a project, it's important to be able to present the end result in a clear, concise and convincing way that your users can understand and understand.
You may have read my previous article "5 Quick and Easy Data Visualizations in Python with Code" , which introduces 5 basic visualization methods: scatter plots, line plots, histograms, bar plots, and box plots. These five are simple yet powerful visualization methods with which you can definitely get huge gains from your data sets. In this article, we will introduce 4 other data visualization methods, but they are slightly more complicated. You can use them after reading the basic methods introduced in the previous article.
Heat Map
Heat map is a matrix representation of data, in which each matrix value is represented by a color. Different colors represent different levels, and the matrix index connects two contrasting columns or features together. Heatmaps are great at showing the relationships between multiple feature variables because one level can be viewed directly as a color. You can also see how each relationship compares to other relationships in the data set by looking at some points in the heat map. The colors do provide a simple representation as it is very intuitive.# Importing libs importseaborn as sns import pandas aspd importnumpyasnp importmatplotlib.pyplotasplt # Create a random dataset data=pd.DataFrame(np.random.random((10,6)), columns=["Iron Man","CaptainAmerica","BlackWidow","Thor","Hulk", "Hawkeye"]) print(data) # Plot the heatmap heatmap_plot=sns.heatmap(data, center=0, cmap='gist_ncar') plt.show()
Two-dimensional density plot (2D Density Plot)
The two-dimensional density plot is a simple extension of the one-dimensional version, which can see the probability distribution of two variables . Let’s look at the 2D density plot below. The scale on the right represents the probability of each point in color. The highest probability, looking at the data set, seems to be about 0.5 size and 1.4-ish speed. As you can see, 2D density plots are great for quickly determining the areas of the data that are most concentrated for two variables, rather than just one variable like 1D density plots. Two-dimensional density plots are particularly useful when you have two variables that are important to the output and want to understand how they together contribute to the distribution of the output.Spider Plot
Spider diagram is one of the best ways to display one-to-many relationships. That is, you can plot and view the values of multiple variables as distinct from a single variable or category. In a spider diagram, the properties of one variable relative to another are apparent because the area and length vary in some directions. If you want to see how the variables stack up for several categories, plot them side by side. In the image below, it's easy to compare the different attributes of three movie characters and see where their strengths lie!# Import libs import pandas aspd importseabornassns importnumpyasnp importmatplotlib.pyplotasplt # Get the data df=pd.read_csv("avengers_data.csv") print(df) """ # Name Attack Defense Speed Range Health 0 1 Iron Man 83 80 75 70 70 1 2 Captain America 60 62 63 80 80 2 3 Thor 80 82 83 100 100 3 3 Hulk 80 100 67 44 92 4 4 Black Widow 52 43 60 50 65 5 5 Hawkeye 58 64 58 80 65 """ # Get the data for Iron Man labels=np.array(["Attack","Defense","Speed","Range","Health"]) stats=df.loc[0,labels].values # Make some calculations for the plot angles=np.linspace(0, 2*np.pi, len(labels), endpoint=False) stats=np.concatenate((stats,[stats[0]])) angles=np.concatenate((angles,[angles[0]])) # Plot stuff fig=plt.figure() ax=fig.add_subplot(111, polar=True) ax.plot(angles, stats, 'o-', linewidth=2) ax.fill(angles, stats, alpha=0.25) ax.set_thetagrids(angles *180/np.pi, labels) ax.set_title([df.loc[0,"Name"]]) ax.grid(True) plt.show()
Tree Diagram
We have been using tree diagrams since elementary school. Tree diagrams are natural, intuitive, and easy to explain. Nodes that are directly connected are closely related and are very different from nodes that have multiple connections. In the image below, I have plotted a small portion of thePokemon with stats data set from Kaggle based on statistics:
HP, Attack, Defense, Special Attack, Special Defense, Speed因此,与stats wise最匹配的Pokemon将紧密连接在一起。例如,我们看到,在顶部,Arbok和Fearow是直接连接的,而且,如果我们查看数据,Arbok总共有438个,而Fearow有442个,非常接近。但是一旦我们移动到Raticate,我们得到的总数是413,这与Arbok和Fearow的差别很大,这就是它们被分开的原因。当我们移动树的时候,基于相似性,Pokemon被分的组越来越多。在绿色组中的Pokemon相互之间比红色组中的更相似,即使没有直接的绿色连接。
对于树形图,我们实际上要使用Scipy的。在查看了数据集之后,我们将去掉字符串类型的列。我们这么做只是为了要得到正确的可视化结果,但在实践中,最好是把这些字符串转换成分类变量,为了得到更好的结果和进行比较,我们还设置了数据帧索引,以便能够适当地用它作为引用每个节点的列。最后,在Scipy中计算和绘制树形图是非常简单的事了。
# Import libs import pandas aspd frommatplotlibimportpyplotasplt fromscipy.clusterimport hierarchy importnumpyasnp # Read in the dataset # Drop any fields that are strings # Only get the first 40 because this dataset is big df=pd.read_csv('Pokemon.csv') df=df.set_index('Name') del df.index.name df=df.drop(["Type 1", "Type 2", "Legendary"], axis=1) df=df.head(n=40) # Calculate the distance between each sample Z =hierarchy.linkage(df, 'ward') # Orientation our tree hierarchy.dendrogram(Z, orientation="left", labels=df.index) plt.show()
The above is the detailed content of Introduction to four methods of Python data visualization (with examples). For more information, please follow other related articles on the PHP Chinese website!

Hot AI Tools

Undresser.AI Undress
AI-powered app for creating realistic nude photos

AI Clothes Remover
Online AI tool for removing clothes from photos.

Undress AI Tool
Undress images for free

Clothoff.io
AI clothes remover

Video Face Swap
Swap faces in any video effortlessly with our completely free AI face swap tool!

Hot Article

Hot Tools

Notepad++7.3.1
Easy-to-use and free code editor

SublimeText3 Chinese version
Chinese version, very easy to use

Zend Studio 13.0.1
Powerful PHP integrated development environment

Dreamweaver CS6
Visual web development tools

SublimeText3 Mac version
God-level code editing software (SublimeText3)

Hot Topics
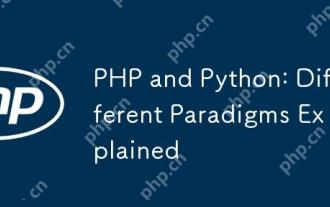
PHP is mainly procedural programming, but also supports object-oriented programming (OOP); Python supports a variety of paradigms, including OOP, functional and procedural programming. PHP is suitable for web development, and Python is suitable for a variety of applications such as data analysis and machine learning.
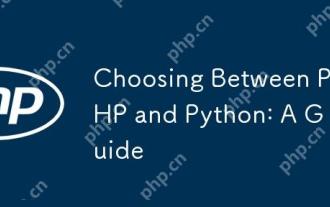
PHP is suitable for web development and rapid prototyping, and Python is suitable for data science and machine learning. 1.PHP is used for dynamic web development, with simple syntax and suitable for rapid development. 2. Python has concise syntax, is suitable for multiple fields, and has a strong library ecosystem.
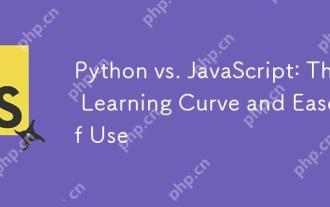
Python is more suitable for beginners, with a smooth learning curve and concise syntax; JavaScript is suitable for front-end development, with a steep learning curve and flexible syntax. 1. Python syntax is intuitive and suitable for data science and back-end development. 2. JavaScript is flexible and widely used in front-end and server-side programming.
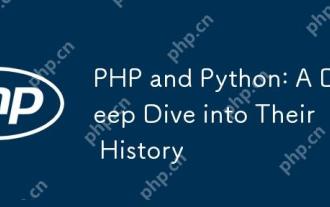
PHP originated in 1994 and was developed by RasmusLerdorf. It was originally used to track website visitors and gradually evolved into a server-side scripting language and was widely used in web development. Python was developed by Guidovan Rossum in the late 1980s and was first released in 1991. It emphasizes code readability and simplicity, and is suitable for scientific computing, data analysis and other fields.
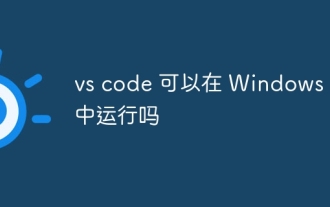
VS Code can run on Windows 8, but the experience may not be great. First make sure the system has been updated to the latest patch, then download the VS Code installation package that matches the system architecture and install it as prompted. After installation, be aware that some extensions may be incompatible with Windows 8 and need to look for alternative extensions or use newer Windows systems in a virtual machine. Install the necessary extensions to check whether they work properly. Although VS Code is feasible on Windows 8, it is recommended to upgrade to a newer Windows system for a better development experience and security.
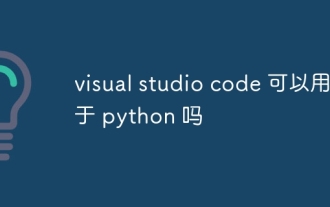
VS Code can be used to write Python and provides many features that make it an ideal tool for developing Python applications. It allows users to: install Python extensions to get functions such as code completion, syntax highlighting, and debugging. Use the debugger to track code step by step, find and fix errors. Integrate Git for version control. Use code formatting tools to maintain code consistency. Use the Linting tool to spot potential problems ahead of time.
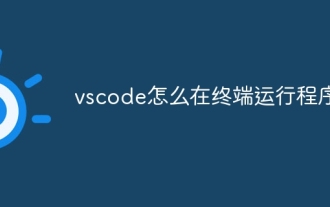
In VS Code, you can run the program in the terminal through the following steps: Prepare the code and open the integrated terminal to ensure that the code directory is consistent with the terminal working directory. Select the run command according to the programming language (such as Python's python your_file_name.py) to check whether it runs successfully and resolve errors. Use the debugger to improve debugging efficiency.
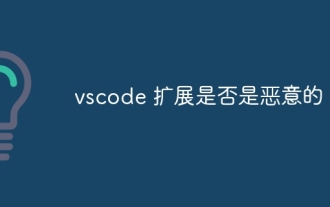
VS Code extensions pose malicious risks, such as hiding malicious code, exploiting vulnerabilities, and masturbating as legitimate extensions. Methods to identify malicious extensions include: checking publishers, reading comments, checking code, and installing with caution. Security measures also include: security awareness, good habits, regular updates and antivirus software.
