


Pandas vs. PySpark: A Java Developer's Guide to Data Processing
Pandas vs. PySpark: A Java Developer’s Guide to Data Processing
This article aims to guide Java developers in understanding and choosing between Pandas and PySpark for data processing tasks. We'll explore their differences, learning curves, and performance implications.
Understanding the Key Differences in Syntax and Functionality
Pandas and PySpark, while both used for data manipulation, operate in fundamentally different ways and target different scales of data. Pandas, a Python library, works with data in memory. It uses DataFrames, which are similar to tables in SQL databases, offering powerful functionalities for data cleaning, transformation, and analysis. Its syntax is concise and intuitive, often resembling SQL or R. Operations are performed on the entire DataFrame in memory, making it efficient for smaller datasets.
PySpark, on the other hand, is built on top of Apache Spark, a distributed computing framework. It also utilizes DataFrames, but these are distributed across a cluster of machines. This allows PySpark to handle datasets far larger than what Pandas can manage. While PySpark's DataFrame API shares some similarities with Pandas, its syntax often involves more explicit specification of distributed operations, including data partitioning and shuffling. This is necessary to coordinate processing across multiple machines. For example, a simple Pandas groupby()
operation translates into a more complex series of Spark transformations like groupBy()
followed by agg()
in PySpark. Furthermore, PySpark offers functionalities tailored for distributed processing, such as handling fault tolerance and scaling across a cluster.
Leveraging Existing Java Skills for Pandas or PySpark
A Java developer possesses several skills directly transferable to both Pandas and PySpark. Understanding object-oriented programming (OOP) principles is crucial for both. Java's strong emphasis on data structures translates well to understanding Pandas DataFrames and PySpark's DataFrame schema. Experience with data manipulation in Java (e.g., using collections or streams) directly relates to the transformations applied in Pandas and PySpark.
For Pandas, the learning curve is relatively gentle for Java developers. The Python syntax is easier to grasp than some other languages, and the core concepts of data manipulation are largely consistent. Focusing on mastering NumPy (a foundational library for Pandas) will be particularly beneficial.
For PySpark, the initial learning curve is steeper due to the distributed computing aspect. However, Java developers' experience with multithreading and concurrency will prove advantageous in understanding how PySpark manages tasks across a cluster. Familiarizing oneself with Spark's concepts, such as RDDs (Resilient Distributed Datasets) and transformations/actions, is key. Understanding the limitations and advantages of distributed computation is essential.
Performance Implications: Pandas vs. PySpark
The choice between Pandas and PySpark hinges significantly on data size and processing requirements. Pandas excels with smaller datasets that comfortably fit within the available memory of a single machine. Its in-memory operations are generally faster than the overhead of distributed processing in PySpark for such scenarios. For data manipulation tasks involving complex calculations or iterative processing on relatively small datasets, Pandas offers a more straightforward and often faster solution.
PySpark, however, is designed for massive datasets that exceed the capacity of a single machine's memory. Its distributed nature allows it to handle terabytes or even petabytes of data. While the overhead of distributing data and coordinating tasks introduces latency, this is far outweighed by the ability to process datasets that are impossible to handle with Pandas. For large-scale data processing tasks like ETL (Extract, Transform, Load), machine learning on big data, and real-time analytics on streaming data, PySpark is the clear winner in terms of scalability and performance. However, for smaller datasets, the overhead of PySpark can negate any performance gains compared to Pandas. Therefore, careful consideration of data size and task complexity is vital when choosing between the two.
The above is the detailed content of Pandas vs. PySpark: A Java Developer's Guide to Data Processing. For more information, please follow other related articles on the PHP Chinese website!

Hot AI Tools

Undresser.AI Undress
AI-powered app for creating realistic nude photos

AI Clothes Remover
Online AI tool for removing clothes from photos.

Undress AI Tool
Undress images for free

Clothoff.io
AI clothes remover

Video Face Swap
Swap faces in any video effortlessly with our completely free AI face swap tool!

Hot Article

Hot Tools

Notepad++7.3.1
Easy-to-use and free code editor

SublimeText3 Chinese version
Chinese version, very easy to use

Zend Studio 13.0.1
Powerful PHP integrated development environment

Dreamweaver CS6
Visual web development tools

SublimeText3 Mac version
God-level code editing software (SublimeText3)

Hot Topics
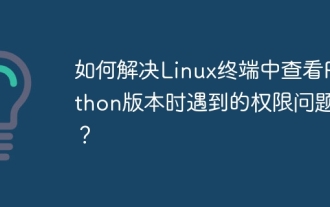
Solution to permission issues when viewing Python version in Linux terminal When you try to view Python version in Linux terminal, enter python...
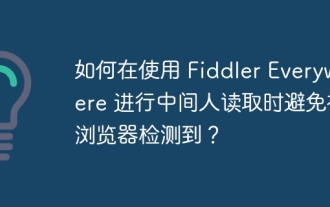
How to avoid being detected when using FiddlerEverywhere for man-in-the-middle readings When you use FiddlerEverywhere...
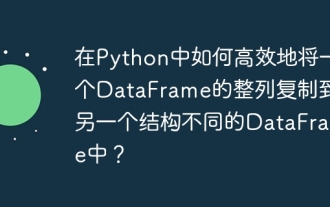
When using Python's pandas library, how to copy whole columns between two DataFrames with different structures is a common problem. Suppose we have two Dats...
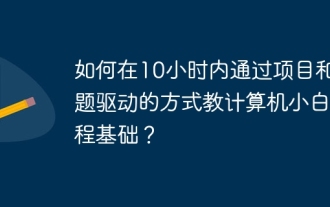
How to teach computer novice programming basics within 10 hours? If you only have 10 hours to teach computer novice some programming knowledge, what would you choose to teach...
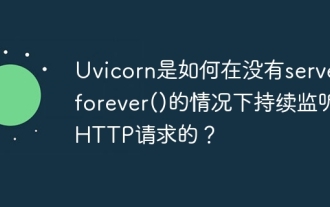
How does Uvicorn continuously listen for HTTP requests? Uvicorn is a lightweight web server based on ASGI. One of its core functions is to listen for HTTP requests and proceed...
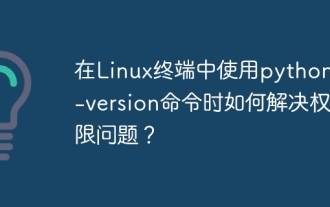
Using python in Linux terminal...
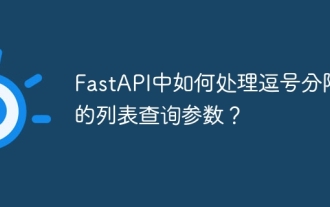
Fastapi ...
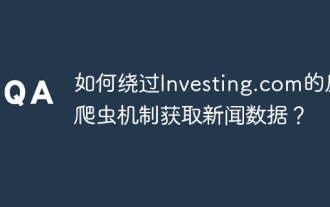
Understanding the anti-crawling strategy of Investing.com Many people often try to crawl news data from Investing.com (https://cn.investing.com/news/latest-news)...
