


In-depth understanding of Python collection module and deep and shallow copy
ccollection module is an extension of Python's general built-in containers: dictionary, list, tuple and set. It contains some professional containersdata types :
Counter (counter): dict subclass, used to count the number of hashable objects.
OrderedDict (ordered dictionary): dict subclass, recording the order in which data members are added.
defaultdict (default dictionary): dict subclass, calling a factory function to provide a default value for missing values of dict.
namedtuple (named tuple): The factory function generates a tuple subclass with named fields.
deque (two-way queue): A function that can quickly dequeue and join the queue at both ends of the "queue", similar to the queue (list-like) container.
ChainMap: A dictionary-like type that creates a single view for multiple maps.
UserDict: Wrapping a dictionary makes it easier to create subclasses of the dictionary.
UserList: Wrapping the list object makes it easier to create subclasses of the list.
UserString: Wrapping a String object makes it easier to create subclasses of String.
1. Counter (counter)
Counter is a subclass of dict, used to count hashable objects. It is an unordered container with elements stored as dictionary keys and count values as dictionary values. Count allows any integer value, including zero or negative counts. The Counter class is similar to language classes such as bags or multisets.
Its elements are counted from an iterable object, or initialized from another map (or counter).
1 2 3 4 5 6 7 8 9 10 11 12 13 14 15 16 17 18 19 20 21 22 23 24 25 26 27 28 29 30 31 32 33 34 35 36 37 38 39 40 41 42 43 44 45 46 47 48 49 50 51 52 53 54 55 56 57 58 59 60 61 62 63 64 65 66 67 68 69 70 71 72 73 74 75 76 77 78 79 80 81 82 83 84 85 86 87 88 89 90 91 92 93 94 95 96 97 98 99 100 101 102 103 104 105 106 107 108 109 110 111 112 113 114 115 116 117 118 119 120 121 122 123 124 125 126 127 128 129 130 131 132 133 134 135 136 137 138 139 140 141 142 143 144 145 146 147 148 149 150 151 152 153 154 155 156 157 158 159 160 161 162 163 164 165 166 167 168 169 170 171 172 173 174 175 176 177 178 179 180 181 182 183 184 185 186 187 188 189 190 191 192 193 194 195 196 197 198 199 200 201 202 203 204 205 206 207 208 209 210 211 212 213 214 215 216 217 218 219 220 221 222 223 224 225 226 227 228 229 230 231 232 233 234 235 236 237 238 239 240 241 242 243 244 245 246 247 248 249 250 251 252 253 254 255 256 257 258 259 260 261 262 263 264 265 266 267 268 269 270 271 272 273 274 275 276 277 278 279 280 281 282 283 284 285 286 287 288 289 290 291 292 293 294 295 296 297 298 299 300 301 302 303 304 305 306 307 308 309 310 311 312 313 314 315 316 317 318 319 320 321 322 323 324 325 326 327 328 329 330 331 332 333 334 335 336 337 338 339 340 341 342 343 344 345 346 347 348 349 350 351 352 353 354 355 356 357 358 359 360 361 362 363 364 365 366 367 368 369 370 371 372 373 374 375 376 377 378 379 380 381 382 383 |
|
1) Creation of counter:
1 2 3 4 5 6 |
|
2) Deletion of counter element
1 2 3 4 5 6 7 8 9 10 11 12 13 |
|
3) Partial functions of counterAttributes
most_common(self, n=None):
Convert the counter into a list, the elements into a tuple, and return a list of the n most common elements and their counts, starting from the most Common to least common. If n is omitted or None, most_common() returns all elements in the counter. Elements with equal counts are ordered arbitrarily.
1 2 3 4 5 6 7 8 9 10 11 12 13 |
|
elements(self):
Returns an iterator that iterates over the elements a count of times. Elements are returned in random order. If the element's count is less than 1, elements() will ignore it.
1 2 3 4 5 6 7 8 9 10 11 12 13 |
|
update(*args, **kwds):
Elements are counted from an iterable or incremented from another map (or counter). Like dict.update(), but increments counts instead of replacing them. Additionally, iterable objects should be a sequence of elements, not (key, value) pairs.
1 2 3 4 5 6 7 8 9 |
|
subtract(*args, **kwds):
Subtract elements from an iterable or another map (or counter). Like dict.update(), but subtracts counts instead of replacing them. Both input and output can be zero or negative.
1 2 3 4 5 6 7 8 |
|
2. Ordered Dictionary (OrderedDict)
Ordered dictionaries are similar to regular dictionaries, but they remember the order in which key-value pairs are inserted. When iterating over an ordered dictionary, items are returned in the order in which their keys were first added.
1 2 3 4 5 6 7 8 9 10 11 12 13 14 15 16 17 18 19 20 21 22 23 24 25 26 27 28 29 30 31 32 33 34 35 36 37 38 39 40 41 42 43 44 45 46 47 48 49 50 51 52 53 54 55 56 57 58 59 60 61 62 63 64 65 66 67 68 69 70 71 72 73 74 75 76 77 78 79 80 81 82 83 84 85 86 87 88 89 90 91 92 93 94 95 96 97 98 99 100 101 102 103 104 105 106 107 108 109 110 111 112 113 114 115 116 117 118 119 120 121 122 123 124 125 126 127 128 129 130 131 132 133 134 135 136 137 138 139 140 141 142 143 144 145 146 147 148 149 150 151 152 153 154 155 156 157 158 159 160 161 162 163 164 165 166 167 168 169 170 171 172 173 174 175 176 177 178 179 180 181 182 183 184 185 186 187 188 189 190 191 192 193 194 195 196 197 198 199 200 201 202 203 204 205 206 207 208 209 210 211 212 213 214 215 216 217 218 219 220 221 222 223 224 225 226 227 228 229 |
|
1) Creation of ordered dictionary:
1 2 3 4 5 6 7 8 9 10 11 12 13 14 15 16 17 18 19 20 |
|
2) Function of ordered dictionary:
Ordered dictionary inherits the function of dictionary , only the functions different from the dictionary are introduced below. popitem(self, last=True):
Return and delete key-value pairs in the dictionary. If last is True (the default), these key-value pairs are returned in LIFO order, if False, they are returned in FIFO order.
1 2 3 4 5 6 7 8 9 10 11 12 13 14 |
|
Move an existing key to the other end of the ordered dictionary. If last is True (the default), the item is moved to the end, if last is False, it is moved to the beginning. If key does not exist, KeyError is raised.
1 2 3 4 5 6 7 8 9 10 11 12 13 14 |
|
defaultdict can specify a default value for the dictionary, which can be a dictionary/list, etc. Returns a new dictionary-like object with the same functionality as the dict class.
Such as:
1 2 3 4 5 6 7 8 9 10 11 12 13 14 15 16 17 18 |
|
1 2 3 4 5 6 7 8 9 10 11 12 13 14 15 |
|
1 2 3 4 5 6 7 8 9 10 11 12 13 14 15 16 17 18 19 20 21 22 23 24 25 26 27 28 29 30 31 32 33 34 35 36 37 38 39 40 41 42 43 44 45 46 47 48 49 50 51 52 53 54 55 56 57 |
|
给元组中每个位置上的元素命名,它们可以使用常规的元组方法,可以让访问元素可以按名称而不是按位置索引。
collections.namedtuple(typename, field_names, verbose=False, rename=False):
返回一个叫做 typename 的tuple子类,这个新的子类用来创建类tuple(tuple-like)的对象,这个对象拥有可以通过属性访问的字段,并且可以通过下标索引和迭代。
field_names 是一个单独的字符串,这个字符串中包含的所有字段用空格或逗号隔开,例如 'xy' 或 'x,y'.另外, field_names 也可以是字符串的列表,例如 ['x', 'y']。
如果verbose 为 True, 在类被建立后将打印类的定义。相反,它打印的是类的 _source 属性,也就是打印源代码。
如果 rename参数 为 True, 无效的field_names会被自动转换成位置的名称.例如, ['abc', 'def', 'ghi', 'abc'] 将被转换为 ['abc', '_1', 'ghi', '_3'], 来消除关键字 def 和重复的字段名 abc。
2)可命名元组的创建
需要先创建一个类。
1 2 3 4 5 6 7 8 9 10 11 |
|
3)可命名元组新创建类的功能属性
如上面创建的myTupleCalss类:
1 2 3 4 5 6 7 8 9 10 11 12 13 14 15 16 17 18 19 20 21 22 23 24 25 26 27 28 29 30 31 32 33 34 35 36 37 38 39 40 41 42 43 44 45 46 47 48 49 50 51 52 53 54 55 56 57 58 59 60 61 62 63 64 65 66 67 68 69 70 71 72 73 74 75 76 77 78 79 80 81 82 83 84 85 86 87 88 89 90 91 92 93 94 95 96 97 98 99 100 101 102 103 104 105 106 107 108 109 110 |
|
5.队列(deque)
1)双向队列(deque)
双向队列(Deque)是栈和队列的一般化。可以在两端添加和删除元素。
双向队列的创建:
1 2 3 4 5 6 7 8 9 10 |
|
双向队列的功能属性:
1 2 3 4 5 6 7 8 9 10 11 12 13 14 15 16 17 18 19 20 21 22 23 24 25 26 27 28 29 30 31 32 33 34 35 36 37 38 39 40 41 42 43 44 45 46 47 48 49 50 51 52 53 54 55 56 57 58 59 60 61 62 63 64 65 66 67 68 69 70 71 72 73 74 75 76 77 78 79 80 81 82 83 84 85 86 87 88 89 90 91 92 93 94 95 96 97 98 99 100 101 102 103 104 105 106 107 108 109 110 111 112 113 114 115 116 117 118 119 120 121 122 123 124 125 126 127 128 129 130 131 132 133 134 135 136 137 138 139 140 141 142 143 144 145 146 147 148 149 150 151 152 153 154 155 156 157 158 159 160 161 162 163 164 165 166 167 168 169 170 171 172 173 174 175 176 177 178 179 180 181 182 |
|
2)单向队列(queue.Queue)
class queue.Queue(maxsize=0)
单向队列与双向队列的区别是FIFO(先进先出),maxsize是个整数,指明了队列中能存放的数据个数的上限。一旦达到上限,插入会导致阻塞,直到队列中的数据被取出。如果maxsize小于或者等于0,则队列大小没有限制。
单向队列的创建:
1 2 3 4 5 6 7 8 9 10 |
|
单向队列的功能属性:
1 2 3 4 5 6 7 8 9 10 11 12 13 14 15 16 17 18 19 20 21 22 23 24 25 26 27 28 29 30 31 32 33 34 35 36 37 38 39 40 41 42 43 44 45 46 47 48 49 50 51 52 53 54 55 56 57 58 59 60 61 62 63 64 65 66 67 68 69 70 71 72 73 74 75 76 77 78 79 80 81 82 83 84 85 86 87 88 89 90 91 92 93 94 95 96 97 98 99 100 101 102 103 104 105 106 107 108 109 110 111 112 113 114 115 116 117 118 119 120 121 122 123 124 125 126 127 128 129 130 131 132 133 134 135 136 137 138 139 140 141 142 143 144 145 146 147 148 149 150 151 152 153 154 155 156 157 158 159 160 161 162 163 164 165 166 167 168 169 170 171 172 173 174 175 176 177 178 179 180 181 182 183 184 185 186 187 188 189 190 191 192 193 |
|
6.深浅拷贝
浅拷贝和深拷贝的主要区别在与操作后内存地址的变化是不同的。
The above is the detailed content of In-depth understanding of Python collection module and deep and shallow copy. For more information, please follow other related articles on the PHP Chinese website!

Hot AI Tools

Undresser.AI Undress
AI-powered app for creating realistic nude photos

AI Clothes Remover
Online AI tool for removing clothes from photos.

Undress AI Tool
Undress images for free

Clothoff.io
AI clothes remover

Video Face Swap
Swap faces in any video effortlessly with our completely free AI face swap tool!

Hot Article

Hot Tools

Notepad++7.3.1
Easy-to-use and free code editor

SublimeText3 Chinese version
Chinese version, very easy to use

Zend Studio 13.0.1
Powerful PHP integrated development environment

Dreamweaver CS6
Visual web development tools

SublimeText3 Mac version
God-level code editing software (SublimeText3)

Hot Topics










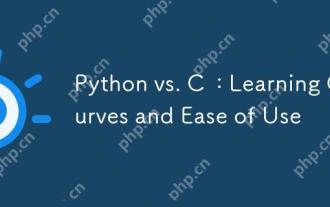
Python is easier to learn and use, while C is more powerful but complex. 1. Python syntax is concise and suitable for beginners. Dynamic typing and automatic memory management make it easy to use, but may cause runtime errors. 2.C provides low-level control and advanced features, suitable for high-performance applications, but has a high learning threshold and requires manual memory and type safety management.
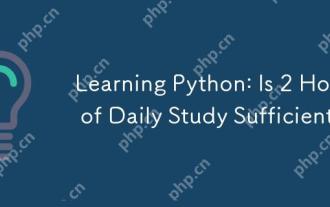
Is it enough to learn Python for two hours a day? It depends on your goals and learning methods. 1) Develop a clear learning plan, 2) Select appropriate learning resources and methods, 3) Practice and review and consolidate hands-on practice and review and consolidate, and you can gradually master the basic knowledge and advanced functions of Python during this period.
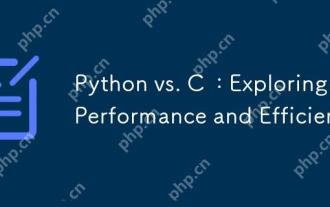
Python is better than C in development efficiency, but C is higher in execution performance. 1. Python's concise syntax and rich libraries improve development efficiency. 2.C's compilation-type characteristics and hardware control improve execution performance. When making a choice, you need to weigh the development speed and execution efficiency based on project needs.
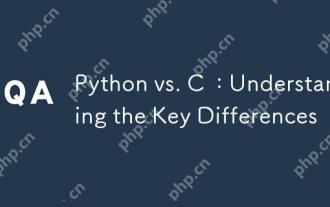
Python and C each have their own advantages, and the choice should be based on project requirements. 1) Python is suitable for rapid development and data processing due to its concise syntax and dynamic typing. 2)C is suitable for high performance and system programming due to its static typing and manual memory management.
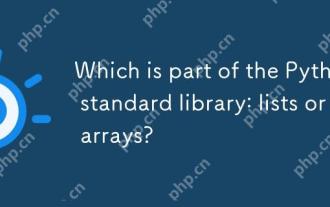
Pythonlistsarepartofthestandardlibrary,whilearraysarenot.Listsarebuilt-in,versatile,andusedforstoringcollections,whereasarraysareprovidedbythearraymoduleandlesscommonlyusedduetolimitedfunctionality.
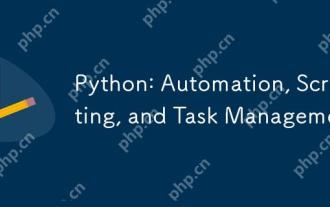
Python excels in automation, scripting, and task management. 1) Automation: File backup is realized through standard libraries such as os and shutil. 2) Script writing: Use the psutil library to monitor system resources. 3) Task management: Use the schedule library to schedule tasks. Python's ease of use and rich library support makes it the preferred tool in these areas.
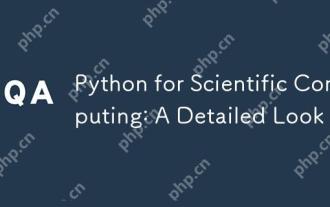
Python's applications in scientific computing include data analysis, machine learning, numerical simulation and visualization. 1.Numpy provides efficient multi-dimensional arrays and mathematical functions. 2. SciPy extends Numpy functionality and provides optimization and linear algebra tools. 3. Pandas is used for data processing and analysis. 4.Matplotlib is used to generate various graphs and visual results.
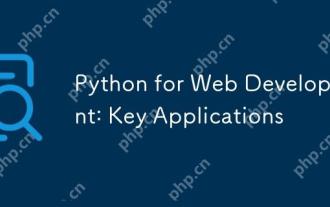
Key applications of Python in web development include the use of Django and Flask frameworks, API development, data analysis and visualization, machine learning and AI, and performance optimization. 1. Django and Flask framework: Django is suitable for rapid development of complex applications, and Flask is suitable for small or highly customized projects. 2. API development: Use Flask or DjangoRESTFramework to build RESTfulAPI. 3. Data analysis and visualization: Use Python to process data and display it through the web interface. 4. Machine Learning and AI: Python is used to build intelligent web applications. 5. Performance optimization: optimized through asynchronous programming, caching and code
