What are the drawbacks of over-normalization?
What are the drawbacks of over-normalization?
Over-normalization, which refers to the process of breaking down data into too many tables in a database, can lead to several drawbacks. Firstly, it can result in increased complexity in the database design. As data is split into more and more tables, the relationships between these tables become more intricate, making it harder to understand and maintain the database structure. This complexity can lead to errors in data management and retrieval.
Secondly, over-normalization can negatively impact database performance. The need to join multiple tables to retrieve data can slow down query execution times, as the database engine has to perform more operations to gather the required information. This can be particularly problematic in large databases or in applications where quick data retrieval is crucial.
Thirdly, over-normalization can lead to data integrity issues. While normalization is intended to reduce data redundancy and improve data integrity, overdoing it can have the opposite effect. For instance, if data is spread across too many tables, maintaining referential integrity becomes more challenging, and the risk of data inconsistencies increases.
Lastly, over-normalization can make it more difficult to scale the database. As the number of tables grows, so does the complexity of scaling operations, which can hinder the ability to adapt the database to changing business needs.
What impact can over-normalization have on data integrity?
Over-normalization can have a significant impact on data integrity, primarily by increasing the risk of data inconsistencies and making it more challenging to maintain referential integrity. When data is excessively normalized, it is spread across numerous tables, which means that maintaining the relationships between these tables becomes more complex. This complexity can lead to errors in data entry or updates, where changes in one table may not be correctly reflected in related tables.
For example, if a piece of data is updated in one table, ensuring that all related tables are updated correctly can be difficult. This can result in data anomalies, where the data in different tables becomes inconsistent. Such inconsistencies can compromise the accuracy and reliability of the data, leading to potential issues in data analysis and decision-making processes.
Additionally, over-normalization can make it harder to enforce data integrity constraints, such as foreign key relationships. With more tables to manage, the likelihood of overlooking or incorrectly implementing these constraints increases, further jeopardizing data integrity.
How does over-normalization affect database performance?
Over-normalization can adversely affect database performance in several ways. The primary impact is on query performance. When data is spread across numerous tables, retrieving it often requires joining multiple tables. Each join operation adds to the complexity and time required to execute a query. In large databases, this can lead to significantly slower query response times, which can be detrimental to applications that rely on quick data access.
Moreover, over-normalization can increase the load on the database server. The need to perform more joins and manage more tables can lead to higher CPU and memory usage, which can slow down the overall performance of the database system. This is particularly problematic in environments where the database is handling a high volume of transactions or concurrent users.
Additionally, over-normalization can complicate indexing strategies. With more tables, deciding which columns to index and how to optimize these indexes becomes more challenging. Poor indexing can further degrade query performance, as the database engine may struggle to efficiently locate and retrieve the required data.
In summary, over-normalization can lead to slower query execution, increased server load, and more complex indexing, all of which can negatively impact database performance.
Can over-normalization lead to increased complexity in database design?
Yes, over-normalization can indeed lead to increased complexity in database design. When data is excessively normalized, it is broken down into numerous smaller tables, each containing a subset of the data. This results in a more intricate network of relationships between tables, which can make the overall database structure more difficult to understand and manage.
The increased number of tables and relationships can lead to several challenges in database design. Firstly, it becomes harder to visualize and document the database schema. With more tables to keep track of, creating clear and comprehensive documentation becomes more time-consuming and error-prone.
Secondly, the complexity of the database design can make it more difficult to implement changes or updates. Modifying the schema of an over-normalized database can be a daunting task, as changes in one table may have ripple effects across many other tables. This can lead to increased development time and a higher risk of introducing errors during the modification process.
Lastly, over-normalization can complicate the process of database maintenance and troubleshooting. Identifying and resolving issues in a highly normalized database can be more challenging due to the intricate relationships between tables. This can lead to longer resolution times and increased maintenance costs.
In conclusion, over-normalization can significantly increase the complexity of database design, making it harder to manage, modify, and maintain the database.
The above is the detailed content of What are the drawbacks of over-normalization?. For more information, please follow other related articles on the PHP Chinese website!

Hot AI Tools

Undresser.AI Undress
AI-powered app for creating realistic nude photos

AI Clothes Remover
Online AI tool for removing clothes from photos.

Undress AI Tool
Undress images for free

Clothoff.io
AI clothes remover

Video Face Swap
Swap faces in any video effortlessly with our completely free AI face swap tool!

Hot Article

Hot Tools

Notepad++7.3.1
Easy-to-use and free code editor

SublimeText3 Chinese version
Chinese version, very easy to use

Zend Studio 13.0.1
Powerful PHP integrated development environment

Dreamweaver CS6
Visual web development tools

SublimeText3 Mac version
God-level code editing software (SublimeText3)

Hot Topics
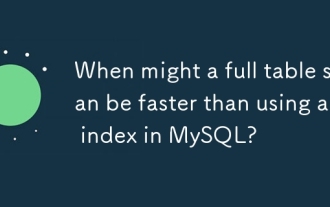
Full table scanning may be faster in MySQL than using indexes. Specific cases include: 1) the data volume is small; 2) when the query returns a large amount of data; 3) when the index column is not highly selective; 4) when the complex query. By analyzing query plans, optimizing indexes, avoiding over-index and regularly maintaining tables, you can make the best choices in practical applications.
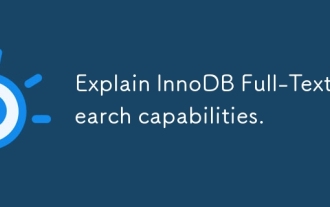
InnoDB's full-text search capabilities are very powerful, which can significantly improve database query efficiency and ability to process large amounts of text data. 1) InnoDB implements full-text search through inverted indexing, supporting basic and advanced search queries. 2) Use MATCH and AGAINST keywords to search, support Boolean mode and phrase search. 3) Optimization methods include using word segmentation technology, periodic rebuilding of indexes and adjusting cache size to improve performance and accuracy.
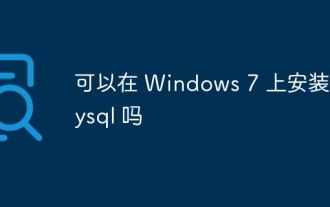
Yes, MySQL can be installed on Windows 7, and although Microsoft has stopped supporting Windows 7, MySQL is still compatible with it. However, the following points should be noted during the installation process: Download the MySQL installer for Windows. Select the appropriate version of MySQL (community or enterprise). Select the appropriate installation directory and character set during the installation process. Set the root user password and keep it properly. Connect to the database for testing. Note the compatibility and security issues on Windows 7, and it is recommended to upgrade to a supported operating system.
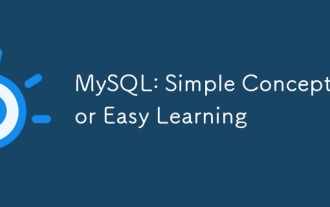
MySQL is an open source relational database management system. 1) Create database and tables: Use the CREATEDATABASE and CREATETABLE commands. 2) Basic operations: INSERT, UPDATE, DELETE and SELECT. 3) Advanced operations: JOIN, subquery and transaction processing. 4) Debugging skills: Check syntax, data type and permissions. 5) Optimization suggestions: Use indexes, avoid SELECT* and use transactions.
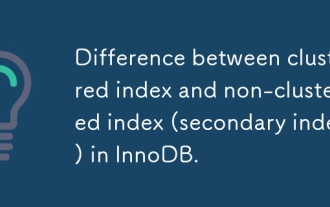
The difference between clustered index and non-clustered index is: 1. Clustered index stores data rows in the index structure, which is suitable for querying by primary key and range. 2. The non-clustered index stores index key values and pointers to data rows, and is suitable for non-primary key column queries.
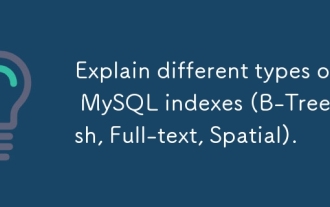
MySQL supports four index types: B-Tree, Hash, Full-text, and Spatial. 1.B-Tree index is suitable for equal value search, range query and sorting. 2. Hash index is suitable for equal value searches, but does not support range query and sorting. 3. Full-text index is used for full-text search and is suitable for processing large amounts of text data. 4. Spatial index is used for geospatial data query and is suitable for GIS applications.
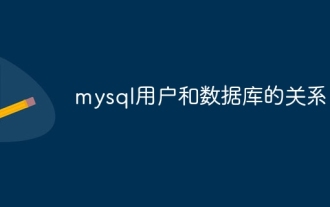
In MySQL database, the relationship between the user and the database is defined by permissions and tables. The user has a username and password to access the database. Permissions are granted through the GRANT command, while the table is created by the CREATE TABLE command. To establish a relationship between a user and a database, you need to create a database, create a user, and then grant permissions.
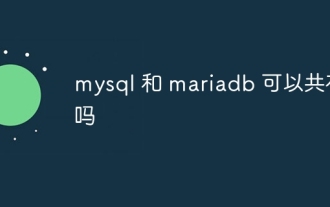
MySQL and MariaDB can coexist, but need to be configured with caution. The key is to allocate different port numbers and data directories to each database, and adjust parameters such as memory allocation and cache size. Connection pooling, application configuration, and version differences also need to be considered and need to be carefully tested and planned to avoid pitfalls. Running two databases simultaneously can cause performance problems in situations where resources are limited.
