


The latest benchmark CMMMU suitable for Chinese LMM physique: includes more than 30 subdivisions and 12K expert-level questions
As multimodal large models (LMMs) continue to advance, the need to evaluate the performance of LMMs is also growing. Especially in the Chinese environment, it becomes more important to evaluate the advanced knowledge and reasoning ability of LMM.
In this context, in order to evaluate the expert-level multi-modal understanding capabilities of the basic model in various tasks in Chinese, the M-A-P open source community, Hong Kong University of Science and Technology, University of Waterloo and Zero-One Everything Jointly launched the CMMMU (Chinese Massive Multi-discipline Multimodal Understanding and Reasoning) benchmark. This benchmark aims to provide a comprehensive evaluation platform for large-scale multi-disciplinary multi-modal understanding and reasoning in Chinese. The benchmark allows researchers to test models on a variety of tasks and compare their multimodal understanding capabilities to professional levels. The goal of this joint project is to promote the development of the field of Chinese multimodal understanding and reasoning and provide a standardized reference for related research.
CMMMU covers six major categories of subjects, including arts, business, health and medicine, science, humanities and social sciences, technology and engineering, involving more than 30 sub-field subjects. The figure below shows an example of a question for each sub-field subject. CMMMU is one of the first multi-modal benchmarks in the Chinese context and one of the few multi-modal benchmarks that examines the complex understanding and reasoning capabilities of LMM.
Dataset construction
##Data collection
Data collection is divided into three stages. First, the researchers collected question sources for each subject that met copyright licensing requirements, including web pages or books. During this process, they worked hard to avoid duplication of question sources to ensure data diversity and accuracy. Secondly, the researchers forwarded the question sources to crowdsourced annotators for further annotation. All annotators are individuals with a bachelor's degree or higher to ensure they can verify the annotated questions and associated explanations. During the annotation process, researchers require annotators to strictly follow the annotation principles. For example, filter out questions that don't require pictures to answer, filter out questions that use the same images whenever possible, and filter out questions that don't require expert knowledge to answer. Finally, in order to balance the number of questions in each subject in the data set, the researchers specifically supplemented the subjects with fewer questions. Doing so ensures the completeness and representativeness of the data set, allowing subsequent analysis and research to be more accurate and comprehensive.
Dataset Cleaning
In order to further improve the data quality of CMMMU, researchers follow strict data quality control protocol. First, each question is personally verified by at least one of the paper's authors. Secondly, in order to avoid data pollution problems, they also screened out questions that several LLMs could answer without resorting to OCR technology. These measures ensure the reliability and accuracy of CMMMU data.
Dataset Overview
CMMMU has a total of 12K questions, which are divided into few-sample development set, verification set and test set. The few-sample development set contains about 5 questions for each subject, the validation set has 900 questions, and the test set has 11K questions. The questions cover 39 types of pictures, including pathological diagrams, musical notation diagrams, circuit diagrams, chemical structure diagrams, etc. The questions are divided into three difficulty levels: easy (30%), medium (58%), and hard (12%) based on logical difficulty rather than intellectual difficulty. More question statistics can be found in Table 2 and Table 3.
experiment
The team tested the performance of a variety of mainstream Chinese and English bilingual LMMs and several LLMs on CMMMU. Both closed source and open source models are included. The evaluation process uses zero-shot settings instead of fine-tuning or few-shot settings to check the raw capabilities of the model. LLM also added experiments in which image OCR result text is used as input. All experiments were performed on an NVIDIA A100 graphics processor.
Main results
Table 4 shows the experimental results:
Some important findings include:
- CMMMU is more challenging than MMMU, and this is when MMMU has Very challenging premise.
The accuracy of GPT-4V in the Chinese context is only 41.7%, while the accuracy in the English context is 55.7%. This shows that existing cross-language generalization methods are not good enough even for state-of-the-art closed-source LMMs.
- Compared with MMMU, the gap between the domestic representative open source model and GPT-4V is relatively small.
The difference between Qwen-VL-Chat and GPT-4V on MMMU is 13.3%, while the difference between BLIP2-FLAN-T5-XXL and GPT-4V on MMMU is 21.9%. Surprisingly, Yi-VL-34B even narrows the gap between the open source bilingual LMM and GPT-4V on CMMMU to 7.5%, which means that in the Chinese environment, the open source bilingual LMM is equivalent to GPT-4V, which is This is a promising development in the open source community.
# - In the open source community, the game in the pursuit of multimodal artificial general intelligence (AGI) for Chinese experts is just beginning.
The team pointed out that except for the recently released Qwen-VL-Chat, Yi-VL-6B and Yi-VL-34B, all bilingual LMMs from the open source community can only Achieve accuracy comparable to CMMMU's frequent choice.
Analysis of different question difficulty and question types
- Different question types
The difference between Yi-VL series, Qwen-VL-Plus and GPT-4V is mainly due to their different abilities to answer multiple-choice questions.
The results of different question types are shown in Table 5:
- Different questions Difficulty
What is worth noting in the results is that the best open source LMM (i.e. Yi-VL-34B) and GPT-4V exist when facing medium and hard problems A larger gap. This is further strong evidence that the key difference between open source LMMs and GPT-4V is the ability to compute and reason under complex conditions.
The results of different question difficulties are shown in Table 6:
Error analysis
Researchers carefully analyzed the wrong answers of GPT-4V. As shown in the figure below, the main types of errors are perception errors, lack of knowledge, reasoning errors, refusal to answer, and annotation errors. Analyzing these error types is key to understanding the capabilities and limitations of current LMMs and can also guide future design and training model improvements.
- Perceived error (26%): Perceived error is an example of an error generated by GPT-4V One of the main reasons. On the one hand, when the model cannot understand the image, it introduces a bias to the underlying perception of the image, leading to incorrect responses. On the other hand, when a model encounters ambiguities in domain-specific knowledge, implicit meanings, or unclear formulas, it often exhibits domain-specific perceptual errors. In this case, GPT-4V tends to rely more on textual information-based answers (i.e. questions and options), prioritizing textual information over visual input, resulting in a bias in understanding multi-modal data.
- Inference Errors (26%) : Inference errors are another major factor in GPT-4V producing erroneous examples. Even when models correctly perceive the meaning conveyed by images and text, errors can still occur during reasoning when solving problems that require complex logical and mathematical reasoning. Typically, this error is caused by the model's weak logical and mathematical reasoning capabilities.
- Lack of knowledge (22%): Lack of expertise is also one of the reasons for incorrect answers to GPT-4V. Since CMMMU is a benchmark for evaluating LMM expert AGI, expert-level knowledge in different disciplines and subfields is required. Therefore, injecting expert-level knowledge into LMM is also one of the directions that can be worked on.
- Refusal to answer (12%): It is also common for models to refuse to answer. Through analysis, they pointed out several reasons why the model refused to answer the question: (1) The model failed to perceive information from the image; (2) It was a question involving religious issues or personal real-life information, and the model would actively avoid it; (3) When questions involve gender and subjective factors, the model avoids providing direct answers.
- Its errors: The remaining errors include text comprehension errors (7%), annotation errors (2%) and answer extraction errors (5%). These errors are caused by a variety of factors such as complex structure tracking capabilities, complex text logic understanding, limitations in response generation, errors in data annotation, and problems encountered in answer matching extraction.
Conclusion
The CMMMU benchmark marks significant progress in the development of advanced general artificial intelligence (AGI). CMMMU is designed to rigorously evaluate the latest large multimodal models (LMMs) and test basic perceptual skills, complex logical reasoning, and deep expertise in a specific domain. This study pointed out the differences by comparing the reasoning ability of LMM in Chinese and English bilingual contexts. This detailed assessment is critical in determining how far a model falls short of the proficiency of experienced professionals in each field.
The above is the detailed content of The latest benchmark CMMMU suitable for Chinese LMM physique: includes more than 30 subdivisions and 12K expert-level questions. For more information, please follow other related articles on the PHP Chinese website!

Hot AI Tools

Undresser.AI Undress
AI-powered app for creating realistic nude photos

AI Clothes Remover
Online AI tool for removing clothes from photos.

Undress AI Tool
Undress images for free

Clothoff.io
AI clothes remover

Video Face Swap
Swap faces in any video effortlessly with our completely free AI face swap tool!

Hot Article

Hot Tools

Notepad++7.3.1
Easy-to-use and free code editor

SublimeText3 Chinese version
Chinese version, very easy to use

Zend Studio 13.0.1
Powerful PHP integrated development environment

Dreamweaver CS6
Visual web development tools

SublimeText3 Mac version
God-level code editing software (SublimeText3)

Hot Topics










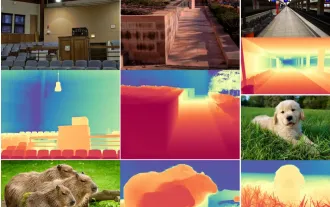
0.What does this article do? We propose DepthFM: a versatile and fast state-of-the-art generative monocular depth estimation model. In addition to traditional depth estimation tasks, DepthFM also demonstrates state-of-the-art capabilities in downstream tasks such as depth inpainting. DepthFM is efficient and can synthesize depth maps within a few inference steps. Let’s read about this work together ~ 1. Paper information title: DepthFM: FastMonocularDepthEstimationwithFlowMatching Author: MingGui, JohannesS.Fischer, UlrichPrestel, PingchuanMa, Dmytr
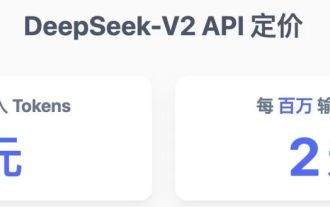
Imagine an artificial intelligence model that not only has the ability to surpass traditional computing, but also achieves more efficient performance at a lower cost. This is not science fiction, DeepSeek-V2[1], the world’s most powerful open source MoE model is here. DeepSeek-V2 is a powerful mixture of experts (MoE) language model with the characteristics of economical training and efficient inference. It consists of 236B parameters, 21B of which are used to activate each marker. Compared with DeepSeek67B, DeepSeek-V2 has stronger performance, while saving 42.5% of training costs, reducing KV cache by 93.3%, and increasing the maximum generation throughput to 5.76 times. DeepSeek is a company exploring general artificial intelligence
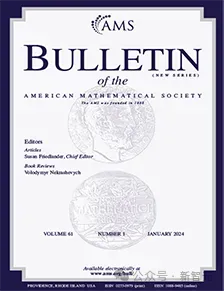
AI is indeed changing mathematics. Recently, Tao Zhexuan, who has been paying close attention to this issue, forwarded the latest issue of "Bulletin of the American Mathematical Society" (Bulletin of the American Mathematical Society). Focusing on the topic "Will machines change mathematics?", many mathematicians expressed their opinions. The whole process was full of sparks, hardcore and exciting. The author has a strong lineup, including Fields Medal winner Akshay Venkatesh, Chinese mathematician Zheng Lejun, NYU computer scientist Ernest Davis and many other well-known scholars in the industry. The world of AI has changed dramatically. You know, many of these articles were submitted a year ago.
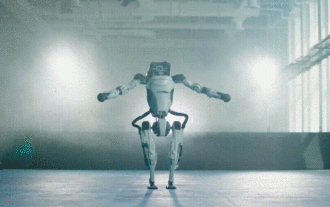
Boston Dynamics Atlas officially enters the era of electric robots! Yesterday, the hydraulic Atlas just "tearfully" withdrew from the stage of history. Today, Boston Dynamics announced that the electric Atlas is on the job. It seems that in the field of commercial humanoid robots, Boston Dynamics is determined to compete with Tesla. After the new video was released, it had already been viewed by more than one million people in just ten hours. The old people leave and new roles appear. This is a historical necessity. There is no doubt that this year is the explosive year of humanoid robots. Netizens commented: The advancement of robots has made this year's opening ceremony look like a human, and the degree of freedom is far greater than that of humans. But is this really not a horror movie? At the beginning of the video, Atlas is lying calmly on the ground, seemingly on his back. What follows is jaw-dropping
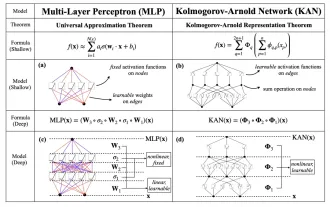
Earlier this month, researchers from MIT and other institutions proposed a very promising alternative to MLP - KAN. KAN outperforms MLP in terms of accuracy and interpretability. And it can outperform MLP running with a larger number of parameters with a very small number of parameters. For example, the authors stated that they used KAN to reproduce DeepMind's results with a smaller network and a higher degree of automation. Specifically, DeepMind's MLP has about 300,000 parameters, while KAN only has about 200 parameters. KAN has a strong mathematical foundation like MLP. MLP is based on the universal approximation theorem, while KAN is based on the Kolmogorov-Arnold representation theorem. As shown in the figure below, KAN has
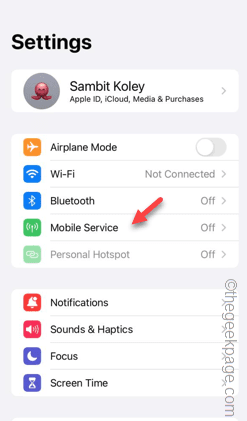
Facing lag, slow mobile data connection on iPhone? Typically, the strength of cellular internet on your phone depends on several factors such as region, cellular network type, roaming type, etc. There are some things you can do to get a faster, more reliable cellular Internet connection. Fix 1 – Force Restart iPhone Sometimes, force restarting your device just resets a lot of things, including the cellular connection. Step 1 – Just press the volume up key once and release. Next, press the Volume Down key and release it again. Step 2 – The next part of the process is to hold the button on the right side. Let the iPhone finish restarting. Enable cellular data and check network speed. Check again Fix 2 – Change data mode While 5G offers better network speeds, it works better when the signal is weaker
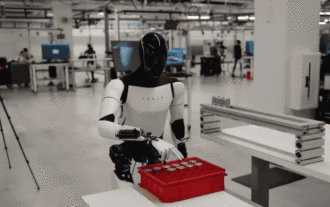
The latest video of Tesla's robot Optimus is released, and it can already work in the factory. At normal speed, it sorts batteries (Tesla's 4680 batteries) like this: The official also released what it looks like at 20x speed - on a small "workstation", picking and picking and picking: This time it is released One of the highlights of the video is that Optimus completes this work in the factory, completely autonomously, without human intervention throughout the process. And from the perspective of Optimus, it can also pick up and place the crooked battery, focusing on automatic error correction: Regarding Optimus's hand, NVIDIA scientist Jim Fan gave a high evaluation: Optimus's hand is the world's five-fingered robot. One of the most dexterous. Its hands are not only tactile
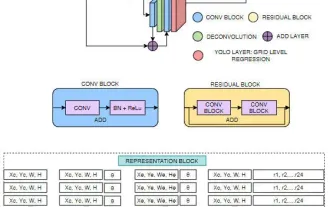
Target detection is a relatively mature problem in autonomous driving systems, among which pedestrian detection is one of the earliest algorithms to be deployed. Very comprehensive research has been carried out in most papers. However, distance perception using fisheye cameras for surround view is relatively less studied. Due to large radial distortion, standard bounding box representation is difficult to implement in fisheye cameras. To alleviate the above description, we explore extended bounding box, ellipse, and general polygon designs into polar/angular representations and define an instance segmentation mIOU metric to analyze these representations. The proposed model fisheyeDetNet with polygonal shape outperforms other models and simultaneously achieves 49.5% mAP on the Valeo fisheye camera dataset for autonomous driving
