


Describe the process of ETL (Extract, Transform, Load). How can you implement an ETL pipeline in Python?
Describe the process of ETL (Extract, Transform, Load). How can you implement an ETL pipeline in Python?
ETL, which stands for Extract, Transform, Load, is a crucial process in data management and analytics. The three stages of ETL are:
- Extract: This stage involves extracting data from various sources, which could be databases, APIs, or even flat files. The data collected can be structured or unstructured and is pulled into a staging area for further processing.
- Transform: In this stage, the extracted data is transformed to meet the business and technical needs of the target system. This can include data cleansing, deduplication, filtering, sorting, and converting data types. The goal is to make the data consistent and ready for loading into the target system.
- Load: The final stage involves loading the transformed data into the target database or data warehouse. This can be done in batches or real-time, depending on the requirements of the system.
To implement an ETL pipeline in Python, you can follow these steps:
-
Extract: Use libraries like
requests
for API data,pandas
for reading CSV files, orSQLAlchemy
for database connections to extract data. Here’s a basic example usingpandas
:import pandas as pd # Extracting data from a CSV file df = pd.read_csv('data.csv')
Copy after login Transform: Use
pandas
to perform various transformations on the dataframe, such as cleaning and reshaping the data:# Transforming data (e.g., removing duplicates and handling missing values) df.drop_duplicates(inplace=True) df.fillna(0, inplace=True) # Replace missing values with 0
Copy after loginLoad: Finally, load the transformed data into the target system. For example, to load data into a SQL database:
from sqlalchemy import create_engine # Creating a SQL engine engine = create_engine('postgresql://username:password@localhost:5432/mydatabase') # Loading data into the database df.to_sql('table_name', engine, if_exists='replace', index=False)
Copy after login
What are the common challenges faced during the ETL process and how can they be mitigated?
The ETL process often faces several common challenges, which include:
Data Quality Issues: Poor quality data with errors, inconsistencies, or missing values can lead to unreliable results.
Mitigation: Implement robust data validation and cleansing techniques. Use automated scripts to identify and correct errors. Regular audits and data profiling can help maintain data quality.
Scalability: As data volumes grow, ETL processes need to handle larger datasets efficiently.
Mitigation: Use distributed computing frameworks like Apache Spark, which can scale to process big data. Optimize the ETL process by breaking it into smaller, manageable chunks and using parallel processing.
Complexity of Transformations: Complex business rules and data transformations can be difficult to manage.
Mitigation: Document transformation rules thoroughly and maintain a version control system. Use modular coding practices to handle complexity, making it easier to update or modify transformations.
Performance Bottlenecks: Slow extraction or loading processes can hinder the overall efficiency of the ETL pipeline.
Mitigation: Optimize database queries, use indexing, and leverage in-memory processing where possible. Monitor the ETL process and identify bottlenecks to address them promptly.
Data Security and Compliance: Ensuring that data handling complies with regulations and standards can be challenging.
Mitigation: Implement robust security measures and encryption during data transfer. Regularly audit the ETL process to ensure compliance with data protection laws like GDPR.
Which Python libraries are most effective for building an ETL pipeline and why?
Several Python libraries are effective for building an ETL pipeline, each offering specific functionalities that enhance the ETL process:
Pandas:
- Why: Pandas is ideal for data manipulation and transformation tasks. It offers powerful data structures like DataFrame for easy data handling and manipulation.
- Use Case: Data cleaning, reshaping, and transformation.
SQLAlchemy:
- Why: SQLAlchemy is a SQL toolkit and Object-Relational Mapping (ORM) library that simplifies database operations. It provides a high-level interface to connect to various databases.
- Use Case: Extracting data from and loading data into SQL databases.
Requests:
- Why: Requests is used for making HTTP requests, making it ideal for extracting data from APIs.
- Use Case: Pulling data from RESTful APIs.
Apache Airflow:
- Why: Airflow is a platform for programmatically authoring, scheduling, and monitoring workflows. It helps in orchestrating complex ETL pipelines.
- Use Case: Scheduling and managing the ETL workflow.
PySpark:
- Why: PySpark is the Python API for Apache Spark, offering distributed data processing capabilities. It is essential for handling large-scale data.
- Use Case: Processing big data in a distributed manner.
How do you ensure data quality and integrity during the ETL process in Python?
Ensuring data quality and integrity during the ETL process in Python involves several steps and techniques:
Data Validation:
Use
pandas
to validate data against predefined rules. For example, check for data types, ranges, and formats:import pandas as pd # Validating data types df = pd.read_csv('data.csv') assert df['age'].dtype == 'int64', "Age column should be integer type"
Copy after login
Data Cleansing:
Clean the data by removing duplicates, handling missing values, and correcting errors:
# Removing duplicates df.drop_duplicates(inplace=True) # Handling missing values df['salary'].fillna(df['salary'].mean(), inplace=True)
Copy after login
Data Profiling:
Use libraries like
pandas-profiling
to generate detailed reports on data quality:from pandas_profiling import ProfileReport profile = ProfileReport(df, title="Data Quality Report") profile.to_file("data_quality_report.html")
Copy after login
Automated Testing:
Implement unit tests to ensure transformations are applied correctly:
import unittest class TestETL(unittest.TestCase): def test_data_transformation(self): # Example test case transformed_data = transform_data(raw_data) self.assertEqual(transformed_data['column_name'], expected_value) if __name__ == '__main__': unittest.main()
Copy after login
Logging and Monitoring:
Use logging to track the ETL process and identify issues:
import logging logging.basicConfig(filename='etl.log', level=logging.INFO) logging.info('Starting ETL process')
Copy after login
Checksums and Data Integrity Checks:
Use checksums to ensure data integrity during the load phase:
import hashlib def calculate_checksum(data): return hashlib.md5(str(data).encode()).hexdigest() # Before loading checksum_before = calculate_checksum(df) # After loading loaded_data = pd.read_sql('SELECT * FROM table_name', engine) checksum_after = calculate_checksum(loaded_data) assert checksum_before == checksum_after, "Data integrity compromised"
Copy after login
By implementing these techniques, you can maintain high data quality and integrity throughout the ETL process in Python.
The above is the detailed content of Describe the process of ETL (Extract, Transform, Load). How can you implement an ETL pipeline in Python?. For more information, please follow other related articles on the PHP Chinese website!

Hot AI Tools

Undresser.AI Undress
AI-powered app for creating realistic nude photos

AI Clothes Remover
Online AI tool for removing clothes from photos.

Undress AI Tool
Undress images for free

Clothoff.io
AI clothes remover

Video Face Swap
Swap faces in any video effortlessly with our completely free AI face swap tool!

Hot Article

Hot Tools

Notepad++7.3.1
Easy-to-use and free code editor

SublimeText3 Chinese version
Chinese version, very easy to use

Zend Studio 13.0.1
Powerful PHP integrated development environment

Dreamweaver CS6
Visual web development tools

SublimeText3 Mac version
God-level code editing software (SublimeText3)

Hot Topics
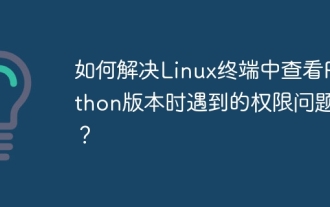
Solution to permission issues when viewing Python version in Linux terminal When you try to view Python version in Linux terminal, enter python...
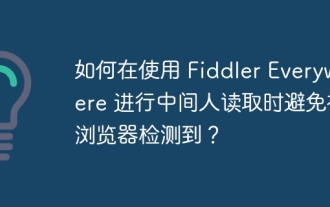
How to avoid being detected when using FiddlerEverywhere for man-in-the-middle readings When you use FiddlerEverywhere...
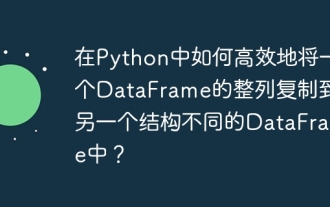
When using Python's pandas library, how to copy whole columns between two DataFrames with different structures is a common problem. Suppose we have two Dats...
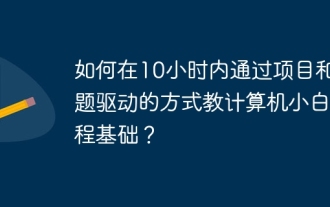
How to teach computer novice programming basics within 10 hours? If you only have 10 hours to teach computer novice some programming knowledge, what would you choose to teach...
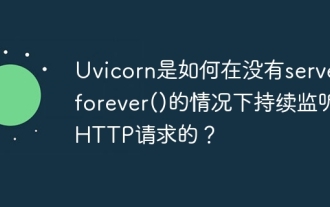
How does Uvicorn continuously listen for HTTP requests? Uvicorn is a lightweight web server based on ASGI. One of its core functions is to listen for HTTP requests and proceed...
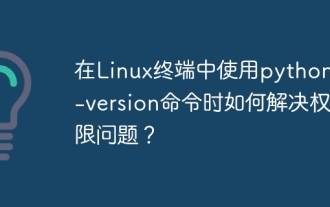
Using python in Linux terminal...
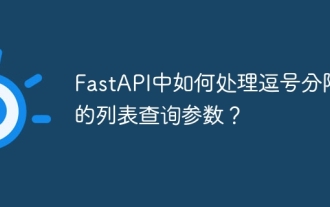
Fastapi ...
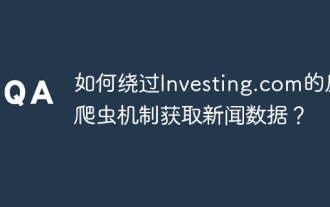
Understanding the anti-crawling strategy of Investing.com Many people often try to crawl news data from Investing.com (https://cn.investing.com/news/latest-news)...
