HBase数据迁移(3)-自己编写MapReduce Job导入数据
英文原文摘自:《HBase Administration Cookbook》??编译:ImportNew?-?陈晨 本篇文章是对数据合并的系列文章之三(共三篇),针对的情景模式就是将现有的各种类型的数据库或数据文件中的数据转入至 HBase 中。 系列之一 ???《HBase数据迁移(1)- 通过单个
英文原文摘自:《HBase Administration Cookbook》??编译:ImportNew?-?陈晨
本篇文章是对数据合并的系列文章之三(共三篇),针对的情景模式就是将现有的各种类型的数据库或数据文件中的数据转入至HBase中。
系列之一 ???《HBase数据迁移(1)- 通过单个客户端导入MySQL数据》
系列之二 ???《HBase数据迁移(1)- 使用bulk load 工具从TSV文件中导入数据》
尽管在将文本文件加载入HBase时importtsv工具十分高效,但在许多情况下为了完全控制整个加载过程,你可能更想自己编写MapReduce?Job向HBase导入数据。例如在你希望加载其他格式文件时不能使用importtsv工具。
HBase提供TableOutputFormat?用于在MapReduce?Job中向HBase的表中写入数据。你也可以使用HFileOutputFormat?类在MapReduce?Job中直接生成HBase自有格式文件HFile,之后使用上一篇(迁移2)中提到的completebulkload?工具加载至运行的HBase集群中。在本文中,我们将详细解释如何编写自己的MapReduce?Job来加载数据。我们会先介绍如何使用TableOutputFormat,在更多章节中介绍在MapReduce?Job中直接生成HBase自有格式文件HFile。
准备
我们本文中使用?“美国国家海洋和大气管理局?1981-2010气候平均值”的公共数据集合。访问http://www1.ncdc.noaa.gov/pub/data/normals/1981-2010/。?在目录?products?|?hourly?下的小时温度数据(可以在上述链接页面中找到)。下载hly-temp-normal.txt文件。对于下载的数据文件无需进行格式处理,我们将使用MapReduce直接读取原始数据。
我们假设您的环境已经可以在HBase上运行MapReduce。若还不行,你可以参考一下之前的文章(迁移1、迁移2)。
如何实施
1.将原始数据从本地文件系统拷贝进HDFS:
hac@client1$ $HADOOP_HOME/bin/hadoop fs -mkdir /user/hac/input/2-3 hac@client1$ $HADOOP_HOME/bin/hadoop fs -copyFromLocal hly-temp-normal.tsv /user/hac/input/2-3
2.编辑客户端服务器上的hadoop-env.sh文件,将HBase的JAR文件加入Hadoop的环境变量中:
hadoop@client1$ vi $HADOOP_HOME/conf/hadoop-env.sh export HADOOP_CLASSPATH=/usr/local/hbase/current/hbase-0.92.1.jar
3.编写MapReduce的Java代码并且打包为JAR文件。Java源码如下:
$ vi Recipe3.java
public class Recipe3 { public static Job createSubmittableJob (Configuration conf, String[] args) throws IOException { String tableName = args[0]; Path inputDir = new Path(args[1]); Job job = new Job (conf, "hac_chapter2_recipe3"); job.setJarByClass(HourlyImporter.class); FileInputFormat.setInputPaths(job, inputDir); job.setInputFormatClass(TextInputFormat.class); job.setMapperClass(HourlyImporter.class); // ++++ insert into table directly using TableOutputFormat ++++ // ++++ 使用TableOutputFormat 直接插入表中++++ TableMapReduceUtil.initTableReducerJob(tableName, null, job); job.setNumReduceTasks(0); TableMapReduceUtil.addDependencyJars(job); return job; } public static void main(String[] args) throws Exception { Configuration conf = HBaseConfiguration.create(); Job job = createSubmittableJob(conf, args); System.exit (job.waitForCompletion(true) ? 0 : 1); } }
4.在Recipe3.java中添加一个内部类。作为MapReduce?Job的mapper类:
$ vi Recipe3.java
static class HourlyImporter extends Mapper<longwritable text immutablebyteswritable put> { private long ts; static byte[] family = Bytes.toBytes("n"); @Override protected void setup(Context context) { ts = System.currentTimeMillis(); } @Override public void map(LongWritable offset, Text value, Context context)throws IOException { try { String line = value.toString(); String stationID = line.substring(0, 11); String month = line.substring(12, 14); String day = line.substring(15, 17); String rowkey = stationID + month + day; byte[] bRowKey = Bytes.toBytes(rowkey); ImmutableBytesWritable rowKey = new ImmutableBytesWritable(bRowKey); Put p = new Put(bRowKey); for (int i = 1; i <p>5.<span style="font-family: 宋体;">为了能够运行</span><span style="font-family: 'Times New Roman';">MapReduce?Job</span><span style="font-family: 宋体;">需要将源码打包为</span><span style="font-family: 'Times New Roman';">JAR</span><span style="font-family: 宋体;">文件,并且从客户端使用</span><span style="font-family: 'Times New Roman';">hadoop?jar</span><span style="font-family: 宋体;">命令:</span></p> <pre class="brush:php;toolbar:false">hac@client1$ $HADOOP_HOME/bin/hadoop jar hac-chapter2.jar hac. chapter2.Recipe3 \ hly_temp \ /user/hac/input/2-3
检查结果。MapReduce?job的运行结果应当显示下内容:
13/03/27 17:42:40 INFO mapred.JobClient: Map-Reduce Framework 13/03/27 17:42:40 INFO mapred.JobClient: Map input records=95630 13/03/27 17:42:40 INFO mapred.JobClient: Physical memory (bytes) snapshot=239820800 13/03/27 17:42:40 INFO mapred.JobClient: Spilled Records=0 13/03/27 17:42:40 INFO mapred.JobClient: CPU time spent (ms)=124530 13/03/27 17:42:40 INFO mapred.JobClient: Total committed heap usage (bytes)=130220032 13/03/27 17:42:40 INFO mapred.JobClient: Virtual memory (bytes) snapshot=1132621824 13/03/27 17:42:40 INFO mapred.JobClient: Map input bytes=69176670 13/03/27 17:42:40 INFO mapred.JobClient: Map output records=95630 13/03/27 17:42:40 INFO mapred.JobClient: SPLIT_RAW_BYTES=118
Map的输入记录数应当与输入路径下的文件内容总行数相同。Map输出记录数应当与输入记录数相同(本文中)。你能够在HBase中使用?count/scan命令来验证上述结果
运行原理
为了运行MapReduce?Job,我们首先在createSubmittableJob()方法中构建一个Job实例。实例建立后,我们对其设置了输入路径,输入格式以及mapper类。之后,我们调用了TableMapReduceUtil.initTableReducerJob()?对job进行适当配置。包括,加入HBase配置,设置TableOutputFormat,以及job运行需要的一些依赖的添加。在HBase上编写MapReduce程序时,TableMapReduceUtil?是一个很有用的工具类。
主函数中调用?job.waitForCompletion()?能够将Job提交到MapReduce框架中,直到运行完成才退出。运行的Job将会读取输入路径下的所有文件,并且将每行都传入到mapper类(HourlyImporter)。
在map方法中,转换行数据并生成row?key,建立Put对象,通过Put.add()方法将转换后的数据添加到对应的列中。最终调用context.write()方法将数据写入HBase表中。本例中无需reduce阶段。
如你所见,编写自定义的MapReduce?Job来向HBase插入数据是很简单的。程序与直接在单台客户端使用HBase?API类似。当面对海量数据时,我们建议使用MapReduce来向HBase中导入数据。
其他
使用自定义的MapReduce?Job来向HBase加载数据在大部分情况下都是合理的。但是,如果你的数据是极大量级的,上述方案不能很好处理时。还有其他方式能够更好的处理数据合并问题。
在MapReduce中生成HFile
除了直接将数据写入HBase表,我们还可以在MapReduce?Job中直接生成HBase自有格式HFile,然后使用completebulkload?工具将文件加载进集群中。这个方案将比使用TableOutputFormat?API更加节省CPU与网络资源:
1.修改Job配置。要生成HFile文件,找到createSubmittableJob()的下面两行:
TableMapReduceUtil.initTableReducerJob(tableName, null, job); job.setNumReduceTasks(0);
2.替换代码
HTable table = new HTable(conf, tableName); job.setReducerClass(PutSortReducer.class); Path outputDir = new Path(args[2]); FileOutputFormat.setOutputPath(job, outputDir); job.setMapOutputKeyClass(ImmutableBytesWritable.class); job.setMapOutputValueClass(Put.class); HFileOutputFormat.configureIncrementalLoad (job, table);
3.在命令行添加输出地址参数。编译并打包源码,然后在运行任务的命令行添加输出地址参数:
hac@client1$ $HADOOP_HOME/bin/hadoop jar hac-chapter2.jar hac. chapter2.Recipe3 \ hly_temp \ /user/hac/input/2-3 \ /user/hac/output/2-3
4.完成bulk?load:
hac@client1$ $HADOOP_HOME/bin/hadoop jar $HBASE_HOME/hbase- 0.92.1.jar completebulkload \ /user/hac/output/2-3 \ hly_temp
步骤1中,我们修改了源码中的job配置。我们设置job使用由HBase提供的PutSortReducer??reduce类。这个类会在数据行写入之前对列进行整理。HFileOutputFormat.configureIncrementalLoad()?方法能够为生成HFile文件设置适当的参数。
在步骤2中的job运行完成之后,自有HFile格式文件会生成在我们指定的输出路径。文件在列族目录2-3/n之下,将会使用completebulkload?加载到HBase集群中。
在MapReduce?Job执行过程中,如果你在浏览器中打开HBase的管理界面,会发现HBase没有发出任何请求。这表明这些数据不是直接写入HBase的表中。
影响数据合并的重要配置
如果你在MapReduce?Job使用TableOutputFormat?类将数据直接写入HBase表中,是一个十分繁重的写操作。尽管HBase是设计用于快速处理写操作,但下面的这些还是你可能需要调整的重要的配置:
- JVM的堆栈和GC设置
- 域服务器处理数量
- ?最大的域文件数量
- ?内存大小
- ?更新块设置
你需要了解HBase架构的基本知识来理解这些配置如何影响HBase的写性能。以后我们会进行详细的描述。
Hadoop和HBase会生成若干日志。当集群中的MapReduce?Job加载数据时存在某些瓶颈或障碍时,检查日志可以给你一些提示。下面是一些比较重要的日志:
- ?Hadoop/HBase/ZooKeeper的守护进程的GC日志
- ?HMaster守护进程的日志
在将数据转移至HBase之前预先搭建域
HBase的每行数据都归属一个特定的域中。一个域中包含了一定范围内的排序号的HBase的数据行。域是由域服务器发布和管理的。
当我们在HBase中建立一个表后,该表会在一个单独的域启动。所有插入该表的数据都会首先进入这个域中。数据持续插入,当到达一个极限之后,域会被分为两份。称之为域的分离。分离的域会分布到其他域服务器上,以达到集群中的负载能够均衡。
如你所想,若我们能够将表初始化在预先建好的域上,使用合适的算法,数据加载的负载会在整个集群中平衡,并且加快了数据加载的速度。
我们将描述如何用预先建好的域来建立一个表。
准备
登入HBase的客户端节点
如何实施
在客户端节点上执行如下命令:
$ $HBASE_HOME/bin/hbase org.apache.hadoop.hbase.util.RegionSplitter -c 10 -f n hly_temp2 12/04/06 23:16:32 DEBUG util.RegionSplitter: Creating table hly_temp2 with 1 column families. Presplitting to 10 regions … 12/04/06 23:16:44 DEBUG util.RegionSplitter: Table created! Waiting for regions to show online in META... 12/04/06 23:16:44 DEBUG util.RegionSplitter: Finished creating table with 10 regions
运行原理
命令行调用了RegionSplitter?类,并且附带如下参数:
- ?-c?10—用预先分割的10个域来建立这个表
- ?-f?n—建立一个名叫n的列族
- ?hly_temp2—?表名
在浏览器中打开HBase管理界面,在用户表中点击hly_temp2,你可以看到预先建立的10个域。
RegionSplitter?是HBase提供的一个工具类。使用RegionSplitter?你可以做下面这些事情:
- ?使用具体数量的预建域来建立一个表。
- ?能够将一个已存在的表进行分离域。
- ?使用自定义算法来分离域。
在上文中使用自定义MapReduce导入数据时,也许你原本认为数据写入应该是分布在集群中所有的域中,但实际不是。在管理页上可以看到,在MapReduce?Job的执行期间所有的请求都发送至相同的服务器。
这是因为默认的分离算法(MD5StringSplit)不是很适合我们的情况。我们所有的数据都发送至相同集群,因此所有的API请求都发送至域所在的域服务器中。我们需要提供自定义的算法来适当的分离域。
预分离的域也能够对生成自有格式HFile文件的的MapReduce?Job产生影响。运行上文中的MapReduce?Job,对hly_temp2表使用生成HFile文件的选项。如下图所示,你可以发现MapReduce?Job的reduce数量从原本的1到10了,这就是预搭建域的数量:
这是因为Job中reduce的数量是基于目标表的域数量。
若reduce数量增加,通常意味加载动作分布到多个服务器上面,所以job的运行速度会更快。
英文原文摘自:《HBase Administration Cookbook》??编译:ImportNew?-?陈晨
译文链接:http://www.importnew.com/3645.html
【如需转载,请在正文中标注并保留原文链接、译文链接和译者等信息,谢谢合作!】
原文地址:HBase数据迁移(3)-自己编写MapReduce Job导入数据, 感谢原作者分享。

Hot AI Tools

Undresser.AI Undress
AI-powered app for creating realistic nude photos

AI Clothes Remover
Online AI tool for removing clothes from photos.

Undress AI Tool
Undress images for free

Clothoff.io
AI clothes remover

Video Face Swap
Swap faces in any video effortlessly with our completely free AI face swap tool!

Hot Article

Hot Tools

Notepad++7.3.1
Easy-to-use and free code editor

SublimeText3 Chinese version
Chinese version, very easy to use

Zend Studio 13.0.1
Powerful PHP integrated development environment

Dreamweaver CS6
Visual web development tools

SublimeText3 Mac version
God-level code editing software (SublimeText3)

Hot Topics
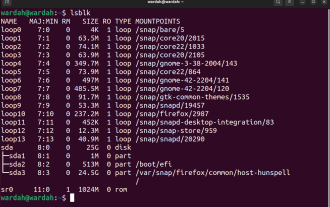
DDREASE is a tool for recovering data from file or block devices such as hard drives, SSDs, RAM disks, CDs, DVDs and USB storage devices. It copies data from one block device to another, leaving corrupted data blocks behind and moving only good data blocks. ddreasue is a powerful recovery tool that is fully automated as it does not require any interference during recovery operations. Additionally, thanks to the ddasue map file, it can be stopped and resumed at any time. Other key features of DDREASE are as follows: It does not overwrite recovered data but fills the gaps in case of iterative recovery. However, it can be truncated if the tool is instructed to do so explicitly. Recover data from multiple files or blocks to a single
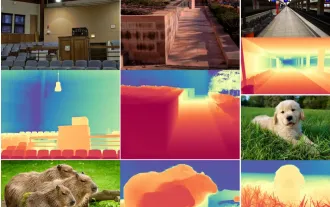
0.What does this article do? We propose DepthFM: a versatile and fast state-of-the-art generative monocular depth estimation model. In addition to traditional depth estimation tasks, DepthFM also demonstrates state-of-the-art capabilities in downstream tasks such as depth inpainting. DepthFM is efficient and can synthesize depth maps within a few inference steps. Let’s read about this work together ~ 1. Paper information title: DepthFM: FastMonocularDepthEstimationwithFlowMatching Author: MingGui, JohannesS.Fischer, UlrichPrestel, PingchuanMa, Dmytr
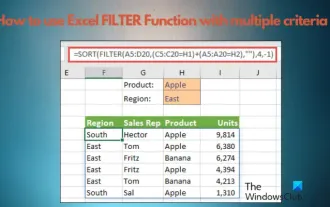
If you need to know how to use filtering with multiple criteria in Excel, the following tutorial will guide you through the steps to ensure you can filter and sort your data effectively. Excel's filtering function is very powerful and can help you extract the information you need from large amounts of data. This function can filter data according to the conditions you set and display only the parts that meet the conditions, making data management more efficient. By using the filter function, you can quickly find target data, saving time in finding and organizing data. This function can not only be applied to simple data lists, but can also be filtered based on multiple conditions to help you locate the information you need more accurately. Overall, Excel’s filtering function is a very practical
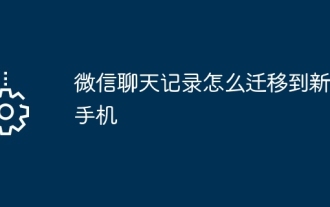
1. Open the WeChat app on the old device, click [Me] in the lower right corner, select the [Settings] function, and click [Chat]. 2. Select [Chat History Migration and Backup], click [Migrate], and select the platform to which you want to migrate the device. 3. Click [Select chats to be migrated], click [Select all] in the lower left corner, or select chat records yourself. 4. After selecting, click [Start] in the lower right corner to log in to this WeChat account using the new device. 5. Then scan the QR code to start migrating chat records. Users only need to wait for the migration to complete.
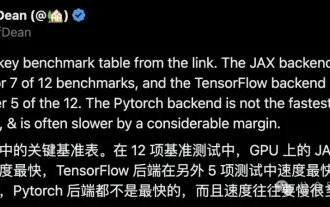
The performance of JAX, promoted by Google, has surpassed that of Pytorch and TensorFlow in recent benchmark tests, ranking first in 7 indicators. And the test was not done on the TPU with the best JAX performance. Although among developers, Pytorch is still more popular than Tensorflow. But in the future, perhaps more large models will be trained and run based on the JAX platform. Models Recently, the Keras team benchmarked three backends (TensorFlow, JAX, PyTorch) with the native PyTorch implementation and Keras2 with TensorFlow. First, they select a set of mainstream
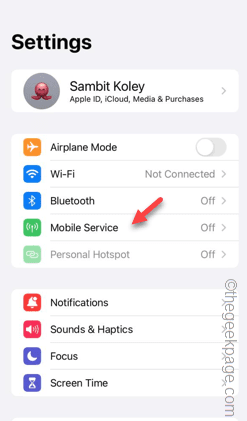
Facing lag, slow mobile data connection on iPhone? Typically, the strength of cellular internet on your phone depends on several factors such as region, cellular network type, roaming type, etc. There are some things you can do to get a faster, more reliable cellular Internet connection. Fix 1 – Force Restart iPhone Sometimes, force restarting your device just resets a lot of things, including the cellular connection. Step 1 – Just press the volume up key once and release. Next, press the Volume Down key and release it again. Step 2 – The next part of the process is to hold the button on the right side. Let the iPhone finish restarting. Enable cellular data and check network speed. Check again Fix 2 – Change data mode While 5G offers better network speeds, it works better when the signal is weaker
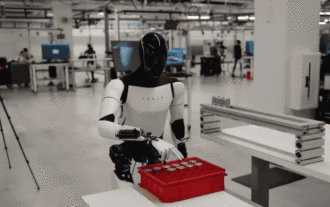
The latest video of Tesla's robot Optimus is released, and it can already work in the factory. At normal speed, it sorts batteries (Tesla's 4680 batteries) like this: The official also released what it looks like at 20x speed - on a small "workstation", picking and picking and picking: This time it is released One of the highlights of the video is that Optimus completes this work in the factory, completely autonomously, without human intervention throughout the process. And from the perspective of Optimus, it can also pick up and place the crooked battery, focusing on automatic error correction: Regarding Optimus's hand, NVIDIA scientist Jim Fan gave a high evaluation: Optimus's hand is the world's five-fingered robot. One of the most dexterous. Its hands are not only tactile
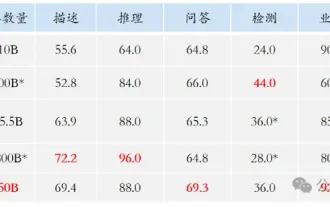
I cry to death. The world is madly building big models. The data on the Internet is not enough. It is not enough at all. The training model looks like "The Hunger Games", and AI researchers around the world are worrying about how to feed these data voracious eaters. This problem is particularly prominent in multi-modal tasks. At a time when nothing could be done, a start-up team from the Department of Renmin University of China used its own new model to become the first in China to make "model-generated data feed itself" a reality. Moreover, it is a two-pronged approach on the understanding side and the generation side. Both sides can generate high-quality, multi-modal new data and provide data feedback to the model itself. What is a model? Awaker 1.0, a large multi-modal model that just appeared on the Zhongguancun Forum. Who is the team? Sophon engine. Founded by Gao Yizhao, a doctoral student at Renmin University’s Hillhouse School of Artificial Intelligence.
