


Complete list of numpy functions and their uses: Detailed explanation of all functions in the numpy library
numpy function encyclopedia: Detailed explanation of all functions and their uses in the numpy library, specific code examples are required
Introduction:
In the field of data analysis and scientific computing , often need to process large-scale numerical data. Numpy is the most commonly used open source library in Python, providing efficient multi-dimensional array objects and a series of functions for operating arrays. This article will introduce in detail all the functions and their uses in the numpy library, and give specific code examples to help readers better understand and use the numpy library.
1. Creation and transformation of arrays
- np.array(): Create an array and convert the input data into an ndarray object.
import numpy as np arr = np.array([1, 2, 3, 4, 5]) print(arr)
The output result is:
[1 2 3 4 5]
- np.arange(): Create an arithmetic array.
import numpy as np arr = np.arange(0, 10, 2) print(arr)
The output result is:
[0 2 4 6 8]
- np.zeros(): Create an array whose elements are all 0.
import numpy as np arr = np.zeros((2, 3)) print(arr)
The output result is:
[[0. 0. 0.] [0. 0. 0.]]
- np.ones(): Create an array with all elements being 1.
import numpy as np arr = np.ones((2, 3)) print(arr)
The output result is:
[[1. 1. 1.] [1. 1. 1.]]
- np.linspace(): Create an equally spaced array.
import numpy as np arr = np.linspace(0,1,5) print(arr)
The output result is:
[0. 0.25 0.5 0.75 1. ]
- np.eye(): Create a matrix with a diagonal of 1.
import numpy as np arr = np.eye(3) print(arr)
The output result is:
[[1. 0. 0.] [0. 1. 0.] [0. 0. 1.]]
2. Array operations and calculations
- Array shape operations
- np.reshape(): Change the shape of the array.
import numpy as np arr = np.arange(1, 10) arr_reshape = np.reshape(arr, (3, 3)) print(arr_reshape)
The output result is:
[[1 2 3] [4 5 6] [7 8 9]]
- arr.flatten(): Convert a multi-dimensional array to a one-dimensional array.
import numpy as np arr = np.array([[1, 2, 3], [4, 5, 6]]) arr_flatten = arr.flatten() print(arr_flatten)
The output result is:
[1 2 3 4 5 6]
- Array element operation
- np.sort(): Array element operation Sort.
import numpy as np arr = np.array([3, 1, 5, 2, 4]) arr_sorted = np.sort(arr) print(arr_sorted)
The output result is:
[1 2 3 4 5]
- np.argmax(): Returns the index of the largest element in the array.
import numpy as np arr = np.array([3, 1, 5, 2, 4]) max_index = np.argmax(arr) print(max_index)
The output result is:
2
- Array operations
- np.add(): Add two arrays .
import numpy as np arr1 = np.array([1, 2, 3]) arr2 = np.array([4, 5, 6]) result = np.add(arr1, arr2) print(result)
The output result is:
[5 7 9]
- np.dot(): Dot multiplication of two arrays.
import numpy as np arr1 = np.array([1, 2, 3]) arr2 = np.array([4, 5, 6]) result = np.dot(arr1, arr2) print(result)
The output result is:
32
3. Statistical functions and linear algebra functions
- Statistical functions
- np.mean(): Calculate the mean of the array.
import numpy as np arr = np.array([1, 2, 3, 4, 5]) mean = np.mean(arr) print(mean)
The output result is:
3.0
- np.std(): Calculate the standard deviation of the array.
import numpy as np arr = np.array([1, 2, 3, 4, 5]) std = np.std(arr) print(std)
The output result is:
1.4142135623730951
- Linear algebra function
- np.linalg.det(): Calculate the matrix determinant.
import numpy as np matrix = np.array([[1, 2], [3, 4]]) det = np.linalg.det(matrix) print(det)
The output result is:
-2.0000000000000004
- np.linalg.inv(): Calculate the inverse matrix of the matrix.
import numpy as np matrix = np.array([[1, 2], [3, 4]]) inv = np.linalg.inv(matrix) print(inv)
The output result is:
[[-2. 1. ] [ 1.5 -0.5]]
IV. Auxiliary functions and general functions
- Auxiliary functions
- np.loadtxt(): Load data from a text file.
import numpy as np arr = np.loadtxt('data.txt') print(arr)
- np.savetxt(): Save data to a text file.
import numpy as np arr = np.array([1, 2, 3, 4, 5]) np.savetxt('data.txt', arr)
- General function
- np.sin(): Calculate the sine value of the elements in the array.
import numpy as np arr = np.array([0, np.pi / 2, np.pi]) sin_val = np.sin(arr) print(sin_val)
The output result is:
[0. 1. 1.2246468e-16]
- np.exp(): Calculate the exponent value of the elements in the array.
import numpy as np arr = np.array([1, 2, 3]) exp_val = np.exp(arr) print(exp_val)
The output result is:
[ 2.71828183 7.3890561 20.08553692]
This article only shows a small part of the functions in the numpy library, and numpy has more powerful functions and functions. I hope readers can flexibly use the functions of the numpy library in actual programming to improve the efficiency and accuracy of data processing.
The above is the detailed content of Complete list of numpy functions and their uses: Detailed explanation of all functions in the numpy library. For more information, please follow other related articles on the PHP Chinese website!

Hot AI Tools

Undresser.AI Undress
AI-powered app for creating realistic nude photos

AI Clothes Remover
Online AI tool for removing clothes from photos.

Undress AI Tool
Undress images for free

Clothoff.io
AI clothes remover

Video Face Swap
Swap faces in any video effortlessly with our completely free AI face swap tool!

Hot Article

Hot Tools

Notepad++7.3.1
Easy-to-use and free code editor

SublimeText3 Chinese version
Chinese version, very easy to use

Zend Studio 13.0.1
Powerful PHP integrated development environment

Dreamweaver CS6
Visual web development tools

SublimeText3 Mac version
God-level code editing software (SublimeText3)

Hot Topics
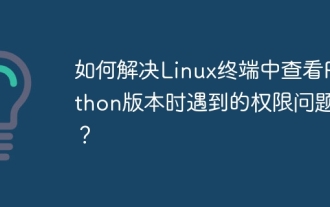
Solution to permission issues when viewing Python version in Linux terminal When you try to view Python version in Linux terminal, enter python...
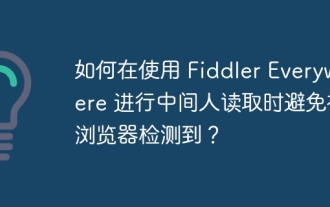
How to avoid being detected when using FiddlerEverywhere for man-in-the-middle readings When you use FiddlerEverywhere...
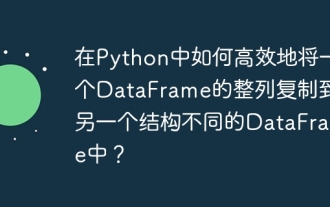
When using Python's pandas library, how to copy whole columns between two DataFrames with different structures is a common problem. Suppose we have two Dats...
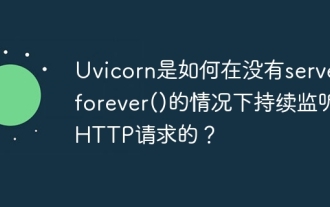
How does Uvicorn continuously listen for HTTP requests? Uvicorn is a lightweight web server based on ASGI. One of its core functions is to listen for HTTP requests and proceed...
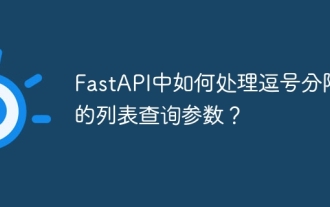
Fastapi ...
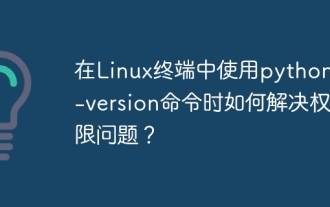
Using python in Linux terminal...
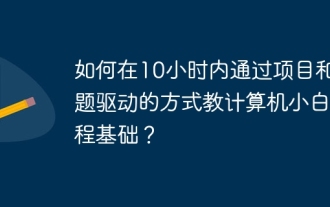
How to teach computer novice programming basics within 10 hours? If you only have 10 hours to teach computer novice some programming knowledge, what would you choose to teach...
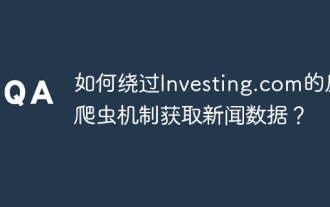
Understanding the anti-crawling strategy of Investing.com Many people often try to crawl news data from Investing.com (https://cn.investing.com/news/latest-news)...
