


How Do Time Steps and Features Affect LSTM Model Performance and Stateful Training?
Understanding LSTM Time Steps and Features
In LSTM models, time steps and features refer to the dimensions of the input data. Time steps represent the number of data points in a sequence, while features represent the different variables or dimensions within each data point.
In your example, the input data is reshaped into a 3D array with the following dimensions:
- Samples (batch size)
- Time steps (length of the sequences, in this case 3)
- Features (number of input variables, in this case 1)
Therefore, each sample is a sequence of 3 data points, with each data point consisting of a single input variable.
Stateful LSTM
Stateful LSTMs maintain a hidden state that is updated at each time step. This allows the model to learn from past inputs and make predictions based on a context. When stateful=True, the LSTM will remember the hidden state between batches, which can be useful for sequential data.
In your code, you are using a batch size of 1 and training the model for 100 epochs. However, you are also resetting the states after each epoch with model.reset_states(). This means that the model is not actually learning from past inputs between batches, and it is essentially treating each batch as a new sequence.
To correctly train a stateful LSTM, you should avoid resetting the states during training. Instead, you should only reset the states when you want to start a new sequence or make a prediction on a new data point.
Unrolled Diagrams
The diagrams you provided illustrate the unrolled architecture of LSTM networks. In both cases, the red boxes represent input steps, and the green boxes represent the hidden state.
Edit 1:
The following diagram corresponds to the first unrolled diagram you provided:
[Image of unrolled diagram with one input step per time step]
The diagram you provided corresponds to the second unrolled diagram:
[Image of unrolled diagram with all input steps at once]
Edit 2:
Understanding time steps and feature arguments is essential for LSTM models. Refer to the resources provided in the original post and comments for further clarification.
Additional Notes:
- LSTM layers can process data in various forms, including one-to-many, many-to-one, and many-to-many configurations.
- You can achieve different configurations by adjusting the return_sequences argument.
- The original post also contains valuable information on using stateful LSTMs for future step prediction tasks.
The above is the detailed content of How Do Time Steps and Features Affect LSTM Model Performance and Stateful Training?. For more information, please follow other related articles on the PHP Chinese website!

Hot AI Tools

Undresser.AI Undress
AI-powered app for creating realistic nude photos

AI Clothes Remover
Online AI tool for removing clothes from photos.

Undress AI Tool
Undress images for free

Clothoff.io
AI clothes remover

Video Face Swap
Swap faces in any video effortlessly with our completely free AI face swap tool!

Hot Article

Hot Tools

Notepad++7.3.1
Easy-to-use and free code editor

SublimeText3 Chinese version
Chinese version, very easy to use

Zend Studio 13.0.1
Powerful PHP integrated development environment

Dreamweaver CS6
Visual web development tools

SublimeText3 Mac version
God-level code editing software (SublimeText3)

Hot Topics
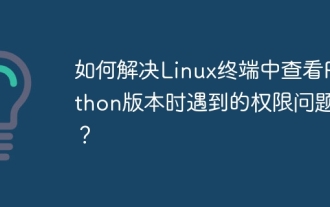
Solution to permission issues when viewing Python version in Linux terminal When you try to view Python version in Linux terminal, enter python...
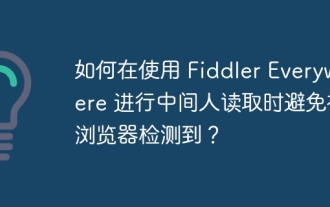
How to avoid being detected when using FiddlerEverywhere for man-in-the-middle readings When you use FiddlerEverywhere...
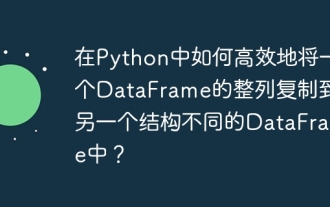
When using Python's pandas library, how to copy whole columns between two DataFrames with different structures is a common problem. Suppose we have two Dats...
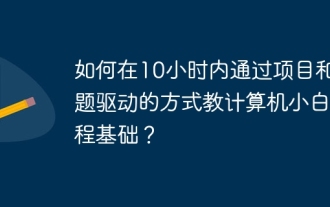
How to teach computer novice programming basics within 10 hours? If you only have 10 hours to teach computer novice some programming knowledge, what would you choose to teach...
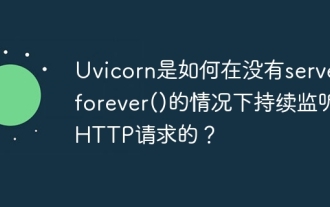
How does Uvicorn continuously listen for HTTP requests? Uvicorn is a lightweight web server based on ASGI. One of its core functions is to listen for HTTP requests and proceed...
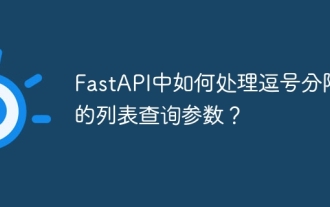
Fastapi ...
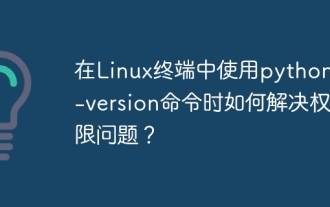
Using python in Linux terminal...
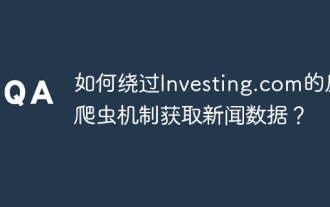
Understanding the anti-crawling strategy of Investing.com Many people often try to crawl news data from Investing.com (https://cn.investing.com/news/latest-news)...
