Python Data Analysis: Data Exploration and Prediction
preface
Data science has become increasingly popular and has been widely used in various industries. pandas is an open source library for data manipulation and modeling and is a valuable tool for data scientists. In this article, we will explore how to use Pandas for data exploration and modeling.
Data Exploration
Data exploration is a crucial first step in the data science process, which allows us to have an intuitive understanding of the data. Using Pandas, we can load the data and view its contents.
import numpy as np import numpy as np import matplotlib.pyplot as plts data = pd.read_csv("data.csv")
Tabular output provides perspective on the data, while charts help us visualize the data to look for trends and outliers.
data.head() data.hist() plt.show()
Data preprocessing
Before modeling data, data preprocessing is usually required to ensure data integrity and consistency. This may involve cleaning up missing values, standardizing features, or converting categorical data into a numerical form that can be trained on the model.
data.dropna(inplace=True) data = (data - data.min()) / (data.max() - data.min()) data["cateGory"] = data["category].astype("category")
Data Modeling
Once the data is ready, we can start modeling. Pandas has built-in support for various libraries for common statistical modeling, such as linear regression, logistic regression, and decision trees.
from sklearn.linear_model import LoGISticRegression model = LogisticRegression() model.fit(data[["feature1", "feature2"]], data["target"])
Model Evaluation
After training the model, the next step is to evaluate its performance. We can use evaluation metrics such as confusion matrix, precision, recall, F1-score, etc.
import sklearn.matrics as metics predictions = model.predict(x_test) print(metices.confusion_matrix(y_test, predictions)) print(metices.accuracy_score(y_test, predictions))
Summarize
Using Pandas for data exploration and modeling is the cornerstone of the data science process. Pandas' intuitive syntax and built-in support for statistical modeling libraries make it ideal for doing data science quickly and efficiently. As we continue to advance in the field of data science, staying proficient in Pandas will greatly benefit us as we navigate the ever-changing landscape of data-driven insights and drive decision-making.
The above is the detailed content of Python Data Analysis: Data Exploration and Prediction. For more information, please follow other related articles on the PHP Chinese website!

Hot AI Tools

Undresser.AI Undress
AI-powered app for creating realistic nude photos

AI Clothes Remover
Online AI tool for removing clothes from photos.

Undress AI Tool
Undress images for free

Clothoff.io
AI clothes remover

Video Face Swap
Swap faces in any video effortlessly with our completely free AI face swap tool!

Hot Article

Hot Tools

Notepad++7.3.1
Easy-to-use and free code editor

SublimeText3 Chinese version
Chinese version, very easy to use

Zend Studio 13.0.1
Powerful PHP integrated development environment

Dreamweaver CS6
Visual web development tools

SublimeText3 Mac version
God-level code editing software (SublimeText3)

Hot Topics
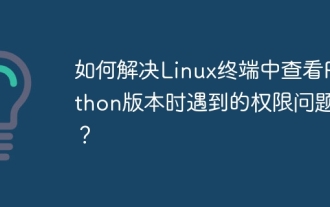
Solution to permission issues when viewing Python version in Linux terminal When you try to view Python version in Linux terminal, enter python...
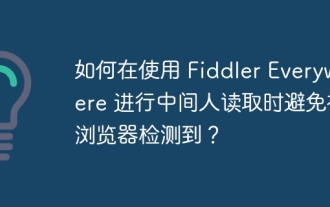
How to avoid being detected when using FiddlerEverywhere for man-in-the-middle readings When you use FiddlerEverywhere...
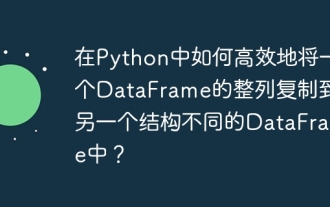
When using Python's pandas library, how to copy whole columns between two DataFrames with different structures is a common problem. Suppose we have two Dats...
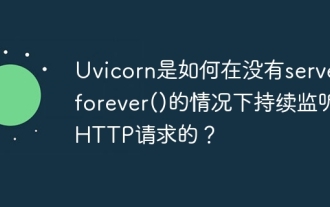
How does Uvicorn continuously listen for HTTP requests? Uvicorn is a lightweight web server based on ASGI. One of its core functions is to listen for HTTP requests and proceed...
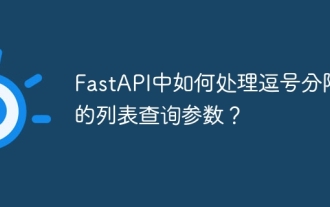
Fastapi ...
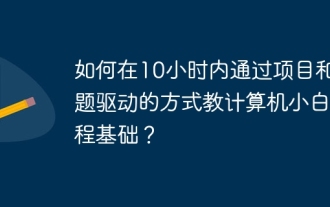
How to teach computer novice programming basics within 10 hours? If you only have 10 hours to teach computer novice some programming knowledge, what would you choose to teach...
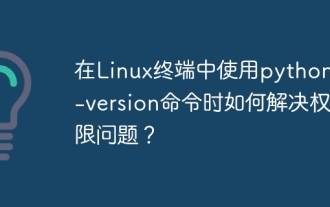
Using python in Linux terminal...
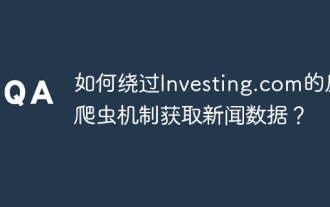
Understanding the anti-crawling strategy of Investing.com Many people often try to crawl news data from Investing.com (https://cn.investing.com/news/latest-news)...
