Simple example of numpy array splicing_python
This article mainly introduces a simple example of numpy array splicing, including an introduction to numpy arrays, properties of numpy arrays, etc. It has certain reference value and friends in need can refer to it.
NumPy array is a multidimensional array object called ndarray. It consists of two parts:
·The actual data
·Metadata describing these data
Most operations Only for metadata, without changing the underlying actual data.
There are a few things you need to know about NumPy arrays:
·The subscripts of NumPy arrays start from 0.
·The types of all elements in the same NumPy array must be the same.
NumPy array properties
Before introducing NumPy arrays in detail. First, let’s introduce the basic properties of NumPy arrays in detail. The dimensionality of a NumPy array is called rank. A one-dimensional array has a rank of 1, a two-dimensional array has a rank of 2, and so on. In NumPy, each linear array is called an axis (axes), and the rank actually describes the number of axes. For example, a two-dimensional array is equivalent to two one-dimensional arrays, where each element in the first one-dimensional array is another one-dimensional array. So a one-dimensional array is the axes (axes) in NumPy. The first axis is equivalent to the underlying array, and the second axis is the array in the underlying array. The number of axes, the rank, is the dimension of the array.
The more important ndarray object attributes in NumPy arrays are:
1.ndarray.ndim: The dimension of the array (that is, the number of array axes), which is equal to the rank. The most common is a two-dimensional array (matrix).
2.ndarray.shape: Dimensions of the array. is a tuple of integers representing the size of the array in each dimension. For example, in a two-dimensional array, it represents the "number of rows" and "number of columns" of the array. ndarray.shape returns a tuple whose length is the number of dimensions, that is, the ndim attribute.
3.ndarray.size: The total number of array elements is equal to the product of the tuple elements in the shape attribute.
4.ndarray.dtype: An object representing the type of elements in the array. You can use standard Python types to create or specify dtype. In addition, you can also use the data types provided by NumPy introduced in the previous article.
5.ndarray.itemsize: The byte size of each element in the array. For example, the itemsiz attribute value of an array whose element type is float64 is 8 (float64 occupies 64 bits, and each byte is 8 in length, so 64/8 takes up 8 bytes). Another example is an array whose element type is complex32. The array item attribute is 4 (32/8).
6.ndarray.data: Buffer containing actual array elements. Since elements are generally obtained through the index of the array, there is usually no need to use this attribute.
Array splicing method one
Idea: first convert the array into a list, and then use the list splicing functions append() and extend() Wait for splicing processing, and finally convert the list into an array.
Example 1:
>>> import numpy as np >>> a=np.array([1,2,5]) >>> b=np.array([10,12,15]) >>> a_list=list(a) >>> b_list=list(b) >>> a_list.extend(b_list) >>> a_list [1, 2, 5, 10, 12, 15] >>> a=np.array(a_list) >>> a array([ 1, 2, 5, 10, 12, 15])
This method is only suitable for simple one-dimensional array splicing, because the conversion process is very It is time-consuming and is generally not recommended for splicing large amounts of data.
Array splicing method two
Idea: numpy provides the numpy.append(arr, values, axis=None) function. For parameter specifications, there must be either one array and one value; or two arrays. Three or more arrays cannot be directly appended and spliced. The append function always returns a one-dimensional array.
Example 2:
>>> a=np.arange(5) >>> a array([0, 1, 2, 3, 4]) >>> np.append(a,10) array([ 0, 1, 2, 3, 4, 10]) >>> a array([0, 1, 2, 3, 4]) >>> b=np.array([11,22,33]) >>> b array([11, 22, 33]) >>> np.append(a,b) array([ 0, 1, 2, 3, 4, 11, 22, 33]) >>> a array([[1, 2, 3], [4, 5, 6]]) >>> b=np.array([[7,8,9],[10,11,12]]) >>> b array([[ 7, 8, 9], [10, 11, 12]]) >>> np.append(a,b) array([ 1, 2, 3, 4, 5, 6, 7, 8, 9, 10, 11, 12])
numpy array does not have the function of dynamically changing the size, numpy.append() function Each time the entire array is reallocated and the original array is copied to the new array.
Array splicing method three
Idea: numpy provides numpy.concatenate((a1,a2,...),axis=0 )function. Able to complete the splicing of multiple arrays at one time. Where a1, a2,... are parameters of array type
Example 3:
>>> a=np.array([1,2,3]) >>> b=np.array([11,22,33]) >>> c=np.array([44,55,66]) >>> np.concatenate((a,b,c),axis=0) # 默认情况下,axis=0可以不写 array([ 1, 2, 3, 11, 22, 33, 44, 55, 66]) #对于一维数组拼接,axis的值不影响最后的结果 >>> a=np.array([[1,2,3],[4,5,6]]) >>> b=np.array([[11,21,31],[7,8,9]]) >>> np.concatenate((a,b),axis=0) array([[ 1, 2, 3], [ 4, 5, 6], [11, 21, 31], [ 7, 8, 9]]) >>> np.concatenate((a,b),axis=1) #axis=1表示对应行的数组进行拼接 array([[ 1, 2, 3, 11, 21, 31], [ 4, 5, 6, 7, 8, 9]])
to numpy. Compare the running time of the two functions append() and numpy.concatenate()
Example 4:
>>> from time import clock as now >>> a=np.arange(9999) >>> b=np.arange(9999) >>> time1=now() >>> c=np.append(a,b) >>> time2=now() >>> print time2-time1 28.2316728446 >>> a=np.arange(9999) >>> b=np.arange(9999) >>> time1=now() >>> c=np.concatenate((a,b),axis=0) >>> time2=now() >>> print time2-time1 20.3934997107
It can be seen that concatenate() is more efficient and suitable for large-scale data splicing
Summary
The above is a simple example of numpy array splicing in this article All the content, I hope it will be helpful to everyone. Interested friends can continue to refer to this site:
Related recommendations:
Python programming for numpy Example of a method for adding a column to a matrix
Python’s method of creating a symmetric matrix based on the numpy module
The above is the detailed content of Simple example of numpy array splicing_python. For more information, please follow other related articles on the PHP Chinese website!

Hot AI Tools

Undresser.AI Undress
AI-powered app for creating realistic nude photos

AI Clothes Remover
Online AI tool for removing clothes from photos.

Undress AI Tool
Undress images for free

Clothoff.io
AI clothes remover

Video Face Swap
Swap faces in any video effortlessly with our completely free AI face swap tool!

Hot Article

Hot Tools

Notepad++7.3.1
Easy-to-use and free code editor

SublimeText3 Chinese version
Chinese version, very easy to use

Zend Studio 13.0.1
Powerful PHP integrated development environment

Dreamweaver CS6
Visual web development tools

SublimeText3 Mac version
God-level code editing software (SublimeText3)

Hot Topics
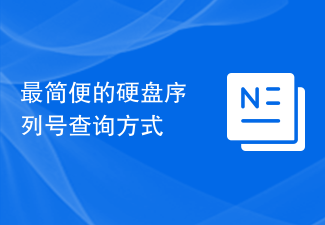
The hard disk serial number is an important identifier of the hard disk and is usually used to uniquely identify the hard disk and identify the hardware. In some cases, we may need to query the hard drive serial number, such as when installing an operating system, finding the correct device driver, or performing hard drive repairs. This article will introduce some simple methods to help you check the hard drive serial number. Method 1: Use Windows Command Prompt to open the command prompt. In Windows system, press Win+R keys, enter "cmd" and press Enter key to open the command
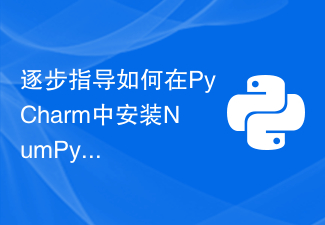
Teach you step by step to install NumPy in PyCharm and make full use of its powerful functions. Preface: NumPy is one of the basic libraries for scientific computing in Python. It provides high-performance multi-dimensional array objects and various functions required to perform basic operations on arrays. function. It is an important part of most data science and machine learning projects. This article will introduce you to how to install NumPy in PyCharm, and demonstrate its powerful features through specific code examples. Step 1: Install PyCharm First, we
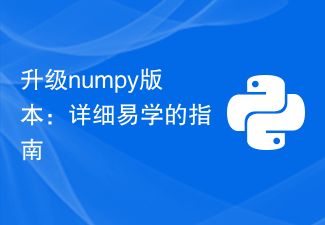
How to upgrade numpy version: Easy-to-follow tutorial, requires concrete code examples Introduction: NumPy is an important Python library used for scientific computing. It provides a powerful multidimensional array object and a series of related functions that can be used to perform efficient numerical operations. As new versions are released, newer features and bug fixes are constantly available to us. This article will describe how to upgrade your installed NumPy library to get the latest features and resolve known issues. Step 1: Check the current NumPy version at the beginning
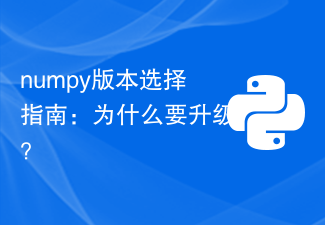
With the rapid development of fields such as data science, machine learning, and deep learning, Python has become a mainstream language for data analysis and modeling. In Python, NumPy (short for NumericalPython) is a very important library because it provides a set of efficient multi-dimensional array objects and is the basis for many other libraries such as pandas, SciPy and scikit-learn. In the process of using NumPy, you are likely to encounter compatibility issues between different versions, then
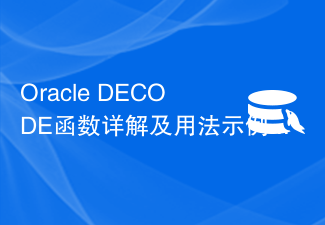
The DECODE function in Oracle is a conditional expression that is often used to return different results based on different conditions in query statements. This article will introduce the syntax, usage and sample code of the DECODE function in detail. 1. DECODE function syntax DECODE(expr,search1,result1[,search2,result2,...,default]) expr: the expression or field to be compared. search1,
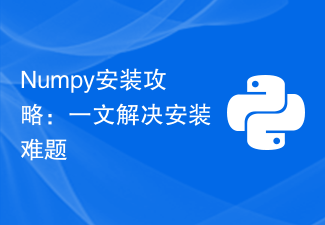
Numpy installation guide: One article to solve installation problems, need specific code examples Introduction: Numpy is a powerful scientific computing library in Python. It provides efficient multi-dimensional array objects and tools for operating array data. However, for beginners, installing Numpy may cause some confusion. This article will provide you with a Numpy installation guide to help you quickly solve installation problems. 1. Install the Python environment: Before installing Numpy, you first need to make sure that Py is installed.
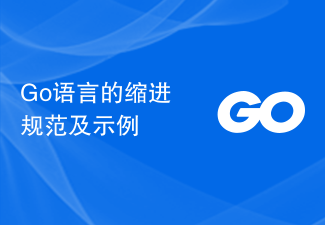
Indentation specifications and examples of Go language Go language is a programming language developed by Google. It is known for its concise and clear syntax, in which indentation specifications play a crucial role in the readability and beauty of the code. effect. This article will introduce the indentation specifications of the Go language and explain in detail through specific code examples. Indentation specifications In the Go language, tabs are used for indentation instead of spaces. Each level of indentation is one tab, usually set to a width of 4 spaces. Such specifications unify the coding style and enable teams to work together to compile
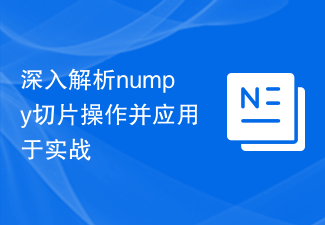
Detailed explanation of numpy slicing operation method and practical application guide Introduction: Numpy is one of the most popular scientific computing libraries in Python, providing powerful array operation functions. Among them, slicing operation is one of the commonly used and powerful functions in numpy. This article will introduce the slicing operation method in numpy in detail, and demonstrate the specific use of slicing operation through practical application guide. 1. Introduction to numpy slicing operation method Numpy slicing operation refers to obtaining a subset of an array by specifying an index interval. Its basic form is:
