


Efficiency increased by 16 times! VRSO: 3D annotation of purely visual static objects, opening up the data closed loop!
The Sorrow of Annotation
Static object detection (SOD), including traffic lights, guide signs and traffic cones, most algorithms are data-driven deep neural networks and require a large amount of training data. Current practice typically involves manual annotation of large numbers of training samples on LiDAR-scanned point cloud data to fix long-tail cases.
Manual annotation is difficult to capture the variability and complexity of real scenes, and often fails to take into account occlusions, different lighting conditions and diverse viewing angles (yellow arrow in Figure 1) . The whole process has long links, is extremely time-consuming, error-prone, and costly (Figure 2). So currently companies are looking for automatic labeling solutions, especially based on pure vision. After all, not every car has lidar.
VRSO is a vision-based annotation system for static object annotation. It mainly uses information from SFM, 2D object detection and instance segmentation results. Overall effect: The average projection error of
- annotations is only 2.6 pixels, which is about a quarter of Waymo annotations (10.6 pixels)
- Compared with manual annotation, the speed is improved About 16 times
For static objects, VRSO solves the challenge of integrating and deduplicating static objects from different viewing angles through instance segmentation and contour extraction of key points, as well as the difficulty of insufficient observation due to occlusion issues , thus improving the accuracy of labeling. From Figure 1, compared with the manual annotation results of the Waymo Open dataset, VRSO demonstrates higher robustness and geometric accuracy.
(You’ve all seen this, why not slide your thumb up and click on the top card to follow me. The whole operation will only take you 1.328 seconds, and then take away all the useful information in the future. What if it works~)
How to break the situation
The VRSO system is mainly divided into two parts: Scene reconstruction and Static objects are marked .
#The reconstruction part is not the focus, it is based on the SFM algorithm to restore the image pose and sparse 3D key points.
Static object annotation algorithm, combined with pseudo code, the general process is (the following will be detailed step by step):
- Use ready-made 2D object detection and segmentation algorithms to generate candidates
- Use the 3D-2D keypoint correspondence in the SFM model to track 2D instances across frames
- Introduce reprojection consistency to optimize the 3D annotation parameters of static objects
1. Tracking association
- #step 1: Extract 3D points within the 3D bounding box based on the key points of the SFM model.
- step 2: Calculate the coordinates of each 3D point on the 2D map based on the 2D-3D matching relationship.
- step 3: Determine the corresponding instance of the 3D point on the current 2D map based on the 2D map coordinates and instance segmentation corner points.
- step 4: Determine the correspondence between 2D observations and 3D bounding boxes for each 2D image.
2.proposal generation
Initialize the 3D frame parameters (position, direction, size) of the static object for the entire video clip. Each key point of SFM has an accurate 3D position and corresponding 2D image. For each 2D instance, feature points within the 2D instance mask are extracted. Then, a set of corresponding 3D keypoints can be considered as candidates for 3D bounding boxes.
Street signs are represented as rectangles with directions in space, which have 6 degrees of freedom, including translation (,,), direction (θ) and size (width and height). Given its depth, a traffic light has 7 degrees of freedom. Traffic cones are represented similarly to traffic lights.
3.proposal refine
- step 1: Extract the outline of each static object from 2D instance segmentation.
- step 2: Fit the minimum oriented bounding box (OBB) for the contour outline.
- step 3: Extract the vertices of the minimum bounding box.
- step 4: Calculate the direction based on the vertices and center points, and determine the vertex order.
- step 5: The segmentation and merging process is performed based on the 2D detection and instance segmentation results.
- step 6: Detect and reject observations containing occlusions. Extracting vertices from the 2D instance segmentation mask requires that all four corners of each sign are visible. If there are occlusions, axis-aligned bounding boxes (AABBs) are extracted from the instance segmentation and the area ratio between AABBs and 2D detection boxes is calculated. If there are no occlusions, these two area calculation methods should be close.
4. Triangulation
Obtain the initial vertex value of the static object under 3D conditions through triangulation.
By checking the number of keypoints in the 3D bounding boxes obtained by SFM and instance segmentation during scene reconstruction, only instances with the number of keypoints exceeding the threshold are considered stable and valid observations. For these instances, the corresponding 2D bounding box is considered a valid observation. Through 2D observation of multiple images, the vertices of the 2D bounding box are triangulated to obtain the coordinates of the bounding box.
For circular signs that do not distinguish the "lower left, upper left, upper right, upper right, and lower right" vertices on the mask, these circular signs need to be identified. 2D detection results are used as observations of circular objects, and 2D instance segmentation masks are used for contour extraction. The center point and radius are calculated through a least squares fitting algorithm. The parameters of the circular sign include the center point (,,), direction (θ) and radius ().
5.tracking refine
Tracking feature point matching based on SFM. Determine whether to merge these separated instances based on the Euclidean distance of the 3D bounding box vertices and the 2D bounding box projection IoU. Once merging is complete, 3D feature points within an instance can be clustered to associate more 2D feature points. Iterative 2D-3D association is performed until no 2D feature points can be added.
6. Final parameter optimization
Taking the rectangular sign as an example, the parameters that can be optimized include position (,,) and direction (θ) and size (,), for a total of six degrees of freedom. The main steps include:
- Convert six degrees of freedom into four 3D points and calculate the rotation matrix.
- Project the converted four 3D points onto the 2D image.
- Calculate the residual between the projection result and the corner point result obtained by instance segmentation.
- Use Huber to optimize and update bounding box parameters
Labeling effect
There are also some challenging long-tail cases, such as extremely low resolution and insufficient lighting.
To summarize
The VRSO framework achieves high-precision and consistent 3D annotation of static objects, tightly integrating detection, segmentation and The SFM algorithm eliminates manual intervention in intelligent driving annotation and provides results comparable to LiDAR-based manual annotation. Qualitative and quantitative evaluations were conducted with the widely recognized Waymo Open Dataset: compared with manual annotation, the speed is increased by about 16 times, while maintaining the best consistency and accuracy.
The above is the detailed content of Efficiency increased by 16 times! VRSO: 3D annotation of purely visual static objects, opening up the data closed loop!. For more information, please follow other related articles on the PHP Chinese website!

Hot AI Tools

Undresser.AI Undress
AI-powered app for creating realistic nude photos

AI Clothes Remover
Online AI tool for removing clothes from photos.

Undress AI Tool
Undress images for free

Clothoff.io
AI clothes remover

Video Face Swap
Swap faces in any video effortlessly with our completely free AI face swap tool!

Hot Article

Hot Tools

Notepad++7.3.1
Easy-to-use and free code editor

SublimeText3 Chinese version
Chinese version, very easy to use

Zend Studio 13.0.1
Powerful PHP integrated development environment

Dreamweaver CS6
Visual web development tools

SublimeText3 Mac version
God-level code editing software (SublimeText3)

Hot Topics
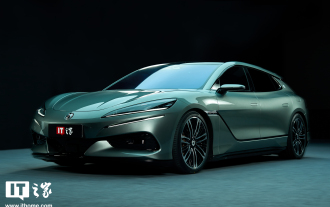
According to news on April 22, this morning, Denza’s new flagship model Z9GT made its debut. Now I would like to share with you some real-life pictures. According to the official introduction, the new car is positioned as a smart luxury flagship sedan, with a length of 5180mm reaching the level of a D-class luxury sedan. BYD's global design director Wolfgang Egger led the efforts to create the appearance. It is equipped with "e³" black technology, multiple lidar and other configurations. , with nearly a thousand horsepower. In terms of design, Denza Z9GT perfectly blends Eastern and Western aesthetics, with an eye-catching front face. On the side of the car body, the "Z" shaped decorative line is exquisite and smooth, and the center of gravity of the car body is relatively far back, creating a backward leaning posture, which is very sporty. The rear of the vehicle is round and full, and the taillights extend from the middle to both sides, echoing the electric tail wings, bringing a high degree of recognition to the entire vehicle. worth
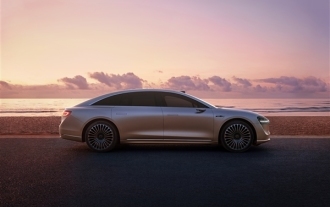
News on April 23: According to the latest reports, the much-anticipated pure electric sedan Enjoy S9 will meet the public for the first time at the upcoming Beijing Auto Show. This car is a masterpiece jointly created by BAIC Blue Valley and Huawei for the two industry giants. It is positioned in the medium and large sedan market and is expected to sell for no less than 500,000 yuan. S9 has recently completed the relevant application process with the Ministry of Industry and Information Technology, indicating that its official launch is approaching. Judging from the exposed information, the new car has a striking exterior design, using a modern through-type LED light group, with three sets of light sources delicately distributed in the headlight group. Its closed front grille and eye-catching front surround heat dissipation vent design jointly create a stable yet fashionable temperament. The data editor understands and enjoys the elegant side lines of the S9's body.
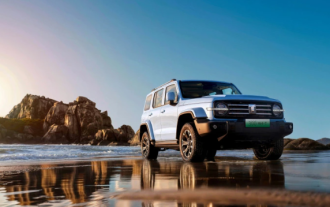
According to news on April 23, recently, the much-anticipated Great Wall Tank 300Hi4-T has finally officially landed on the market. This model has attracted widespread attention due to its unique configuration and limited scarcity. It is reported that only one configuration of the Tank 300Hi4-T is launched this time, with a recommended retail price of 269,800 yuan, and only 3,000 units are available for sale. From the appearance design point of view, Tank 300Hi4-T shows a strong off-road atmosphere. It adopts a professional off-road chassis with a non-load-bearing body, which shows that the vehicle's stability and off-road capabilities are improved. In the front part of the car, the iconic round light set complements the three-frame horizontal grille, and the silver chrome-plated front grille makes the appearance of the vehicle more tough and powerful. The wheel arches and exterior mirrors in the same color as the body add to this car’s
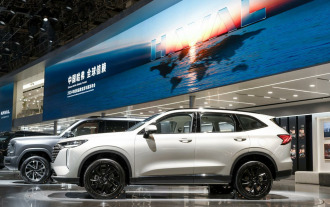
On April 25, 2024, Great Wall Haval made a grand appearance at the 18th Beijing International Automobile Exhibition with its new generation Haval H6, new generation Haval H9, 2024 Haval Raptor and other star models. China Haval is globally trusted, and the Haval brand is accelerating its pursuit of the global market. At this auto show, Great Wall Haval took "China Haval, Global Trust" as its theme, fully interpreting the Haval brand's solid commitment to users as a "global SUV expert". Great Wall Haval has been deeply involved in the SUV field for 13 years, driven by technological innovation, using hard-core product capabilities and reliable services to provide consumers with high-quality SUV products. From leading China to going global, Great Wall Haval continues to create excellent SUV product experiences, respond to the expectations and trust of more users, and write the globalization of Chinese brands
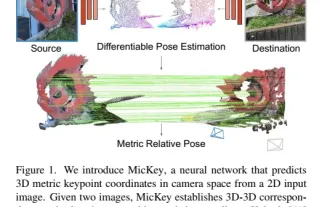
Project link written in front: https://nianticlabs.github.io/mickey/ Given two pictures, the camera pose between them can be estimated by establishing the correspondence between the pictures. Typically, these correspondences are 2D to 2D, and our estimated poses are scale-indeterminate. Some applications, such as instant augmented reality anytime, anywhere, require pose estimation of scale metrics, so they rely on external depth estimators to recover scale. This paper proposes MicKey, a keypoint matching process capable of predicting metric correspondences in 3D camera space. By learning 3D coordinate matching across images, we are able to infer metric relative
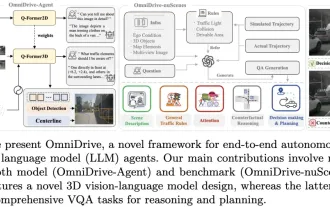
Written above & the author’s personal understanding: This paper is dedicated to solving the key challenges of current multi-modal large language models (MLLMs) in autonomous driving applications, that is, the problem of extending MLLMs from 2D understanding to 3D space. This expansion is particularly important as autonomous vehicles (AVs) need to make accurate decisions about 3D environments. 3D spatial understanding is critical for AVs because it directly impacts the vehicle’s ability to make informed decisions, predict future states, and interact safely with the environment. Current multi-modal large language models (such as LLaVA-1.5) can often only handle lower resolution image inputs (e.g.) due to resolution limitations of the visual encoder, limitations of LLM sequence length. However, autonomous driving applications require
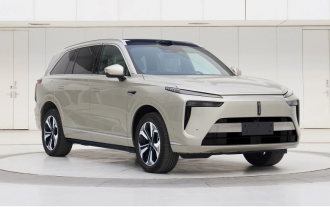
According to reports on May 9, 2024, at this year’s Beijing International Auto Show, Wei Brand, a subsidiary of Great Wall Motors, launched a new model - the Blue Mountain Smart Driving Edition, which attracted the attention of many visitors. According to "Knowing Car Emperor's Vision", this highly anticipated new car is expected to officially land on the market in June this year. The design of the Blue Mountain Smart Driving Edition continues to follow the classic appearance of the Blue Mountain DHT-PHEV on sale, but it has been significantly upgraded in terms of intelligent driving perception. The most eye-catching thing is that a watchtower-style lidar is installed on the roof. At the same time, the vehicle is also equipped with 3 millimeter wave radars and 12 ultrasonic radars, as well as 11 high-definition visual perception cameras, for a total of 27 Assisted driving sensors greatly enhance the vehicle's environmental perception capabilities. according to
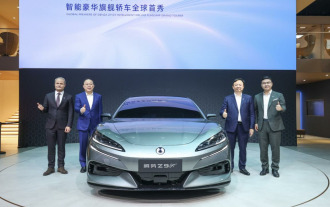
On April 25, the 18th Beijing International Automobile Exhibition 2024 officially kicked off. Denza Motors made a stunning appearance in Hall W4 with the most powerful smart luxury product matrix in history. The booth was crowded with people, making it the most popular check-in point at this Beijing Auto Show! Among them, Denza Z9GT makes its world debut. The new car is positioned as an intelligent luxury flagship sedan. It is another pinnacle work of Wolfgang Egger, design director of BYD Group. It is also the first to use the world's leading disruptive technology platform Yi Sanfang, leading the way. The new trend of electrification of luxury cars. Led by Iger and empowered by disruptive technology, the Denza Z9GT is the focus of this year's auto show. As the first model of Denza's new design concept "Elegance in motion", the appearance of the Denza Z9GT is led by Iger and is perfectly designed.
