


Comparing Golang and Python crawlers: analysis of differences in anti-crawling, data processing and framework selection
In-depth exploration of the similarities and differences between Golang crawlers and Python crawlers: anti-crawling response, data processing and framework selection
Introduction:
In recent years, with the development of the Internet With rapid development, the amount of data on the network has exploded. As a technical means to obtain Internet data, crawlers have attracted the attention of developers. The two mainstream languages, Golang and Python, each have their own advantages and characteristics. This article will delve into the similarities and differences between Golang crawlers and Python crawlers, including anti-crawling responses, data processing, and framework selection.
1. Anti-crawling response
Anti-crawling technology is an important challenge that web crawlers must face. As a popular scripting language, Python has a wealth of third-party libraries and frameworks, providing various anti-crawling solutions. For example, selenium can be used to simulate browser operations and dynamically load data, bypassing the website's JavaScript. In addition, Python's request library also provides Cookie and User-Agent settings, which can be disguised as different browsers for access, increasing concealment. By processing the request header information, the anti-crawling mechanism of the website can be effectively circumvented.
Different from this, Golang is an emerging statically typed language, and developers need more manual processing during the crawling process. Although there are not as rich third-party libraries as Python, Golang's strongly typed language features can provide better performance and concurrency support. The anti-crawling solution mainly uses HTTP request packages such as "requests", "http", etc. to manually set request headers, cookies, User-Agent and other information. In addition, Golang also provides rich concurrent programming mechanisms, such as goroutine and channel, making it easier to crawl multiple pages at the same time.
To sum up, Python is more convenient and faster in anti-crawling, while Golang is more flexible and efficient.
2. Data processing
Data processing is a key link in the crawler process. Python has a wealth of data processing libraries and tools, such as BeautifulSoup, pandas, and numpy. Through these libraries, we can easily parse and process HTML, XML and other documents, extract the required data, and perform various complex data analysis, cleaning and visualization operations. In addition, Python also supports various databases, such as MySQL, MongoDB, etc., to facilitate the storage and query of crawled data.
On the contrary, Golang is relatively simple in data processing. Although Golang also has similar libraries, such as goquery and gocsv, its ecosystem and third-party library support are weaker than Python. Therefore, Golang usually needs to write its own code for parsing, processing and storage in data processing.
Overall, Python is more convenient and powerful in data processing, while Golang requires more code writing and processing.
3. Framework selection
The choice of framework has an important impact on the development efficiency and performance of the crawler. In Python, there are many mature frameworks to choose from, such as Scrapy and PySpider. These frameworks provide automated crawler processes and task scheduling, reducing developers' workload. At the same time, they also provide powerful data processing capabilities and concurrency capabilities.
Golang is relatively new when it comes to crawler frameworks, but there are some good options. For example, colly is a feature-rich and highly configurable crawler framework that provides powerful concurrency and data processing capabilities. In addition, libraries such as gocolly and go-crawler also provide similar functions.
To sum up, Python has more mature and rich choices in crawler frameworks, while Golang has relatively few frameworks, but there are already many potential options.
Conclusion:
This article deeply explores the similarities and differences between Golang crawlers and Python crawlers in terms of anti-crawling response, data processing and framework selection. Overall, Python is more convenient and powerful in anti-crawling and data processing, while Golang is more flexible and efficient. In terms of framework selection, Python has more mature choices, while Golang has relatively few. Developers can choose appropriate languages and frameworks based on specific needs and project characteristics to achieve efficient crawler development.
Although this article provides some code and examples, due to space limitations, it is impossible to show all code implementations in detail. We hope that readers can use the introduction and ideas of this article to deeply study and practice the development of Golang and Python crawlers, and further explore the application and development of these two languages in the field of Internet data acquisition.
The above is the detailed content of Comparing Golang and Python crawlers: analysis of differences in anti-crawling, data processing and framework selection. For more information, please follow other related articles on the PHP Chinese website!

Hot AI Tools

Undresser.AI Undress
AI-powered app for creating realistic nude photos

AI Clothes Remover
Online AI tool for removing clothes from photos.

Undress AI Tool
Undress images for free

Clothoff.io
AI clothes remover

Video Face Swap
Swap faces in any video effortlessly with our completely free AI face swap tool!

Hot Article

Hot Tools

Notepad++7.3.1
Easy-to-use and free code editor

SublimeText3 Chinese version
Chinese version, very easy to use

Zend Studio 13.0.1
Powerful PHP integrated development environment

Dreamweaver CS6
Visual web development tools

SublimeText3 Mac version
God-level code editing software (SublimeText3)

Hot Topics
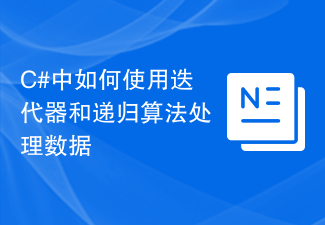
How to use iterators and recursive algorithms to process data in C# requires specific code examples. In C#, iterators and recursive algorithms are two commonly used data processing methods. Iterators can help us traverse the elements in a collection, and recursive algorithms can handle complex problems efficiently. This article details how to use iterators and recursive algorithms to process data, and provides specific code examples. Using Iterators to Process Data In C#, we can use iterators to iterate over the elements in a collection without knowing the size of the collection in advance. Through the iterator, I
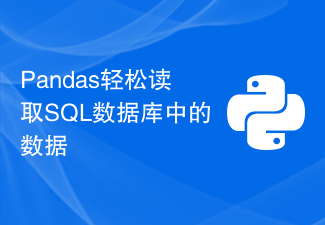
Data processing tool: Pandas reads data in SQL databases and requires specific code examples. As the amount of data continues to grow and its complexity increases, data processing has become an important part of modern society. In the data processing process, Pandas has become one of the preferred tools for many data analysts and scientists. This article will introduce how to use the Pandas library to read data from a SQL database and provide some specific code examples. Pandas is a powerful data processing and analysis tool based on Python
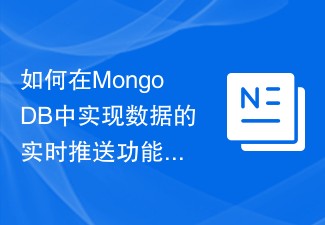
How to implement real-time data push function in MongoDB MongoDB is a document-oriented NoSQL database, which is characterized by high scalability and flexible data model. In some application scenarios, we need to push data updates to the client in real time in order to update the interface or perform corresponding operations in a timely manner. This article will introduce how to implement the real-time push function of data in MongoDB and give specific code examples. There are many ways to implement real-time push functionality, such as using polling, long polling, Web
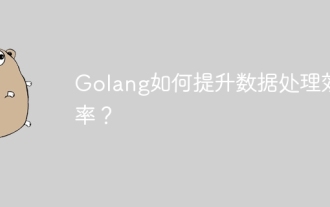
Golang improves data processing efficiency through concurrency, efficient memory management, native data structures and rich third-party libraries. Specific advantages include: Parallel processing: Coroutines support the execution of multiple tasks at the same time. Efficient memory management: The garbage collection mechanism automatically manages memory. Efficient data structures: Data structures such as slices, maps, and channels quickly access and process data. Third-party libraries: covering various data processing libraries such as fasthttp and x/text.
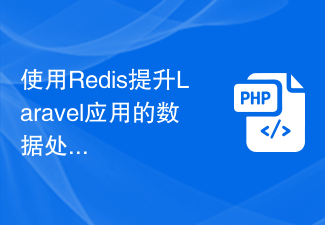
Use Redis to improve the data processing efficiency of Laravel applications. With the continuous development of Internet applications, data processing efficiency has become one of the focuses of developers. When developing applications based on the Laravel framework, we can use Redis to improve data processing efficiency and achieve fast access and caching of data. This article will introduce how to use Redis for data processing in Laravel applications and provide specific code examples. 1. Introduction to Redis Redis is a high-performance memory data
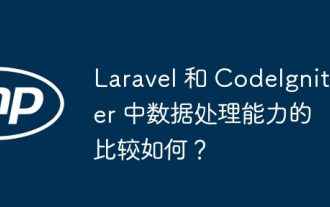
Compare the data processing capabilities of Laravel and CodeIgniter: ORM: Laravel uses EloquentORM, which provides class-object relational mapping, while CodeIgniter uses ActiveRecord to represent the database model as a subclass of PHP classes. Query builder: Laravel has a flexible chained query API, while CodeIgniter’s query builder is simpler and array-based. Data validation: Laravel provides a Validator class that supports custom validation rules, while CodeIgniter has less built-in validation functions and requires manual coding of custom rules. Practical case: User registration example shows Lar
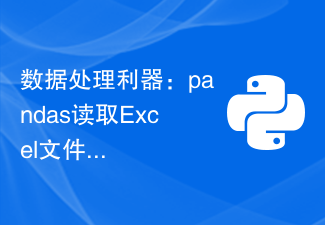
With the increasing popularity of data processing, more and more people are paying attention to how to use data efficiently and make the data work for themselves. In daily data processing, Excel tables are undoubtedly the most common data format. However, when a large amount of data needs to be processed, manually operating Excel will obviously become very time-consuming and laborious. Therefore, this article will introduce an efficient data processing tool - pandas, and how to use this tool to quickly read Excel files and perform data processing. 1. Introduction to pandas pandas
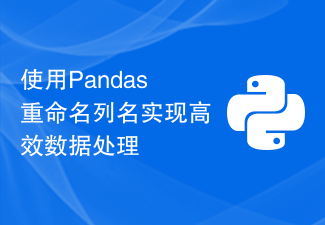
Efficient data processing: Using Pandas to modify column names requires specific code examples. Data processing is a very important part of data analysis, and during the data processing process, it is often necessary to modify the column names of the data. Pandas is a powerful data processing library that provides a wealth of methods and functions to help us process data quickly and efficiently. This article will introduce how to use Pandas to modify column names and provide specific code examples. In actual data analysis, the column names of the original data may have inconsistent naming standards and are difficult to understand.
