How do I optimize MongoDB queries using explain plans?
How do I optimize MongoDB queries using explain plans?
To optimize MongoDB queries using explain plans, you first need to understand what an explain plan is and how it helps in query optimization. An explain plan in MongoDB provides detailed information about the execution path of a query, helping you identify potential bottlenecks and areas where performance can be improved.
Here's a step-by-step approach to using explain plans for query optimization:
-
Run the Query with Explain: Append
.explain()
to your query to generate an explain plan. For instance, if your query isdb.collection.find({age: 30})
, you would rundb.collection.find({age: 30}).explain()
. - Analyze the Output: The explain plan's output contains several sections, including 'queryPlanner', 'executionStats', and 'allPlansExecution'. Focus on these sections to understand how the query was executed and what resources were used.
- Check the Query Planner: The 'queryPlanner' section shows the winning plan and any rejected plans. It helps you understand which index was used, if any, and the reasoning behind the choice of the plan.
- Examine Execution Stats: The 'executionStats' section provides metrics like the number of documents scanned, execution time, and memory usage. These metrics are crucial for identifying inefficient queries.
- Iterate Based on Findings: Based on the insights from the explain plan, you can make adjustments such as adding or modifying indexes, restructuring queries, or changing the query's selectivity to improve performance.
-
Re-run the Query with Explain: After making changes, re-run the query with
.explain()
to see if the performance has improved. Compare the new results with the previous ones to assess the impact of your optimizations.
By following this approach, you can iteratively refine your queries to achieve better performance.
What specific metrics should I focus on in MongoDB's explain plan output?
When analyzing MongoDB's explain plan output, there are several key metrics you should focus on to understand and improve query performance:
- nReturned: This metric shows the number of documents returned by the query. A large discrepancy between 'nReturned' and the number of documents scanned (e.g., 'totalDocsExamined') might indicate an inefficient query that could benefit from better indexing.
- executionTimeMillis: This indicates the total time taken to execute the query. A high value here can signal that the query needs optimization, especially if other metrics suggest inefficiencies.
- totalDocsExamined and totalKeysExamined: These metrics show the total number of documents and index keys examined during the query execution. High values relative to 'nReturned' can indicate that the query is not using indexes effectively.
- indexBounds: This section details the range of values that the query scanned within the index. Understanding this helps in assessing whether the index is being used optimally.
- stage: The stage in the 'winningPlan' section shows the sequence of operations MongoDB performed to execute the query. Look for stages like 'COLLSCAN' (collection scan), which indicates that no index was used, leading to slower performance.
- isMultiKey: This indicates whether the index is multi-key, which can impact performance. Multi-key indexes can lead to slower queries, especially for large collections.
By focusing on these metrics, you can gain a comprehensive view of query performance and identify areas for improvement.
How can I interpret the 'winningPlan' section of a MongoDB explain plan to improve query performance?
The 'winningPlan' section in a MongoDB explain plan outlines the chosen execution path for a query. Interpreting this section can help you understand how the query was executed and identify ways to improve its performance. Here's how to do it:
- Identify the Stages: The 'winningPlan' is composed of stages like 'IXSCAN' (index scan), 'FETCH' (document fetch), and 'COLLSCAN' (collection scan). Each stage represents an operation in the query execution process. A 'COLLSCAN' stage indicates that MongoDB scanned the entire collection, which can be inefficient for large datasets.
- Examine Index Usage: Look for 'IXSCAN' stages to see which index was used. If an appropriate index was not used, you may need to add or modify indexes to improve performance.
- Understand Direction and Bounds: The 'direction' and 'indexBounds' fields within an 'IXSCAN' stage show how the index was traversed and which range of values was scanned. A wide range in 'indexBounds' might indicate that the query is not selective enough.
- Check for Multi-Key Indexes: If the 'isMultiKey' field is true, it means the index contains arrays, which can impact performance. Consider whether a multi-key index is necessary or if restructuring the data could improve query performance.
- Analyze Nested Stages: Sometimes, the 'winningPlan' includes nested stages. For instance, an 'IXSCAN' might be nested within a 'FETCH' stage, indicating that the query first scanned the index and then fetched the corresponding documents. Understanding these relationships can help optimize the query.
By carefully interpreting the 'winningPlan' section, you can make informed decisions about indexing, query structure, and data organization to enhance performance.
Can I use the explain plan to identify and resolve index-related issues in MongoDB?
Yes, you can use the explain plan to identify and resolve index-related issues in MongoDB. Here's how:
- Identify Missing Indexes: If the explain plan shows a 'COLLSCAN' stage, it indicates that MongoDB scanned the entire collection instead of using an index. This suggests that a relevant index might be missing. You can create an appropriate index to improve query performance.
- Analyze Index Usage: The 'winningPlan' section shows which index, if any, was used. If the chosen index seems suboptimal, you might need to create a more specific index or restructure the query to leverage existing indexes better.
- Check Index Selectivity: The 'indexBounds' field within an 'IXSCAN' stage shows the range of values scanned. If this range is too broad, the query may not be selective enough. You can create a compound index or modify the query to be more specific.
- Identify Index Overhead: The 'isMultiKey' field indicates whether the index is multi-key. If multi-key indexes are causing performance issues, consider restructuring your data to avoid them or use alternative indexing strategies.
-
Assess Index Fragmentation: Over time, indexes can become fragmented, leading to decreased performance. The 'executionStats' section can help you identify if too many index keys are being scanned, which might suggest fragmentation. You can then run the
reIndex
command to rebuild the index. - Evaluate Query Performance: By comparing the 'executionTimeMillis' and the number of documents examined ('totalDocsExamined') before and after index changes, you can assess the impact of your index optimizations.
By using the explain plan in these ways, you can effectively identify and resolve index-related issues, leading to significant performance improvements in your MongoDB queries.
The above is the detailed content of How do I optimize MongoDB queries using explain plans?. For more information, please follow other related articles on the PHP Chinese website!

Hot AI Tools

Undresser.AI Undress
AI-powered app for creating realistic nude photos

AI Clothes Remover
Online AI tool for removing clothes from photos.

Undress AI Tool
Undress images for free

Clothoff.io
AI clothes remover

Video Face Swap
Swap faces in any video effortlessly with our completely free AI face swap tool!

Hot Article

Hot Tools

Notepad++7.3.1
Easy-to-use and free code editor

SublimeText3 Chinese version
Chinese version, very easy to use

Zend Studio 13.0.1
Powerful PHP integrated development environment

Dreamweaver CS6
Visual web development tools

SublimeText3 Mac version
God-level code editing software (SublimeText3)

Hot Topics
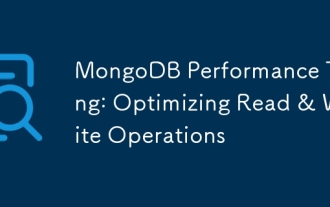
The core strategies of MongoDB performance tuning include: 1) creating and using indexes, 2) optimizing queries, and 3) adjusting hardware configuration. Through these methods, the read and write performance of the database can be significantly improved, response time, and throughput can be improved, thereby optimizing the user experience.
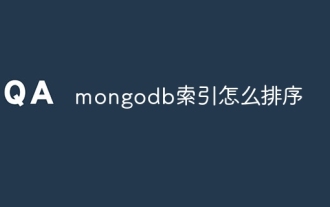
Sorting index is a type of MongoDB index that allows sorting documents in a collection by specific fields. Creating a sort index allows you to quickly sort query results without additional sorting operations. Advantages include quick sorting, override queries, and on-demand sorting. The syntax is db.collection.createIndex({ field: <sort order> }), where <sort order> is 1 (ascending order) or -1 (descending order). You can also create multi-field sorting indexes that sort multiple fields.
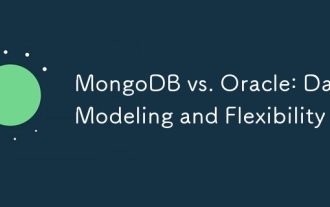
MongoDB is more suitable for processing unstructured data and rapid iteration, while Oracle is more suitable for scenarios that require strict data consistency and complex queries. 1.MongoDB's document model is flexible and suitable for handling complex data structures. 2. Oracle's relationship model is strict to ensure data consistency and complex query performance.
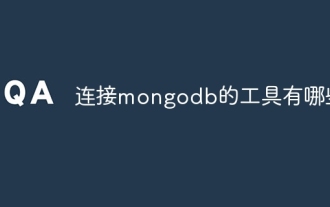
The main tools for connecting to MongoDB are: 1. MongoDB Shell, suitable for quickly viewing data and performing simple operations; 2. Programming language drivers (such as PyMongo, MongoDB Java Driver, MongoDB Node.js Driver), suitable for application development, but you need to master the usage methods; 3. GUI tools (such as Robo 3T, Compass) provide a graphical interface for beginners and quick data viewing. When selecting tools, you need to consider application scenarios and technology stacks, and pay attention to connection string configuration, permission management and performance optimization, such as using connection pools and indexes.
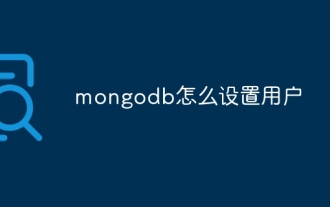
To set up a MongoDB user, follow these steps: 1. Connect to the server and create an administrator user. 2. Create a database to grant users access. 3. Use the createUser command to create a user and specify their role and database access rights. 4. Use the getUsers command to check the created user. 5. Optionally set other permissions or grant users permissions to a specific collection.
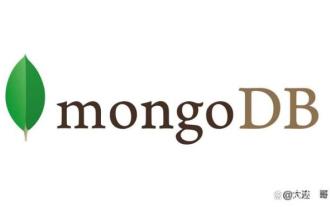
This article explains the advanced MongoDB query skills, the core of which lies in mastering query operators. 1. Use $and, $or, and $not combination conditions; 2. Use $gt, $lt, $gte, and $lte for numerical comparison; 3. $regex is used for regular expression matching; 4. $in and $nin match array elements; 5. $exists determine whether the field exists; 6. $elemMatch query nested documents; 7. Aggregation Pipeline is used for more powerful data processing. Only by proficiently using these operators and techniques and paying attention to index design and performance optimization can you conduct MongoDB data queries efficiently.
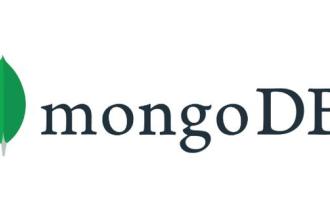
Choosing MongoDB or relational database depends on application requirements. 1. Relational databases (such as MySQL) are suitable for applications that require high data integrity and consistency and fixed data structures, such as banking systems; 2. NoSQL databases such as MongoDB are suitable for processing massive, unstructured or semi-structured data and have low requirements for data consistency, such as social media platforms. The final choice needs to weigh the pros and cons and decide based on the actual situation. There is no perfect database, only the most suitable database.
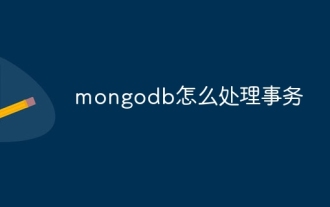
Transaction processing in MongoDB provides solutions such as multi-document transactions, snapshot isolation, and external transaction managers to achieve transaction behavior, ensure multiple operations are executed as one atomic unit, ensuring atomicity and isolation. Suitable for applications that need to ensure data integrity, prevent concurrent operational data corruption, or implement atomic updates in distributed systems. However, its transaction processing capabilities are limited and are only suitable for a single database instance. Multi-document transactions only support read and write operations. Snapshot isolation does not provide atomic guarantees. Integrating external transaction managers may also require additional development work.
