


The innovative 'meta-tip' strategy of the Byte Fudan team has improved the performance of diffusion model image understanding, reaching an unprecedented level!
Text-to-image (T2I) diffusion model excels in generating high-definition images thanks to its pre-training on large-scale image-text pairs.
This raises a natural question: Can diffusion models be used to solve visual perception tasks?
Recently, teams from ByteDance and Fudan University proposed a diffusion model to handle visual tasks.
Paper address: https://arxiv.org/abs/2312.14733
Open source project: https://github.com/fudan-zvg/meta-prompts
The team’s key insight is to introduce learnable meta-prompts into the pre-trained diffusion model to extract suitable Characteristics of specific perceptual tasks.
Technical Introduction
The team applies the text-to-image diffusion model as a feature extractor to visual perception tasks.
First, the input image is compressed by the VQVAE encoder, the resolution is reduced to 1/8 of the original size, and a latent space feature representation is generated. It is worth noting that the VQVAE encoder parameters are fixed and do not participate in subsequent training.
Next step, send the data without noise to UNet for feature extraction. To better adapt to different tasks, UNet receives modulated time-step embeddings and multiple meta-cues simultaneously to generate shape-consistent features.
During the entire process, in order to enhance feature expression, this method performs repeated refinement. This enables better interactive fusion of features from different layers within UNet. In the second cycle, the parameters of UNet are adjusted by specific learnable temporal modulation features.
Finally, the multi-scale features generated by UNet are input into a decoder specifically designed for the target vision task.
Learnable meta prompts design
Stable The diffusion model adopts the UNet architecture and integrates text cues into image features through cross-attention to achieve a Vincentian graph. This integration ensures that image generation is contextually and semantically accurate.
However, the diversity of visual perception tasks goes beyond this scope, as image understanding faces different challenges and often lacks textual information as guidance, making text-driven methods sometimes unrealistic.
To address this challenge, the technical team’s approach adopts a more diverse strategy—rather than relying on external text cues, we design an internal learnable meta-cue. Known as meta prompts, these meta prompts are integrated into diffusion models to adapt to perceptual tasks.
Meta prompts are expressed in the form of a matrix, which represents the number of meta prompts and represents the dimension. Perceptual diffusion models with meta prompts avoid the need for external text prompts, such as dataset category labels or image titles, and do not require a pre-trained text encoder to generate the final text prompts.
Meta prompts can be trained end-to-end according to the target task and data set, thereby establishing specially customized adaptation conditions for denoising UNet. These meta prompts contain rich semantic information adapted to specific tasks. For example:
- In the semantic segmentation task, meta prompts effectively demonstrate the ability to identify categories, and the same meta prompts tend to activate features of the same category .
- In the depth estimation task, meta prompts show the ability to perceive depth, and the activation value changes with depth. Enables prompts to focus on objects at a consistent distance.
- In pose estimation, meta prompts exhibit a different set of capabilities, especially the perception of key points, which facilitates human pose detection.
#These qualitative results together highlight the effectiveness of the meta prompts proposed by the technical team in activating task-related abilities in various tasks.
As an alternative to text prompts, meta prompts well fill the gap between text-to-image diffusion models and visual perception tasks.
Feature reorganization based on meta-cues
The diffusion model is generated in denoising UNet through its inherent design Multi-scale features that focus on finer, lower-level details closer to the output layer.
While this low-level detail is sufficient for tasks that emphasize texture and fine-grainedness, visual perception tasks often require understanding content that includes both low-level detail and high-level semantic interpretation.
Therefore, not only does it need to generate rich features, it is also very important to determine which combination of these multi-scale features can provide the best representation for the current task.
This is where meta prompts come in -
These prompts hold context during training that is specific to the dataset used Knowledge. This contextual knowledge enables meta prompts to act as filters for feature recombination, guiding the feature selection process and filtering out the most relevant features for the task from the many features generated by UNet.
The team uses a dot product approach to combine the richness of multi-scale features of UNet with the task adaptability of meta prompts.
Consider multi-scale features, each of which. and represent the height and width of the feature map. Meta prompts. The rearranged features at each scale are calculated as:
Finally, these features filtered by meta prompts are then input into a task-specific decoder.
Recurrent refinement based on learnable temporal modulation features
In the diffusion model, add noise and then multi-step The iterative process of denoising forms the framework for image generation.
Inspired by this mechanism, the technical team designed a simple recurrent refinement process for visual perception tasks—without adding noise to the output features, but directly adding the output features of UNet Loop input into UNet.
At the same time, in order to solve the inconsistency problem that as the model passes through the loop, the distribution of input features changes but the parameters of UNet remain unchanged, the technical team introduced learnable for each loop Unique timestep embeddings to modulate UNet's parameters.
This ensures that the network remains adaptable and responsive to the variability of input features in different steps, optimizing the feature extraction process and enhancing the model's performance in visual recognition tasks.
The results show that this method has achieved optimal results on multiple perception task data sets.
The methods and technologies proposed in this article have broad application prospects and can promote technological development and innovation in multiple fields:
- Improvement of visual perception tasks: This research can improve the performance of various visual perception tasks, such as image segmentation, depth estimation and pose estimation. These improvements can be applied to fields such as autonomous driving, medical image analysis, and robot vision systems.
- Enhanced Computer Vision Model: The proposed technology can make computer vision models more accurate and efficient in processing complex scenes, especially in the absence of explicit text descriptions in the case of. This is particularly important for applications such as image content understanding.
- Cross-field applications: The methods and findings of this study can inspire cross-field research and applications, such as in art creation, virtual reality, and augmented reality. To improve the quality and interactivity of images and videos.
- Long-term outlook: As technology advances, these methods may be further improved, bringing more advanced image generation and content understanding technology.
Team Introduction
The intelligent creation team is ByteDance’s AI & multimedia technology center, covering computer vision, audio and video editing, special effects processing and other technical fields , with the help of the company's rich business scenarios, infrastructure resources and technical collaboration atmosphere, it has realized a closed loop of cutting-edge algorithms-engineering systems-products, aiming to provide the company's internal businesses with cutting-edge content understanding and content in various forms. Capabilities and industry solutions for creation, interactive experience and consumption.
Currently, the intelligent creation team has opened its technical capabilities and services to enterprises through Volcano Engine, a cloud service platform owned by ByteDance. More positions related to large model algorithms are open, please click 「Read the original text」 to view.
The above is the detailed content of The innovative 'meta-tip' strategy of the Byte Fudan team has improved the performance of diffusion model image understanding, reaching an unprecedented level!. For more information, please follow other related articles on the PHP Chinese website!

Hot AI Tools

Undresser.AI Undress
AI-powered app for creating realistic nude photos

AI Clothes Remover
Online AI tool for removing clothes from photos.

Undress AI Tool
Undress images for free

Clothoff.io
AI clothes remover

Video Face Swap
Swap faces in any video effortlessly with our completely free AI face swap tool!

Hot Article

Hot Tools

Notepad++7.3.1
Easy-to-use and free code editor

SublimeText3 Chinese version
Chinese version, very easy to use

Zend Studio 13.0.1
Powerful PHP integrated development environment

Dreamweaver CS6
Visual web development tools

SublimeText3 Mac version
God-level code editing software (SublimeText3)

Hot Topics
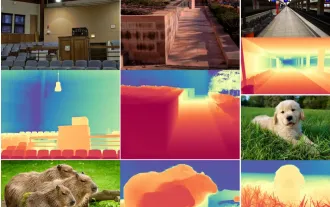
0.What does this article do? We propose DepthFM: a versatile and fast state-of-the-art generative monocular depth estimation model. In addition to traditional depth estimation tasks, DepthFM also demonstrates state-of-the-art capabilities in downstream tasks such as depth inpainting. DepthFM is efficient and can synthesize depth maps within a few inference steps. Let’s read about this work together ~ 1. Paper information title: DepthFM: FastMonocularDepthEstimationwithFlowMatching Author: MingGui, JohannesS.Fischer, UlrichPrestel, PingchuanMa, Dmytr
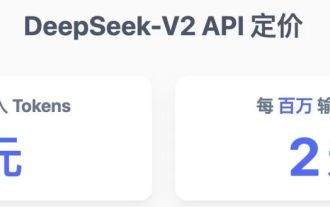
Imagine an artificial intelligence model that not only has the ability to surpass traditional computing, but also achieves more efficient performance at a lower cost. This is not science fiction, DeepSeek-V2[1], the world’s most powerful open source MoE model is here. DeepSeek-V2 is a powerful mixture of experts (MoE) language model with the characteristics of economical training and efficient inference. It consists of 236B parameters, 21B of which are used to activate each marker. Compared with DeepSeek67B, DeepSeek-V2 has stronger performance, while saving 42.5% of training costs, reducing KV cache by 93.3%, and increasing the maximum generation throughput to 5.76 times. DeepSeek is a company exploring general artificial intelligence
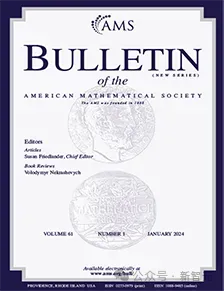
AI is indeed changing mathematics. Recently, Tao Zhexuan, who has been paying close attention to this issue, forwarded the latest issue of "Bulletin of the American Mathematical Society" (Bulletin of the American Mathematical Society). Focusing on the topic "Will machines change mathematics?", many mathematicians expressed their opinions. The whole process was full of sparks, hardcore and exciting. The author has a strong lineup, including Fields Medal winner Akshay Venkatesh, Chinese mathematician Zheng Lejun, NYU computer scientist Ernest Davis and many other well-known scholars in the industry. The world of AI has changed dramatically. You know, many of these articles were submitted a year ago.
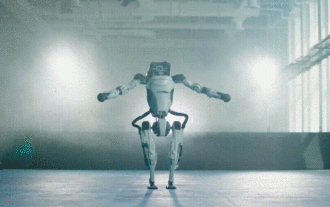
Boston Dynamics Atlas officially enters the era of electric robots! Yesterday, the hydraulic Atlas just "tearfully" withdrew from the stage of history. Today, Boston Dynamics announced that the electric Atlas is on the job. It seems that in the field of commercial humanoid robots, Boston Dynamics is determined to compete with Tesla. After the new video was released, it had already been viewed by more than one million people in just ten hours. The old people leave and new roles appear. This is a historical necessity. There is no doubt that this year is the explosive year of humanoid robots. Netizens commented: The advancement of robots has made this year's opening ceremony look like a human, and the degree of freedom is far greater than that of humans. But is this really not a horror movie? At the beginning of the video, Atlas is lying calmly on the ground, seemingly on his back. What follows is jaw-dropping
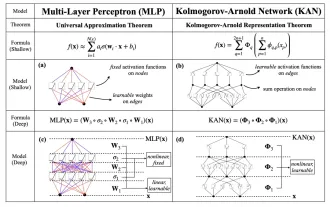
Earlier this month, researchers from MIT and other institutions proposed a very promising alternative to MLP - KAN. KAN outperforms MLP in terms of accuracy and interpretability. And it can outperform MLP running with a larger number of parameters with a very small number of parameters. For example, the authors stated that they used KAN to reproduce DeepMind's results with a smaller network and a higher degree of automation. Specifically, DeepMind's MLP has about 300,000 parameters, while KAN only has about 200 parameters. KAN has a strong mathematical foundation like MLP. MLP is based on the universal approximation theorem, while KAN is based on the Kolmogorov-Arnold representation theorem. As shown in the figure below, KAN has
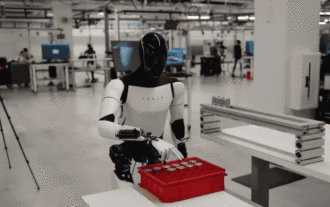
The latest video of Tesla's robot Optimus is released, and it can already work in the factory. At normal speed, it sorts batteries (Tesla's 4680 batteries) like this: The official also released what it looks like at 20x speed - on a small "workstation", picking and picking and picking: This time it is released One of the highlights of the video is that Optimus completes this work in the factory, completely autonomously, without human intervention throughout the process. And from the perspective of Optimus, it can also pick up and place the crooked battery, focusing on automatic error correction: Regarding Optimus's hand, NVIDIA scientist Jim Fan gave a high evaluation: Optimus's hand is the world's five-fingered robot. One of the most dexterous. Its hands are not only tactile
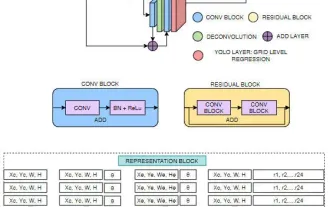
Target detection is a relatively mature problem in autonomous driving systems, among which pedestrian detection is one of the earliest algorithms to be deployed. Very comprehensive research has been carried out in most papers. However, distance perception using fisheye cameras for surround view is relatively less studied. Due to large radial distortion, standard bounding box representation is difficult to implement in fisheye cameras. To alleviate the above description, we explore extended bounding box, ellipse, and general polygon designs into polar/angular representations and define an instance segmentation mIOU metric to analyze these representations. The proposed model fisheyeDetNet with polygonal shape outperforms other models and simultaneously achieves 49.5% mAP on the Valeo fisheye camera dataset for autonomous driving
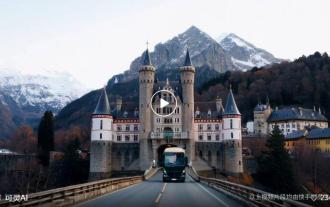
What? Is Zootopia brought into reality by domestic AI? Exposed together with the video is a new large-scale domestic video generation model called "Keling". Sora uses a similar technical route and combines a number of self-developed technological innovations to produce videos that not only have large and reasonable movements, but also simulate the characteristics of the physical world and have strong conceptual combination capabilities and imagination. According to the data, Keling supports the generation of ultra-long videos of up to 2 minutes at 30fps, with resolutions up to 1080p, and supports multiple aspect ratios. Another important point is that Keling is not a demo or video result demonstration released by the laboratory, but a product-level application launched by Kuaishou, a leading player in the short video field. Moreover, the main focus is to be pragmatic, not to write blank checks, and to go online as soon as it is released. The large model of Ke Ling is already available in Kuaiying.
