


How to use Pandas to handle duplicate values in data: a comprehensive analysis of deduplication methods
Comprehensive analysis of Pandas deduplication method: easily handle duplicate values in data, specific code examples are required
Introduction:
In the process of data analysis and processing, It is common to encounter situations where data contains duplicate values. These duplicate values may mislead analysis results or affect the accuracy of the data. Therefore, deduplication is an important part of data processing. As a widely used data processing library in Python, Pandas provides a variety of deduplication methods and can easily handle duplicate values in the data. This article will analyze the commonly used deduplication methods in Pandas and give specific code examples to help readers better understand and apply these methods.
1. drop_duplicates method
The drop_duplicates method is one of the most commonly used deduplication methods in Pandas. It removes duplicate values from data based on specified columns or rows. The specific usage is as follows:
df.drop_duplicates(subset=None, keep='first', inplace=False)
Among them, df represents the data set to be deduplicated, subset is the specified column or row, and the default is None, which means that all columns are deduplicated. The keep parameter indicates which repeated value to keep. The default is 'first', which means to keep the first appearing value. You can also choose 'last', which means to keep the last appearing value. The inplace parameter indicates whether to modify the original data set. The default value is False, which means returning a new deduplicated data set.
Specific example:
Suppose we have a data set df containing duplicate values:
import pandas as pd df = pd.DataFrame({'A': [1, 2, 3, 1, 2, 3], 'B': ['a', 'b', 'c', 'a', 'b', 'c']}) print(df)
The running results are as follows:
A B 0 1 a 1 2 b 2 3 c 3 1 a 4 2 b 5 3 c
We can use the drop_duplicates method to remove duplicate values :
df_drop_duplicates = df.drop_duplicates() print(df_drop_duplicates)
The running results are as follows:
A B 0 1 a 1 2 b 2 3 c
From the results, we can see that the drop_duplicates method successfully deleted duplicate values in the data set.
2. Duplicated method
The duplicated method is another commonly used deduplication method in Pandas. Unlike the drop_duplicates method, the duplicated method returns a Boolean Series to determine whether the elements in each row or column are duplicated. The specific usage is as follows:
df.duplicated(subset=None, keep='first')
Among them, df represents the data set to be duplicated, subset is the specified column or row, and the default is None, which means that all columns are judged. The meaning of the keep parameter is the same as that of the drop_duplicates method.
Specific example:
Assuming we still use the above data set df, we can use the duplicated method to determine whether each row is repeated:
df_duplicated = df.duplicated() print(df_duplicated)
The running results are as follows:
0 False 1 False 2 False 3 True 4 True 5 True dtype: bool
It can be seen from the results that rows 0, 1, and 2 in the returned Series are False, indicating that these rows are not repeated; rows 3, 4, and 5 are True, indicating that these rows are duplicated.
3. Application scenarios of drop_duplicates and duplicated methods
The drop_duplicates and duplicated methods are widely used in data cleaning and data analysis. Common application scenarios include:
- Data deduplication : Delete duplicate values in the data based on specified columns or rows to ensure data accuracy.
- Data analysis: Through deduplication, duplicate samples or observations can be removed to ensure the accuracy of data analysis results.
Specific example:
Suppose we have a sales data set df, containing sales records in multiple cities. We want to count the total sales in each city and remove duplicate cities. We can use the following code to achieve this:
import pandas as pd df = pd.DataFrame({'City': ['Beijing', 'Shanghai', 'Guangzhou', 'Shanghai', 'Beijing'], 'Sales': [1000, 2000, 3000, 1500, 1200]}) df_drop_duplicates = df.drop_duplicates(subset='City') df_total_sales = df.groupby('City')['Sales'].sum() print(df_drop_duplicates) print(df_total_sales)
The running results are as follows:
City Sales 0 Beijing 1000 1 Shanghai 2000 2 Guangzhou 3000 Sales City Beijing 2200 Guangzhou 3000 Shanghai 3500
As can be seen from the results, we first used the drop_duplicates method to remove duplicate cities, and then used the groupby and sum methods to calculate Total sales per city.
Conclusion:
Through the analysis of this article, we understand the usage and application scenarios of the commonly used deduplication methods drop_duplicates and duplicated in Pandas. These methods can help us easily handle duplicate values in the data and ensure the accuracy of data analysis and processing. In practical applications, we can choose appropriate methods according to specific problems and combine them with other Pandas methods for data cleaning and analysis.
Code example:
import pandas as pd df = pd.DataFrame({'A': [1, 2, 3, 1, 2, 3], 'B': ['a', 'b', 'c', 'a', 'b', 'c']}) # 使用drop_duplicates方法去重 df_drop_duplicates = df.drop_duplicates() print(df_drop_duplicates) # 使用duplicated方法判断重复值 df_duplicated = df.duplicated() print(df_duplicated) # 应用场景示例 df = pd.DataFrame({'City': ['Beijing', 'Shanghai', 'Guangzhou', 'Shanghai', 'Beijing'], 'Sales': [1000, 2000, 3000, 1500, 1200]}) df_drop_duplicates = df.drop_duplicates(subset='City') df_total_sales = df.groupby('City')['Sales'].sum() print(df_drop_duplicates) print(df_total_sales)
The above code is run in the Python environment, and the result will be the output of the deduplicated data set and total sales statistics.
References:
- Pandas official documentation: https://pandas.pydata.org/docs/
- "Using Python for Data Analysis" (Second Edition), author: Wes McKinney, People's Posts and Telecommunications Publishing House, 2019.
The above is the detailed content of How to use Pandas to handle duplicate values in data: a comprehensive analysis of deduplication methods. For more information, please follow other related articles on the PHP Chinese website!

Hot AI Tools

Undresser.AI Undress
AI-powered app for creating realistic nude photos

AI Clothes Remover
Online AI tool for removing clothes from photos.

Undress AI Tool
Undress images for free

Clothoff.io
AI clothes remover

Video Face Swap
Swap faces in any video effortlessly with our completely free AI face swap tool!

Hot Article

Hot Tools

Notepad++7.3.1
Easy-to-use and free code editor

SublimeText3 Chinese version
Chinese version, very easy to use

Zend Studio 13.0.1
Powerful PHP integrated development environment

Dreamweaver CS6
Visual web development tools

SublimeText3 Mac version
God-level code editing software (SublimeText3)

Hot Topics
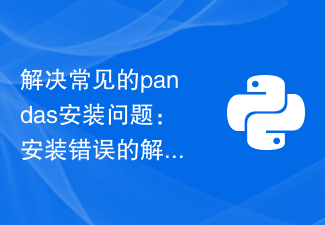
Pandas installation tutorial: Analysis of common installation errors and their solutions, specific code examples are required Introduction: Pandas is a powerful data analysis tool that is widely used in data cleaning, data processing, and data visualization, so it is highly respected in the field of data science . However, due to environment configuration and dependency issues, you may encounter some difficulties and errors when installing pandas. This article will provide you with a pandas installation tutorial and analyze some common installation errors and their solutions. 1. Install pandas
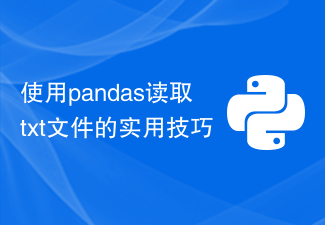
Practical tips for reading txt files using pandas, specific code examples are required. In data analysis and data processing, txt files are a common data format. Using pandas to read txt files allows for fast and convenient data processing. This article will introduce several practical techniques to help you better use pandas to read txt files, along with specific code examples. Reading txt files with delimiters When using pandas to read txt files with delimiters, you can use read_c
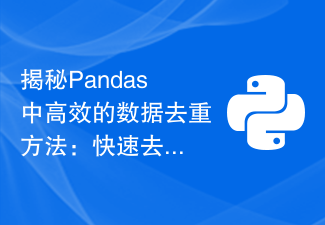
The secret of Pandas deduplication method: a fast and efficient way to deduplicate data, which requires specific code examples. In the process of data analysis and processing, duplication in the data is often encountered. Duplicate data may mislead the analysis results, so deduplication is a very important step. Pandas, a powerful data processing library, provides a variety of methods to achieve data deduplication. This article will introduce some commonly used deduplication methods, and attach specific code examples. The most common case of deduplication based on a single column is based on whether the value of a certain column is duplicated.
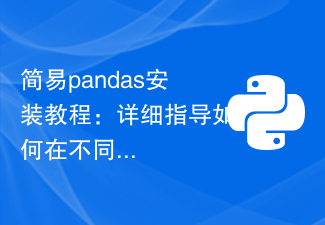
Simple pandas installation tutorial: Detailed guidance on how to install pandas on different operating systems, specific code examples are required. As the demand for data processing and analysis continues to increase, pandas has become one of the preferred tools for many data scientists and analysts. pandas is a powerful data processing and analysis library that can easily process and analyze large amounts of structured data. This article will detail how to install pandas on different operating systems and provide specific code examples. Install on Windows operating system
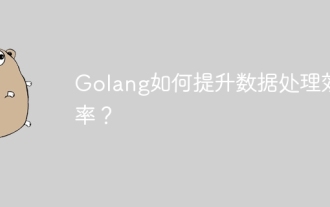
Golang improves data processing efficiency through concurrency, efficient memory management, native data structures and rich third-party libraries. Specific advantages include: Parallel processing: Coroutines support the execution of multiple tasks at the same time. Efficient memory management: The garbage collection mechanism automatically manages memory. Efficient data structures: Data structures such as slices, maps, and channels quickly access and process data. Third-party libraries: covering various data processing libraries such as fasthttp and x/text.
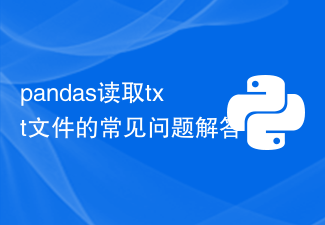
Pandas is a data analysis tool for Python, especially suitable for cleaning, processing and analyzing data. During the data analysis process, we often need to read data files in various formats, such as Txt files. However, some problems will be encountered during the specific operation. This article will introduce answers to common questions about reading txt files with pandas and provide corresponding code examples. Question 1: How to read txt file? txt files can be read using the read_csv() function of pandas. This is because
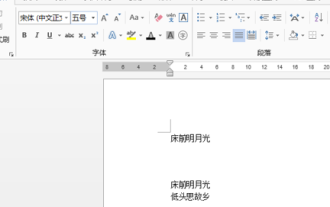
Sometimes when we use word office software to operate and edit files, some content is repeated. How can we quickly find the repeatedly entered information and then delete the repeated content? It is easy to find duplicates in an Excel spreadsheet, but will you find duplicates in a word document? Below, we will share how to remove duplicates in word, so that you can quickly find duplicate content and perform editing operations. First, open a new Word document and enter some content in the document. Consider inserting some repetitive parts to help demonstrate operations. 2. To find duplicate content, we need to click [Start]-[Search] tool in the menu bar, select [Advanced Search] in the drop-down menu, and click
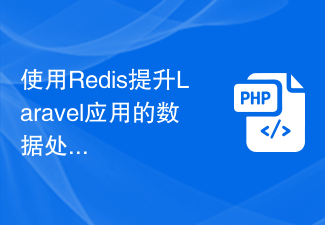
Use Redis to improve the data processing efficiency of Laravel applications. With the continuous development of Internet applications, data processing efficiency has become one of the focuses of developers. When developing applications based on the Laravel framework, we can use Redis to improve data processing efficiency and achieve fast access and caching of data. This article will introduce how to use Redis for data processing in Laravel applications and provide specific code examples. 1. Introduction to Redis Redis is a high-performance memory data
