


Shanghai Jiao Tong University team develops data-driven active learning framework to accelerate research progress in carbon nanomaterials
Editor| .
Although the controlled synthesis of substrate-catalyzed growth into carbon nanostructures is considered a promising approach, there are still challenges in dynamic catalytic surface growth mechanisms and design strategies that require further research and development.
Recently, a research team from Shanghai Jiao Tong University and Tohoku University in Japan demonstrated the effectiveness of an active machine learning model in revealing the microscopic process of substrate catalytic growth. Through the collaborative application of molecular dynamics and Monte Carlo methods, they successfully performed a comprehensive dynamic simulation of the growth of graphene on Cu(111). To enhance the accuracy of the simulation, the research team adopted a Gaussian approximation potential. This research provides new tools and methods for in-depth understanding of the catalytic growth process.
Through this research, we derived a practical and effective method that can be used to design metal or alloy substrates to obtain the desired carbon nanostructures and explore more reaction possibilities.
The research, titled "Active machine learning model for the dynamic simulation and growth mechanisms of carbon on metal surface", was published in "Nature Communications" on January 6, 2024.
Paper link: https://www.nature.com/articles/s41467-023-44525-z
Substrate catalytic deposition is considered one of the most promising methods to achieve controllable growth of two- or three-dimensional covalently bonded networks of carbon atoms. While growth mechanisms on ordinary surfaces have been extensively studied, knowledge of the dynamic and atomic-scale factors that control graphene mass on high-index or composite surfaces is limited. This research gap has greatly hindered the development of theory-guided design approaches for novel catalytic metal substrates in the growth of carbon nanostructures.
Experimentally finding metal or alloy catalysts presents considerable challenges due to the wide range of potential substrates and the sensitivity of the carbon nanomaterial growth process to various experimental parameters.
Therefore, there is enough room for theoretical simulations and many atomic details are easily obtained. Examples include DFT, Kinetic Monte Carlo (KMC), and Ab initio Molecular Dynamics (AIMD). However, these methods each have their limitations. Therefore, there is still an urgent need for a robust design model that can accurately describe the carbon growth mechanism on metal surfaces.
Machine learning potentials (MLP) based on artificial neural networks or kernel methods are considered to be effective methods to solve the limited accuracy and transferability of classical force fields and maintain DFT-level accuracy. Despite significant achievements in data-driven MD simulations, constructing accurate MLPs remains a difficult task. One solution to this problem is dynamic learning techniques.
To improve the efficiency and effectiveness of dynamic training of deposition processes, a well-defined selection protocol is required. On the other hand, the dynamics of carbon growth on metallic substrates can be controlled by important rare events. Therefore, how to improve the training efficiency of MLP by combining boosted sampling methods with classical dynamics requires further research.
Data-driven automatic learning framework to generate MLP with minimum manpowerThis study proposes a data-driven automatic learning framework to generate MLP with minimum manpower , which is suitable for carbon growth on metal or alloy surfaces.
To achieve this task, the researchers used (1) Gaussian approximation potential (GAP) processing learning model; (2) an enhanced sampling method called timestamp force bias Monte Carlo (time-stamped force-biased Monte Carlo, tfMC) method to accelerate the relaxation process after carbon deposition, thereby including important rare events in the training database; (3) Smooth overlap based on atomic positions (SOAP) Efficient strategies for descriptor selection of representative training data; (4) well-established carbon training sets; (5) automated screening, fitting, and validation procedures.
Figure 1: Carbon growth on metal generated by dynamic active learning machine learning potential (CGM) during a hybrid MD/tfMC simulation -MLP) schematic diagram. (Source: Paper)
By exploiting the high accuracy of the Carbon Growth Machine Learning Potential (CGM-MLP) and incorporating rare atomic events in the MD/tfMC method, we successfully replicated graphene with metal surfaces The basic subprocesses related to nucleation and carbon growth are shown in the figure below.
Figure 2: CGM-MLP driven simulation of graphene growth on Cu(111) with different carbon incident kinetic energies (Ek). (Source: Paper)
The resulting potential was then applied to study the deposition growth of carbon atoms on the Cu (111) surface. This method can correctly capture the key processes of carbon growth on Cu(111), such as the formation and migration of subsurface carbon monomers and surface dimers, the emergence of one-dimensional carbon nanocrystals, graphene nucleation involving Cu atoms and Edge passivation of carbon chains, and precipitation growth process.
Figure 3: Carbon structure analysis and observation of carbon ring breakage by high-energy bombardment. (Source: Paper)
#Figure 4: Carbon diffusion and graphite obtained using CGM-MLP on metal and DFT-based crawling image nudging elastic band (CI-NEB) calculations The minimum energy path for ene nucleation. (Source: Paper)
Researchers investigated the initial nucleation of different metal surfaces, especially carbon deposition on Cu(111), Cr(110), Ti(001) and O-contaminated Cu(111) The simulations show consistency with experimental observations and DFT calculations.
Figure 5: Representative metal surfaces for carbon nanostructure growth. (Source: Paper)
Research Significance
In summary, this research represents a pioneering advance in the integration of MLP and MD/tfMC for designing metal or alloy substrates provides a transferable and efficient strategy to obtain desired carbon nanostructures.
CGM-MLP effectively combines the accuracy of first principles methods with the efficiency of classical force fields. The tfMC method overcomes the time scale limitations of traditional AIMD or classical MD methods. Furthermore, the automated training framework of CGM-MLP incorporates specialized query strategies for building dynamic training sets in deposition simulations, emphasizing the importance of considering the local environment around deposited atoms.
These developments make it possible to directly study the mechanism of carbon growth on complex metal surfaces. The machine learning-driven deposition model proposed in this study may provide opportunities to study the growth of a variety of carbon nanostructures (e.g., graphene, carbon nanotubes, graphite, or diamond-like carbon films) on multi-element metal or alloy substrates.
The above is the detailed content of Shanghai Jiao Tong University team develops data-driven active learning framework to accelerate research progress in carbon nanomaterials. For more information, please follow other related articles on the PHP Chinese website!

Hot AI Tools

Undresser.AI Undress
AI-powered app for creating realistic nude photos

AI Clothes Remover
Online AI tool for removing clothes from photos.

Undress AI Tool
Undress images for free

Clothoff.io
AI clothes remover

Video Face Swap
Swap faces in any video effortlessly with our completely free AI face swap tool!

Hot Article

Hot Tools

Notepad++7.3.1
Easy-to-use and free code editor

SublimeText3 Chinese version
Chinese version, very easy to use

Zend Studio 13.0.1
Powerful PHP integrated development environment

Dreamweaver CS6
Visual web development tools

SublimeText3 Mac version
God-level code editing software (SublimeText3)

Hot Topics
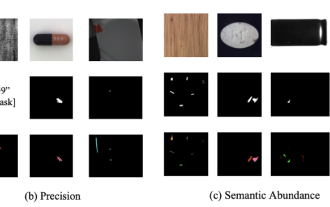
In modern manufacturing, accurate defect detection is not only the key to ensuring product quality, but also the core of improving production efficiency. However, existing defect detection datasets often lack the accuracy and semantic richness required for practical applications, resulting in models unable to identify specific defect categories or locations. In order to solve this problem, a top research team composed of Hong Kong University of Science and Technology Guangzhou and Simou Technology innovatively developed the "DefectSpectrum" data set, which provides detailed and semantically rich large-scale annotation of industrial defects. As shown in Table 1, compared with other industrial data sets, the "DefectSpectrum" data set provides the most defect annotations (5438 defect samples) and the most detailed defect classification (125 defect categories
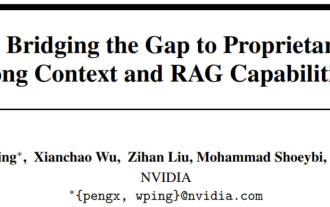
The open LLM community is an era when a hundred flowers bloom and compete. You can see Llama-3-70B-Instruct, QWen2-72B-Instruct, Nemotron-4-340B-Instruct, Mixtral-8x22BInstruct-v0.1 and many other excellent performers. Model. However, compared with proprietary large models represented by GPT-4-Turbo, open models still have significant gaps in many fields. In addition to general models, some open models that specialize in key areas have been developed, such as DeepSeek-Coder-V2 for programming and mathematics, and InternVL for visual-language tasks.
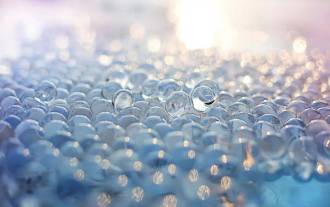
Editor |KX To this day, the structural detail and precision determined by crystallography, from simple metals to large membrane proteins, are unmatched by any other method. However, the biggest challenge, the so-called phase problem, remains retrieving phase information from experimentally determined amplitudes. Researchers at the University of Copenhagen in Denmark have developed a deep learning method called PhAI to solve crystal phase problems. A deep learning neural network trained using millions of artificial crystal structures and their corresponding synthetic diffraction data can generate accurate electron density maps. The study shows that this deep learning-based ab initio structural solution method can solve the phase problem at a resolution of only 2 Angstroms, which is equivalent to only 10% to 20% of the data available at atomic resolution, while traditional ab initio Calculation
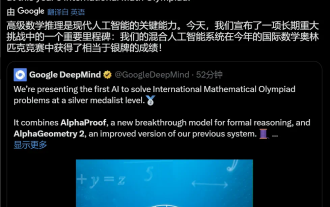
For AI, Mathematical Olympiad is no longer a problem. On Thursday, Google DeepMind's artificial intelligence completed a feat: using AI to solve the real question of this year's International Mathematical Olympiad IMO, and it was just one step away from winning the gold medal. The IMO competition that just ended last week had six questions involving algebra, combinatorics, geometry and number theory. The hybrid AI system proposed by Google got four questions right and scored 28 points, reaching the silver medal level. Earlier this month, UCLA tenured professor Terence Tao had just promoted the AI Mathematical Olympiad (AIMO Progress Award) with a million-dollar prize. Unexpectedly, the level of AI problem solving had improved to this level before July. Do the questions simultaneously on IMO. The most difficult thing to do correctly is IMO, which has the longest history, the largest scale, and the most negative
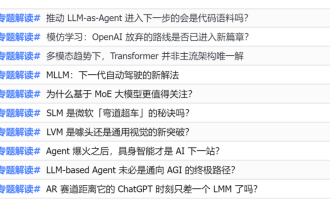
In 2023, almost every field of AI is evolving at an unprecedented speed. At the same time, AI is constantly pushing the technological boundaries of key tracks such as embodied intelligence and autonomous driving. Under the multi-modal trend, will the situation of Transformer as the mainstream architecture of AI large models be shaken? Why has exploring large models based on MoE (Mixed of Experts) architecture become a new trend in the industry? Can Large Vision Models (LVM) become a new breakthrough in general vision? ...From the 2023 PRO member newsletter of this site released in the past six months, we have selected 10 special interpretations that provide in-depth analysis of technological trends and industrial changes in the above fields to help you achieve your goals in the new year. be prepared. This interpretation comes from Week50 2023
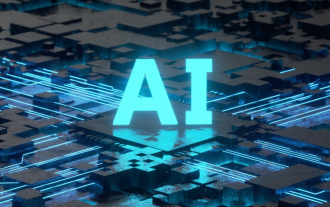
Editor |ScienceAI Question Answering (QA) data set plays a vital role in promoting natural language processing (NLP) research. High-quality QA data sets can not only be used to fine-tune models, but also effectively evaluate the capabilities of large language models (LLM), especially the ability to understand and reason about scientific knowledge. Although there are currently many scientific QA data sets covering medicine, chemistry, biology and other fields, these data sets still have some shortcomings. First, the data form is relatively simple, most of which are multiple-choice questions. They are easy to evaluate, but limit the model's answer selection range and cannot fully test the model's ability to answer scientific questions. In contrast, open-ended Q&A
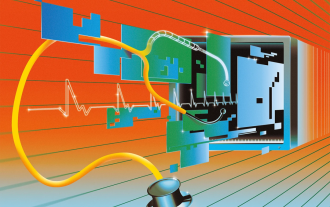
Editor | ScienceAI Based on limited clinical data, hundreds of medical algorithms have been approved. Scientists are debating who should test the tools and how best to do so. Devin Singh witnessed a pediatric patient in the emergency room suffer cardiac arrest while waiting for treatment for a long time, which prompted him to explore the application of AI to shorten wait times. Using triage data from SickKids emergency rooms, Singh and colleagues built a series of AI models that provide potential diagnoses and recommend tests. One study showed that these models can speed up doctor visits by 22.3%, speeding up the processing of results by nearly 3 hours per patient requiring a medical test. However, the success of artificial intelligence algorithms in research only verifies this
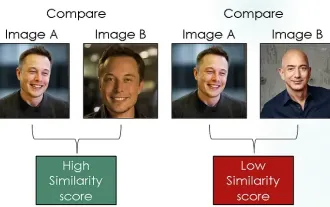
Introduction In the field of computer vision, accurately measuring image similarity is a critical task with a wide range of practical applications. From image search engines to facial recognition systems and content-based recommendation systems, the ability to effectively compare and find similar images is important. The Siamese network combined with contrastive loss provides a powerful framework for learning image similarity in a data-driven manner. In this blog post, we will dive into the details of Siamese networks, explore the concept of contrastive loss, and explore how these two components work together to create an effective image similarity model. First, the Siamese network consists of two identical subnetworks that share the same weights and parameters. Each sub-network encodes the input image into a feature vector, which
