


MoE and Mamba collaborate to scale state-space models to billions of parameters
State Space Model (SSM) is a technology that has attracted much attention and is considered as an alternative to Transformer. Compared with Transformer, SSM can achieve linear time reasoning when processing long context tasks, and has parallel training and excellent performance. In particular, Mamba, which is based on selective SSM and hardware-aware design, has shown outstanding performance and has become one of the powerful alternatives to the attention-based Transformer architecture.
Recently, researchers are also exploring combining SSM and Mamba with other methods to create more powerful architectures. For example, Machine Heart once reported that "Mamba can replace Transformer, but they can also be used in combination."
Recently, a Polish research team discovered that if SSM is combined with a hybrid expert system (MoE/Mixture of Experts), SSM can be expected to achieve large-scale expansion. MoE is a technology commonly used to extend Transformer. For example, the recent Mixtral model uses this technology. Please refer to the Heart of the Machine article.
The research result given by this Polish research team is MoE-Mamba, a model that combines Mamba and a hybrid expert layer.
Paper address: https://arxiv.org/pdf/2401.04081.pdf
MoE -Mamba can improve the efficiency of SSM and MoE at the same time. And the team also found that MoE-Mamba behaved predictably when the number of experts varied.
The team conducted experimental demonstrations. The results showed that compared with Mamba, MoE-Mamba required 2.2 times fewer training steps with the same performance requirements, showing that the new method is comparable. Potential advantages over Transformer and Transformer-MoE. These preliminary results also point to a promising research direction: SSM may be scalable to tens of billions of parameters.
Related research
##State space model
State Space Model (SSM) is a type of architecture used for sequence modeling. The ideas for these models originate from the field of cybernetics and can be viewed as a combination of RNN and CNN. Although they have considerable advantages, they also have some problems that prevent them from becoming the dominant architecture for language modeling tasks. However, recent research breakthroughs have allowed deep SSM to scale to billions of parameters while maintaining computational efficiency and strong performance.
Mamba
Mamba is a model built based on SSM, which can achieve linear time reasoning speed (for context length ), and it also achieves an efficient training process through hardware-aware design. Mamba uses a work-efficient parallel scan approach that mitigates the impact of loop sequentiality, while fused GPU operations eliminate the need to implement extended state. Intermediate states necessary for backpropagation are not saved but are recomputed during the backward pass, thereby reducing memory requirements. The advantage of Mamba over the attention mechanism is particularly significant in the inference stage because it not only reduces computational complexity, but also the memory usage does not depend on the context length.
Mamba solves the fundamental trade-off between efficiency and effectiveness of sequence models, which highlights the importance of state compression. An efficient model must have a small state, and an effective model must have a state that contains all the key information of the context. Unlike other SSMs that require temporal and input invariance, Mamba introduces a selection mechanism that controls how information is propagated along the sequence dimension. This design choice was inspired by an intuitive understanding of first-class synthesis tasks such as selective replication and induction, allowing the model to discern and retain critical information while filtering out irrelevant information.
Research has found that Mamba has the ability to efficiently utilize longer contexts (up to 1M tokens), and as the context length increases, the pre-training perplexity will also improve. The Mamba model is composed of stacked Mamba blocks and has achieved very good results in many different fields such as NLP, genomics, audio, etc. Its performance is comparable to and surpasses the existing Transformer model. Therefore, Mamba has become a strong candidate model for the general sequence modeling backbone model. Please refer to "Five times throughput, performance fully surrounds Transformer: New architecture Mamba detonates the AI circle 》.
Hybrid Expert
Mixed Expert (MoE) technology can greatly increase the number of parameters of the model, and at the same time Does not affect FLOPs required for model inference and training. MoE was first proposed by Jacobs et al. in 1991 and started to be used for NLP tasks in 2017 by Shazeer et al.
MoE has an advantage: the activations are very sparse - for each token processed, only a small part of the parameters of the model are used. Due to its computational requirements, the forward layer in the Transformer has become a standard target for several MoE techniques.
The research community has proposed various methods to solve the core problem of MoE, which is the process of assigning tokens to experts, also known as the routing process. There are currently two basic routing algorithms: Token Choice and Expert Choice. The former is to route each token to a certain number (K) of experts, while the latter is to route each token to a fixed number of experts.
Fedus et al. proposed Switch in the 2022 paper "Switch transformers: Scaling to trillion parameter models with simple and efficient sparsity". It is a Token Choice architecture that combines Each token is routed to a single expert (K=1), and they used this method to successfully expand the Transformer parameter size to 1.6 trillion. This team in Poland also used this MoE design in their experiments.
Recently, MoE has also begun to enter the open source community, such as OpenMoE.
Project address: https://github.com/XueFuzhao/OpenMoE
Particularly worth mentioning is Mistral’s open source Mixtral 8× 7B, its performance is comparable to LLaMa 2 70B, while the required inference computing budget is only about one-sixth of the latter.
Model Architecture
Although the main underlying mechanism of Mamba is quite different from the attention mechanism used in Transformer, Mamba retains the Transformer model high-level, module-based structure. Using this paradigm, one or more layers of identical modules are stacked on top of each other, and the output of each layer is added to a residual stream, see Figure 2. The final value of this residual stream is then used to predict the next token for the language modeling task.
MoE-Mamba takes advantage of the compatibility of both architectures. As shown in Figure 2, in MoE-Mamba, every interval Mamba layer is replaced by a Switch-based MoE feedforward layer.
However, the team also noticed that this design is somewhat similar to the design of "Mamba: Linear-time sequence modeling with selective state spaces"; later The model alternately stacks Mamba layers and feedforward layers, but the resulting model is slightly inferior to pure Mamba. This design is denoted as Mamba-MLP in Figure 1 .
MoE-Mamba separates the unconditional processing of each token performed by the Mamba layer and the conditional processing performed by the MoE layer; the unconditional processing can efficiently integrate the entire context of the sequence into An internal representation, while conditional processing can use the most relevant experts for each token. This idea of alternating conditional and unconditional processing has been applied in some MoE-based models, but they usually alternate basic and MoE feedforward layers.
Key results
Training settings
The team compared 5 different settings: Basic Transformer, Mamba, Mamba-MLP, MoE and MoE-Mamba.
In most Transformers, the feedforward layer contains 8dm² parameters, while the Mamba paper makes Mamba smaller (about 6dm²), so that the number of parameters of two Mamba layers is the same as that of one feedforward layer and one The attention layers add up to about the same. To get roughly the same number of active parameters per token in Mamba and the new model, the team reduced the size of each expert forward layer to 6dm². Except for the embedding and unembedding layers, all models use approximately 26 million parameters per token. The training process uses 6.5 billion tokens and the number of training steps is 100k.
The data set used for training is the English C4 data set, and the task is to predict the next token. Text is tokenized using the GPT2 tokenizer. Table 3 gives the complete list of hyperparameters.
Results
Table 1 gives the training results. MoE-Mamba performs significantly better than the regular Mamba model.
Notably, MoE-Mamba achieved the same level of results as regular Mamba in just 46% of the training steps. Since the learning rate is adjusted for ordinary Mamba, it can be expected that if the training process is optimized for MoE-Mamba, MoE-Mamba will perform better.
Ablation Study
#To evaluate whether Mamba scales well as the number of experts grows, the researchers compared using different numbers of experts model.
Figure 3 shows the training run steps when using different numbers of experts.
Table 2 gives the results after 100k steps.
#These results show that the newly proposed method scales well with the number of experts. If the number of experts is 8 or more, the final performance of the new model is better than the normal Mamba. Since Mamba-MLP is worse than plain Mamba, it can be expected that MoE-Mamba using a small number of experts will perform worse than Mamba. The new method gave the best results when the number of experts was 32.
The above is the detailed content of MoE and Mamba collaborate to scale state-space models to billions of parameters. For more information, please follow other related articles on the PHP Chinese website!

Hot AI Tools

Undresser.AI Undress
AI-powered app for creating realistic nude photos

AI Clothes Remover
Online AI tool for removing clothes from photos.

Undress AI Tool
Undress images for free

Clothoff.io
AI clothes remover

Video Face Swap
Swap faces in any video effortlessly with our completely free AI face swap tool!

Hot Article

Hot Tools

Notepad++7.3.1
Easy-to-use and free code editor

SublimeText3 Chinese version
Chinese version, very easy to use

Zend Studio 13.0.1
Powerful PHP integrated development environment

Dreamweaver CS6
Visual web development tools

SublimeText3 Mac version
God-level code editing software (SublimeText3)

Hot Topics
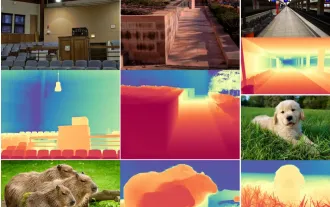
0.What does this article do? We propose DepthFM: a versatile and fast state-of-the-art generative monocular depth estimation model. In addition to traditional depth estimation tasks, DepthFM also demonstrates state-of-the-art capabilities in downstream tasks such as depth inpainting. DepthFM is efficient and can synthesize depth maps within a few inference steps. Let’s read about this work together ~ 1. Paper information title: DepthFM: FastMonocularDepthEstimationwithFlowMatching Author: MingGui, JohannesS.Fischer, UlrichPrestel, PingchuanMa, Dmytr
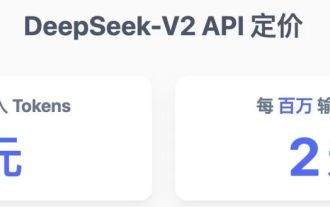
Imagine an artificial intelligence model that not only has the ability to surpass traditional computing, but also achieves more efficient performance at a lower cost. This is not science fiction, DeepSeek-V2[1], the world’s most powerful open source MoE model is here. DeepSeek-V2 is a powerful mixture of experts (MoE) language model with the characteristics of economical training and efficient inference. It consists of 236B parameters, 21B of which are used to activate each marker. Compared with DeepSeek67B, DeepSeek-V2 has stronger performance, while saving 42.5% of training costs, reducing KV cache by 93.3%, and increasing the maximum generation throughput to 5.76 times. DeepSeek is a company exploring general artificial intelligence
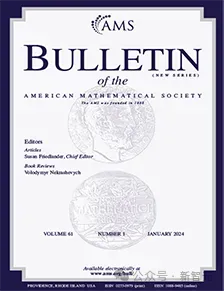
AI is indeed changing mathematics. Recently, Tao Zhexuan, who has been paying close attention to this issue, forwarded the latest issue of "Bulletin of the American Mathematical Society" (Bulletin of the American Mathematical Society). Focusing on the topic "Will machines change mathematics?", many mathematicians expressed their opinions. The whole process was full of sparks, hardcore and exciting. The author has a strong lineup, including Fields Medal winner Akshay Venkatesh, Chinese mathematician Zheng Lejun, NYU computer scientist Ernest Davis and many other well-known scholars in the industry. The world of AI has changed dramatically. You know, many of these articles were submitted a year ago.
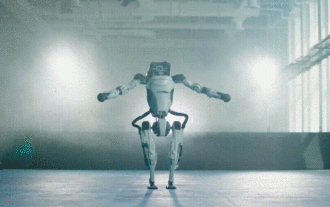
Boston Dynamics Atlas officially enters the era of electric robots! Yesterday, the hydraulic Atlas just "tearfully" withdrew from the stage of history. Today, Boston Dynamics announced that the electric Atlas is on the job. It seems that in the field of commercial humanoid robots, Boston Dynamics is determined to compete with Tesla. After the new video was released, it had already been viewed by more than one million people in just ten hours. The old people leave and new roles appear. This is a historical necessity. There is no doubt that this year is the explosive year of humanoid robots. Netizens commented: The advancement of robots has made this year's opening ceremony look like a human, and the degree of freedom is far greater than that of humans. But is this really not a horror movie? At the beginning of the video, Atlas is lying calmly on the ground, seemingly on his back. What follows is jaw-dropping
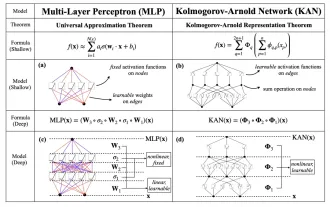
Earlier this month, researchers from MIT and other institutions proposed a very promising alternative to MLP - KAN. KAN outperforms MLP in terms of accuracy and interpretability. And it can outperform MLP running with a larger number of parameters with a very small number of parameters. For example, the authors stated that they used KAN to reproduce DeepMind's results with a smaller network and a higher degree of automation. Specifically, DeepMind's MLP has about 300,000 parameters, while KAN only has about 200 parameters. KAN has a strong mathematical foundation like MLP. MLP is based on the universal approximation theorem, while KAN is based on the Kolmogorov-Arnold representation theorem. As shown in the figure below, KAN has
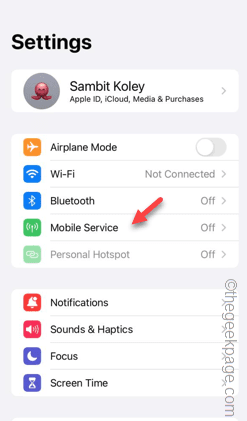
Facing lag, slow mobile data connection on iPhone? Typically, the strength of cellular internet on your phone depends on several factors such as region, cellular network type, roaming type, etc. There are some things you can do to get a faster, more reliable cellular Internet connection. Fix 1 – Force Restart iPhone Sometimes, force restarting your device just resets a lot of things, including the cellular connection. Step 1 – Just press the volume up key once and release. Next, press the Volume Down key and release it again. Step 2 – The next part of the process is to hold the button on the right side. Let the iPhone finish restarting. Enable cellular data and check network speed. Check again Fix 2 – Change data mode While 5G offers better network speeds, it works better when the signal is weaker
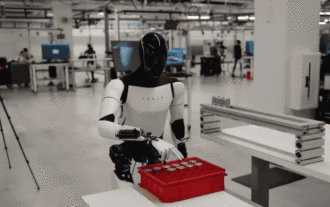
The latest video of Tesla's robot Optimus is released, and it can already work in the factory. At normal speed, it sorts batteries (Tesla's 4680 batteries) like this: The official also released what it looks like at 20x speed - on a small "workstation", picking and picking and picking: This time it is released One of the highlights of the video is that Optimus completes this work in the factory, completely autonomously, without human intervention throughout the process. And from the perspective of Optimus, it can also pick up and place the crooked battery, focusing on automatic error correction: Regarding Optimus's hand, NVIDIA scientist Jim Fan gave a high evaluation: Optimus's hand is the world's five-fingered robot. One of the most dexterous. Its hands are not only tactile
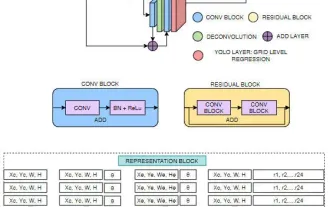
Target detection is a relatively mature problem in autonomous driving systems, among which pedestrian detection is one of the earliest algorithms to be deployed. Very comprehensive research has been carried out in most papers. However, distance perception using fisheye cameras for surround view is relatively less studied. Due to large radial distortion, standard bounding box representation is difficult to implement in fisheye cameras. To alleviate the above description, we explore extended bounding box, ellipse, and general polygon designs into polar/angular representations and define an instance segmentation mIOU metric to analyze these representations. The proposed model fisheyeDetNet with polygonal shape outperforms other models and simultaneously achieves 49.5% mAP on the Valeo fisheye camera dataset for autonomous driving
