


One article to understand the technical challenges and optimization strategies for fine-tuning large language models
Hello everyone, my name is Luga. Today we will continue to explore technologies in the artificial intelligence ecosystem, especially LLM Fine-Tuning. This article will continue to analyze LLM Fine-Tuning technology in depth to help everyone better understand its implementation mechanism so that it can be better applied to market development and other fields.
LLMs (Large Language Models) are leading a new wave of artificial intelligence technology. This advanced AI simulates human cognitive and language abilities by analyzing massive amounts of data using statistical models to learn complex patterns between words and phrases. The powerful functions of LLMs have aroused strong interest from many leading companies and technology enthusiasts, who are rushing to adopt these innovative solutions driven by artificial intelligence, aiming to improve operational efficiency, reduce work burden, reduce cost expenditures, and ultimately inspire Come up with more innovative ideas that create business value.
However, to truly realize the potential of LLMs, the key lies in “customization”. That is, how enterprises can transform general pre-trained models into exclusive models that meet their own unique business needs and use case scenarios through specific optimization strategies. In view of the differences between different enterprises and application scenarios, it is particularly important to choose an appropriate LLM integration method. Therefore, accurately assessing specific use case requirements and understanding the subtle differences and trade-offs between different integration options will help enterprises make informed decisions.
What is Fine-Tuning?
In today’s era of popularization of knowledge, it has never been easier to obtain information and opinions about AI and LLM. However, finding practical, context-specific professional answers remains a challenge. In our daily life, we often encounter such a common misunderstanding: it is generally believed that Fine-Tuning (fine-tuning) models are the only (or perhaps the best) way for LLM to acquire new knowledge. In fact, whether you are adding intelligent collaborative assistants to your products or using LLM to analyze large amounts of unstructured data stored in the cloud, your actual data and business environment are key factors in choosing the right LLM approach.
In many cases, it is often useful to adopt alternative strategies that are less complex to operate, more robust to frequently changing data sets, and produce more reliable and accurate results than traditional fine-tuning methods. Achieve corporate goals more effectively. Although fine-tuning is a common LLM customization technique that performs additional training on a pre-trained model on a specific dataset to better adapt it to a specific task or domain, it also has some important trade-offs and limitations.
So, what is Fine-Tuning?
LLM (Large Language Model) Fine-tuning is one of the technologies that has attracted much attention in the field of NLP (Natural Language Processing) in recent years. It allows the model to better adapt to a specific domain or task by performing additional training on an already trained model. This method enables the model to learn more knowledge related to a specific domain, thereby achieving better performance in this domain or task. The advantage of LLM fine-tuning is that it takes advantage of the general knowledge that the pre-trained model has learned, and then further fine-tunes it on a specific domain to achieve higher accuracy and performance on specific tasks. This method has been widely used in various NLP tasks and has achieved remarkable results.
The main concept of LLM fine-tuning is to use the parameters of the pre-trained model as the basis for new tasks, and through fine-tuning a small amount of specific domain or task data, the model can quickly adapt to new tasks or data sets. This method can save a lot of training time and resources while improving the model's performance on new tasks. The flexibility and efficiency of LLM fine-tuning make it one of the preferred methods in many natural language processing tasks. By fine-tuning on top of a pre-trained model, the model can learn features and patterns for new tasks faster, thereby improving overall performance. This
In actual business scenarios, the main purposes of fine-tuning usually include the following points:
(1) Domain adaptation
LLM is usually trained on cross-domain general data, but when applied to specific fields, such as finance, medical, legal and other scenarios, the performance may be greatly compromised. Through fine-tuning, the pre-trained model can be adjusted and adapted to the target domain so that it can better capture the language characteristics and semantic relationships of a specific domain, thereby improving performance in this domain.
(2) Task customization
Even in the same field, different specific tasks may have differentiated requirements. For example, NLP tasks such as text classification, question answering, named entity recognition, etc. will put forward different requirements for language understanding and generation capabilities. Through fine-tuning, the performance indicators of the model on specific tasks, such as accuracy, Recall, F1 value, etc., can be optimized according to the specific needs of downstream tasks.
(3) Performance improvement
Even on a specific task, the pre-trained model may have bottlenecks in accuracy, speed, etc. Through fine-tuning, we can further improve the model's performance on this task. For example, for real-time application scenarios that require high inference speed, the model can be compressed and optimized; for key tasks that require higher accuracy, the model's judgment ability can also be further improved through fine-tuning.
What are the benefits and difficulties faced by Fine-Tuning (fine-tuning)?
Generally speaking, the main benefit of Fine-Tuning (fine-tuning) is that it can effectively improve the performance of existing pre-trained models in specific Performance in application scenarios. Through continuous training and parameter adjustment of the basic model in the target field or task, it can better capture the semantic characteristics and patterns in specific scenarios, thereby significantly improving the key indicators of the model in this field or task. For example, by fine-tuning the Llama 2 model, performance on some features can be better than Meta's original language model implementation.
Although Fine-Tuning brings significant benefits to LLM, there are also some disadvantages to consider. So, what are the difficulties faced by Fine-Tuning?
Challenges and limitations:
- Catastrophic forgetting: Fine-tuning may lead to "catastrophic forgetting", that is, the model forgets some common sense learned during pre-training. This can happen if the nudge data is too specific or focuses primarily on a narrow area.
- Data requirements: Although fine-tuning requires less data than training from scratch, high-quality and relevant data is still required for the specific task. Insufficient or improperly labeled data can lead to poor performance.
- Computational resources: The fine-tuning process remains computationally expensive, especially for complex models and large data sets. For smaller organizations or those with limited resources, this can be a barrier.
- Expertise required: Fine-tuning often requires expertise in areas such as machine learning, NLP, and the specific task at hand. Choosing the right pretrained model, configuring hyperparameters and evaluating results can be complicated for those without the necessary knowledge.
Potential issues:
- Bias amplification: Pre-trained models can inherit bias from their training data. If the nudged data reflects similar biases, the nudge may inadvertently amplify these biases. This may lead to unfair or discriminatory outcomes.
- Interpretability Challenge: Fine-tuned models are more difficult to interpret than pre-trained models. Understanding how a model reaches its results can be difficult, which hinders debugging and trust in the model's output.
- Security Risk: Fine-tuned models may be vulnerable to adversarial attacks, in which a malicious actor manipulates input data, causing the model to produce incorrect output.
How does Fine-Tuning compare to other customization methods?
Generally speaking, Fine-Tuning is not the only way to customize model output or integrate custom data . In fact, it may not be suitable for our specific needs and use cases. There are some other alternatives worth exploring and considering, as follows:
1. Prompt Engineering
Prompt Engineering is a process that increases the likelihood of obtaining the desired output by providing detailed instructions or contextual data in the hints sent to the AI model. Prompt Engineering is much less complex to operate than fine-tuning, and prompts can be modified and redeployed at any time without any changes to the underlying model.
This strategy is relatively simple, but a data-driven approach should still be used to quantitatively evaluate the accuracy of various tips to ensure the desired performance. In this way, we can systematically refine the cues to find the most efficient way to guide the model to produce the desired output.
However, Prompt Engineering is not without its shortcomings. First, it cannot directly integrate large data sets because prompts are usually modified and deployed manually. This means that Prompt Engineering may appear less efficient when processing large-scale data.
In addition, Prompt Engineering cannot allow the model to generate new behaviors or functions that do not exist in the basic training data. This limitation means that if we need the model to have completely new capabilities, relying solely on hint engineering may not be able to meet the needs, and other methods may need to be considered, such as fine-tuning or training the model from scratch.
2. RAG (Retrieval Augmented Generation)
RAG (Retrieval Augmented Generation) is an effective way to combine large unstructured data sets (such as documents) with LLM. It leverages semantic search and vector database technologies, combined with a hinting mechanism, to enable LLM to obtain the required knowledge and context from rich external information to generate more accurate and insightful output.
Although RAG itself is not a mechanism for generating new model features, it is an extremely powerful tool for efficiently integrating LLM with large-scale unstructured data sets. Using RAG, we can easily provide LLMs with a large amount of relevant background information, enhancing their knowledge and understanding, thereby significantly improving generation performance.
In actual scenarios, the biggest obstacle to the effectiveness of RAG is that many models have a limited context window, that is, the maximum text length that the model can process at one time is limited. In some situations where extensive background knowledge is required, it may prevent the model from obtaining enough information to achieve good performance.
However, with the rapid development of technology, the context window of the model is rapidly expanding. Even some open source models have been able to handle long text inputs of up to 32,000 tokens. This means that RAG will have broader application prospects in the future and can provide strong support for more complex tasks.
Next, let us understand and compare the specific performance of these three technologies in terms of data privacy. For details, please refer to the following:
(1) Fine-Tuning )
The main disadvantage of Fine-Tuning is that the information used when training the model is encoded into the parameters of the model. This means that even if the model's output is private to the user, the underlying training data may still be leaked. Research shows that malicious attackers can even extract raw training data from models through injection attacks. Therefore, we must assume that any data used to train the model may be accessible to future users.
(2) Prompt Engineering(Prompt Engineering)
In comparison, Prompt Engineering’s data security footprint is much smaller. Because prompts can be isolated and customized for each user, the data contained in the prompts seen by different users can be different. But we still need to ensure that any data contained in the prompt is non-sensitive or permissible to any user with access to the prompt.
(3) RAG (Retrieval Enhancement Generation)
The security of RAG depends on the data access control in its underlying retrieval system. We need to ensure that the underlying vector database and prompt templates are configured with appropriate privacy and data controls to prevent unauthorized access. Only in this way can RAG truly ensure data privacy.
Overall, Prompt Engineering and RAG have clear advantages over fine-tuning when it comes to data privacy. But no matter which method is adopted, we must manage data access and privacy protection very carefully to ensure that users' sensitive information is fully protected.
So, in a sense, whether we ultimately choose Fine-Tuning, Prompt Engineering or RAG, the approach adopted should be consistent with the organization's strategic goals, available resources, expertise and expected return on investment Rates and other factors remain highly consistent. It’s not just about pure technical capabilities, but also about how these approaches fit with our business strategy, timelines, current workflows, and market needs.
Understanding the intricacies of the Fine-Tuning option is key to making informed decisions. The technical details and data preparation involved in Fine-Tuning are relatively complex and require an in-depth understanding of the model and data. Therefore, it is crucial to work closely with a partner with extensive fine-tuning experience. These partners must not only have reliable technical capabilities, but also be able to fully understand our business processes and goals and choose the most appropriate customized technical solutions for us.
Similarly, if we choose to use Prompt Engineering or RAG, we also need to carefully evaluate whether these methods can match our business needs, resource conditions, and expected effects. Ultimately success can only be achieved by ensuring that the customized technology chosen can truly create value for our organization.
Reference:
- [1] https://medium.com/@younesh.kc/rag-vs-fine-tuning-in-large-language-models-a -comparison-c765b9e21328
- [2] https://kili-technology.com/large-language-models-llms/the-ultimate-guide-to-fine-tuning-llms-2023
The above is the detailed content of One article to understand the technical challenges and optimization strategies for fine-tuning large language models. For more information, please follow other related articles on the PHP Chinese website!

Hot AI Tools

Undresser.AI Undress
AI-powered app for creating realistic nude photos

AI Clothes Remover
Online AI tool for removing clothes from photos.

Undress AI Tool
Undress images for free

Clothoff.io
AI clothes remover

Video Face Swap
Swap faces in any video effortlessly with our completely free AI face swap tool!

Hot Article

Hot Tools

Notepad++7.3.1
Easy-to-use and free code editor

SublimeText3 Chinese version
Chinese version, very easy to use

Zend Studio 13.0.1
Powerful PHP integrated development environment

Dreamweaver CS6
Visual web development tools

SublimeText3 Mac version
God-level code editing software (SublimeText3)

Hot Topics
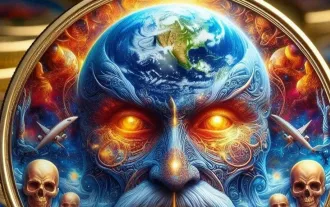
WorldCoin (WLD) stands out in the cryptocurrency market with its unique biometric verification and privacy protection mechanisms, attracting the attention of many investors. WLD has performed outstandingly among altcoins with its innovative technologies, especially in combination with OpenAI artificial intelligence technology. But how will the digital assets behave in the next few years? Let's predict the future price of WLD together. The 2025 WLD price forecast is expected to achieve significant growth in WLD in 2025. Market analysis shows that the average WLD price may reach $1.31, with a maximum of $1.36. However, in a bear market, the price may fall to around $0.55. This growth expectation is mainly due to WorldCoin2.
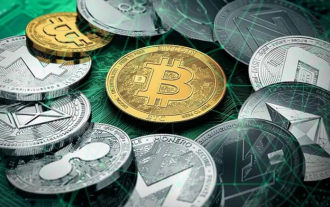
Factors of rising virtual currency prices include: 1. Increased market demand, 2. Decreased supply, 3. Stimulated positive news, 4. Optimistic market sentiment, 5. Macroeconomic environment; Decline factors include: 1. Decreased market demand, 2. Increased supply, 3. Strike of negative news, 4. Pessimistic market sentiment, 5. Macroeconomic environment.
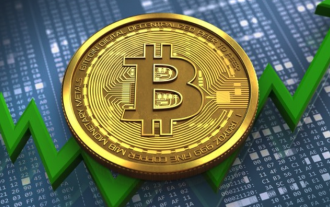
Exchanges that support cross-chain transactions: 1. Binance, 2. Uniswap, 3. SushiSwap, 4. Curve Finance, 5. Thorchain, 6. 1inch Exchange, 7. DLN Trade, these platforms support multi-chain asset transactions through various technologies.

Aavenomics is a proposal to modify the AAVE protocol token and introduce token repos, which has implemented a quorum for AAVEDAO. Marc Zeller, founder of the AAVE Project Chain (ACI), announced this on X, noting that it marks a new era for the agreement. Marc Zeller, founder of the AAVE Chain Initiative (ACI), announced on X that the Aavenomics proposal includes modifying the AAVE protocol token and introducing token repos, has achieved a quorum for AAVEDAO. According to Zeller, this marks a new era for the agreement. AaveDao members voted overwhelmingly to support the proposal, which was 100 per week on Wednesday
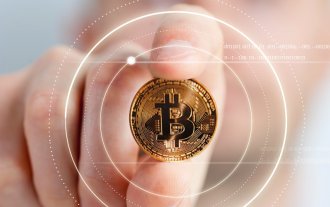
The steps to draw a Bitcoin structure analysis chart include: 1. Determine the purpose and audience of the drawing, 2. Select the right tool, 3. Design the framework and fill in the core components, 4. Refer to the existing template. Complete steps ensure that the chart is accurate and easy to understand.
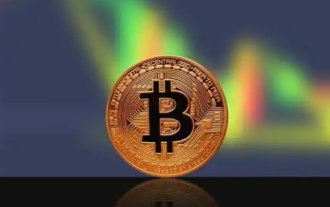
Cryptocurrency data platforms suitable for beginners include CoinMarketCap and non-small trumpet. 1. CoinMarketCap provides global real-time price, market value, and trading volume rankings for novice and basic analysis needs. 2. The non-small quotation provides a Chinese-friendly interface, suitable for Chinese users to quickly screen low-risk potential projects.
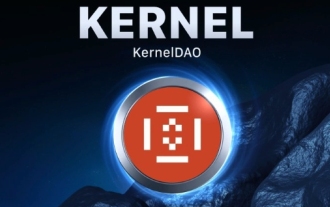
In the bustling world of cryptocurrencies, new opportunities always emerge. At present, KernelDAO (KERNEL) airdrop activity is attracting much attention and attracting the attention of many investors. So, what is the origin of this project? What benefits can BNB Holder get from it? Don't worry, the following will reveal it one by one for you.
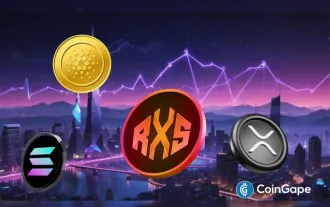
In the volatile cryptocurrency market, investors are looking for alternatives that go beyond popular currencies. Although well-known cryptocurrencies such as Solana (SOL), Cardano (ADA), XRP and Dogecoin (DOGE) also face challenges such as market sentiment, regulatory uncertainty and scalability. However, a new emerging project, RexasFinance (RXS), is emerging. It does not rely on celebrity effects or hype, but focuses on combining real-world assets (RWA) with blockchain technology to provide investors with an innovative way to invest. This strategy makes it hoped to be one of the most successful projects of 2025. RexasFi
