Introduction to Transformer model application
Transformers is a model that uses a self-attention mechanism, which adopts an encoder-decoder architecture to achieve results. Some common Transformer architecture-based models include BERT and RoBERTa.
The Transformer architecture is specially designed to handle sequence-to-sequence problems in natural language processing tasks. Compared with traditional RNN, LSTM and other architectures, the main advantage of Transformer lies in its unique self-attention mechanism. This mechanism enables Transformer to accurately capture long-range dependencies and correlations between tokens in input sentences and greatly reduces computing time. Through the self-attention mechanism, Transformer can adaptively weight each position in the input sequence to better capture contextual information at different positions. This mechanism makes the Transformer more effective in handling long-distance dependencies, resulting in excellent performance in many natural language processing tasks.
This architecture is based on encoder-decoder and consists of multiple layers of encoders and decoders. Each encoder contains multiple sub-layers, including a multi-head self-attention layer and a positional fully connected feed-forward neural network. Likewise, each decoder also has the same two sub-layers, with the addition of a third sub-layer called the encoder-decoder attention layer, which is applied to the output of the encoder stack.
There is a normalization layer after each sub-layer, and there are residual connections around each feedforward neural network. This residual connection provides a free path for gradient and data flow, helping to avoid vanishing gradient problems when training deep neural networks.
The encoder's attention vector is passed to the feedforward neural network, which converts it into a vector representation and passes it to the next attention layer. The decoder’s task is to transform the encoder’s attention vector into output data. During the training phase, the decoder can use the attention vectors and expected results generated by the encoder.
The decoder uses the same tokenization, word embedding and attention mechanisms to process the expected results and generate attention vectors. This attention vector then interacts with the attention layer in the encoder module to establish the association between the input and output values. The decoder attention vector is processed by the feedforward layer and then mapped into a large vector of the target data size.
The above is the detailed content of Introduction to Transformer model application. For more information, please follow other related articles on the PHP Chinese website!

Hot AI Tools

Undresser.AI Undress
AI-powered app for creating realistic nude photos

AI Clothes Remover
Online AI tool for removing clothes from photos.

Undress AI Tool
Undress images for free

Clothoff.io
AI clothes remover

Video Face Swap
Swap faces in any video effortlessly with our completely free AI face swap tool!

Hot Article

Hot Tools

Notepad++7.3.1
Easy-to-use and free code editor

SublimeText3 Chinese version
Chinese version, very easy to use

Zend Studio 13.0.1
Powerful PHP integrated development environment

Dreamweaver CS6
Visual web development tools

SublimeText3 Mac version
God-level code editing software (SublimeText3)

Hot Topics
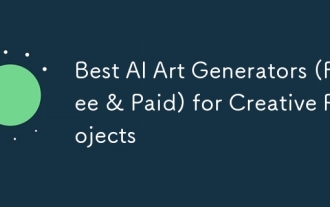
The article reviews top AI art generators, discussing their features, suitability for creative projects, and value. It highlights Midjourney as the best value for professionals and recommends DALL-E 2 for high-quality, customizable art.
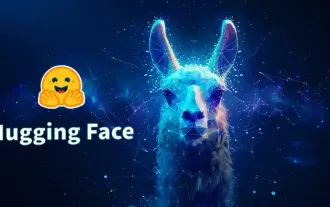
Meta's Llama 3.2: A Leap Forward in Multimodal and Mobile AI Meta recently unveiled Llama 3.2, a significant advancement in AI featuring powerful vision capabilities and lightweight text models optimized for mobile devices. Building on the success o
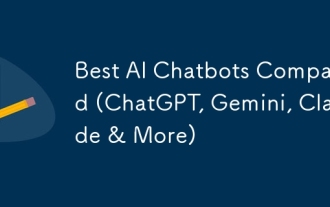
The article compares top AI chatbots like ChatGPT, Gemini, and Claude, focusing on their unique features, customization options, and performance in natural language processing and reliability.
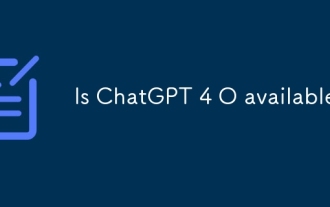
ChatGPT 4 is currently available and widely used, demonstrating significant improvements in understanding context and generating coherent responses compared to its predecessors like ChatGPT 3.5. Future developments may include more personalized interactions and real-time data processing capabilities, further enhancing its potential for various applications.
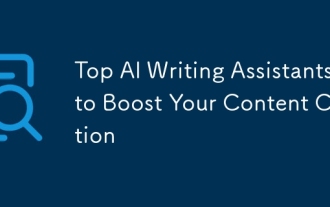
The article discusses top AI writing assistants like Grammarly, Jasper, Copy.ai, Writesonic, and Rytr, focusing on their unique features for content creation. It argues that Jasper excels in SEO optimization, while AI tools help maintain tone consist
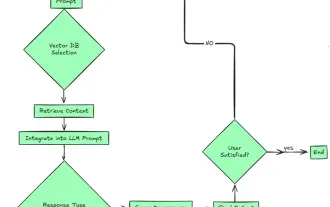
2024 witnessed a shift from simply using LLMs for content generation to understanding their inner workings. This exploration led to the discovery of AI Agents – autonomous systems handling tasks and decisions with minimal human intervention. Buildin
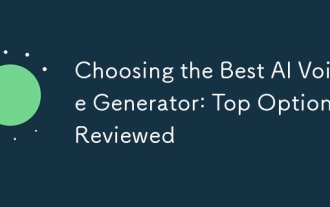
The article reviews top AI voice generators like Google Cloud, Amazon Polly, Microsoft Azure, IBM Watson, and Descript, focusing on their features, voice quality, and suitability for different needs.
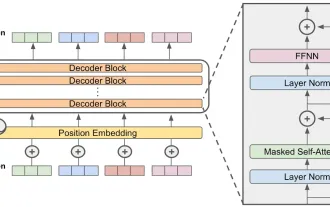
Falcon 3: A Revolutionary Open-Source Large Language Model Falcon 3, the latest iteration in the acclaimed Falcon series of LLMs, represents a significant advancement in AI technology. Developed by the Technology Innovation Institute (TII), this open
