


Small but mighty models are on the rise: TinyLlama and LiteLlama become popular choices
Currently, researchers are beginning to focus on compact and high-performance small models, although everyone is studying large models with parameter sizes reaching tens of billions or even hundreds of billions.
Small models are widely used in edge devices, such as smartphones, IoT devices, and embedded systems. These devices often have limited computing power and storage space and cannot efficiently run large language models. Therefore, studying small models becomes particularly important.
The two studies we are going to introduce next may meet your needs for small models.
TinyLlama-1.1B
Researchers at the Singapore University of Technology and Design (SUTD) recently released TinyLlama, a 1.1 billion-parameter The language model is pre-trained on approximately 3 trillion tokens.
- Paper address: https://arxiv.org/pdf/2401.02385.pdf
- Project address: https://github.com/jzhang38/TinyLlama/blob/main/README_zh-CN.md
TinyLlama is based on the Llama 2 architecture and tokenizer, which allows it to be easily integrated with many open source projects using Llama. In addition, TinyLlama has only 1.1 billion parameters and is small in size, making it ideal for applications that require limited computation and memory footprint.
The study shows that only 16 A100-40G GPUs can complete the training of TinyLlama in 90 days.
The project has continued to receive attention since its launch, and the current number of stars has reached 4.7K.
TinyLlama model architecture details are as follows:
Training details are as follows:
The researcher stated that this study aims to mine the use of larger data sets to train smaller model potential. They focused on exploring the behavior of smaller models when trained with a much larger number of tokens than recommended by the scaling law.
Specifically, the study used approximately 3 trillion tokens to train a Transformer (decoder only) model with 1.1B parameters. To our knowledge, this is the first attempt to use such a large amount of data to train a model with 1B parameters.
Despite its relatively small size, TinyLlama performs quite well on a range of downstream tasks, significantly outperforming existing open source language models of similar size. Specifically, TinyLlama outperforms OPT-1.3B and Pythia1.4B on various downstream tasks.
In addition, TinyLlama also uses various optimization methods, such as flash attention 2, FSDP (Fully Sharded Data Parallel), xFormers, etc.
With the support of these technologies, TinyLlama training throughput reaches 24,000 tokens per second per A100-40G GPU. For example, the TinyLlama-1.1B model requires only 3,456 A100 GPU hours for 300B tokens, compared to 4,830 hours for Pythia and 7,920 hours for MPT. This shows the effectiveness of this study's optimization and the potential to save significant time and resources in large-scale model training.
TinyLlama achieves a training speed of 24k tokens/second/A100. This speed is like the user can train a chinchilla with 1.1 billion parameters and 22 billion tokens on 8 A100s in 32 hours. -optimal model. At the same time, these optimizations also greatly reduce the memory usage. Users can stuff a 1.1 billion parameter model into a 40GB GPU while maintaining a per-gpu batch size of 16k tokens. Just change the batch size a little smaller, and you can train TinyLlama on RTX 3090/4090.
In the experiments, this research focuses on languages with pure decoder architectures model, containing approximately 1 billion parameters. Specifically, the study compared TinyLlama to OPT-1.3B, Pythia-1.0B, and Pythia-1.4B.
The performance of TinyLlama on common sense reasoning tasks is shown below. It can be seen that TinyLlama outperforms the baseline on many tasks and achieves the highest average score.
In addition, the researchers tracked the accuracy of TinyLlama on the common sense reasoning benchmark during pre-training, as shown in Figure 2, the performance of TinyLlama Improves with increasing computing resources, exceeding the accuracy of Pythia-1.4B in most benchmarks.
Table 3 shows that TinyLlama exhibits better problem-solving capabilities compared to existing models.
Netizens with fast hands have already started to get started: the running effect is surprisingly good, running on GTX3060, it can run at a speed of 136 tok/second .
"It's really fast!"
##Small Model LiteLlama
Due to the release of TinyLlama, SLM (Small Language Model) began to attract widespread attention. Xiaotian Han of Texas Tech and A&M University released SLM-LiteLlama. It has 460M parameters and is trained with 1T tokens. This is an open source fork of Meta AI’s LLaMa 2, but with a significantly smaller model size.
Project address: https://huggingface.co/ahxt/LiteLlama-460M-1T
LiteLlama-460M-1T is trained on the RedPajama dataset and uses GPT2Tokenizer to tokenize text. The author evaluated the model on the MMLU task, and the results are shown in the figure below. Even with a significantly reduced number of parameters, LiteLlama-460M-1T can still achieve results that are comparable to or better than other models.
The following is the performance of the model. For more details, please refer to:
https://www.php.cn/link/05ec1d748d9e3bbc975a057f7cd02fb6
The above is the detailed content of Small but mighty models are on the rise: TinyLlama and LiteLlama become popular choices. For more information, please follow other related articles on the PHP Chinese website!

Hot AI Tools

Undresser.AI Undress
AI-powered app for creating realistic nude photos

AI Clothes Remover
Online AI tool for removing clothes from photos.

Undress AI Tool
Undress images for free

Clothoff.io
AI clothes remover

Video Face Swap
Swap faces in any video effortlessly with our completely free AI face swap tool!

Hot Article

Hot Tools

Notepad++7.3.1
Easy-to-use and free code editor

SublimeText3 Chinese version
Chinese version, very easy to use

Zend Studio 13.0.1
Powerful PHP integrated development environment

Dreamweaver CS6
Visual web development tools

SublimeText3 Mac version
God-level code editing software (SublimeText3)

Hot Topics
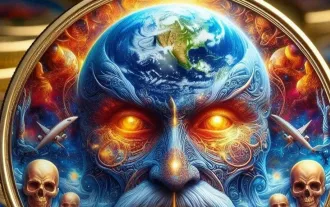
WorldCoin (WLD) stands out in the cryptocurrency market with its unique biometric verification and privacy protection mechanisms, attracting the attention of many investors. WLD has performed outstandingly among altcoins with its innovative technologies, especially in combination with OpenAI artificial intelligence technology. But how will the digital assets behave in the next few years? Let's predict the future price of WLD together. The 2025 WLD price forecast is expected to achieve significant growth in WLD in 2025. Market analysis shows that the average WLD price may reach $1.31, with a maximum of $1.36. However, in a bear market, the price may fall to around $0.55. This growth expectation is mainly due to WorldCoin2.
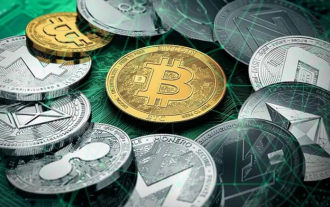
Factors of rising virtual currency prices include: 1. Increased market demand, 2. Decreased supply, 3. Stimulated positive news, 4. Optimistic market sentiment, 5. Macroeconomic environment; Decline factors include: 1. Decreased market demand, 2. Increased supply, 3. Strike of negative news, 4. Pessimistic market sentiment, 5. Macroeconomic environment.
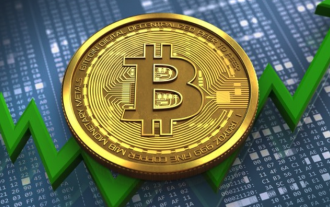
Exchanges that support cross-chain transactions: 1. Binance, 2. Uniswap, 3. SushiSwap, 4. Curve Finance, 5. Thorchain, 6. 1inch Exchange, 7. DLN Trade, these platforms support multi-chain asset transactions through various technologies.

Aavenomics is a proposal to modify the AAVE protocol token and introduce token repos, which has implemented a quorum for AAVEDAO. Marc Zeller, founder of the AAVE Project Chain (ACI), announced this on X, noting that it marks a new era for the agreement. Marc Zeller, founder of the AAVE Chain Initiative (ACI), announced on X that the Aavenomics proposal includes modifying the AAVE protocol token and introducing token repos, has achieved a quorum for AAVEDAO. According to Zeller, this marks a new era for the agreement. AaveDao members voted overwhelmingly to support the proposal, which was 100 per week on Wednesday
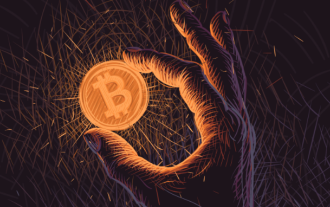
The platforms that have outstanding performance in leveraged trading, security and user experience in 2025 are: 1. OKX, suitable for high-frequency traders, providing up to 100 times leverage; 2. Binance, suitable for multi-currency traders around the world, providing 125 times high leverage; 3. Gate.io, suitable for professional derivatives players, providing 100 times leverage; 4. Bitget, suitable for novices and social traders, providing up to 100 times leverage; 5. Kraken, suitable for steady investors, providing 5 times leverage; 6. Bybit, suitable for altcoin explorers, providing 20 times leverage; 7. KuCoin, suitable for low-cost traders, providing 10 times leverage; 8. Bitfinex, suitable for senior play
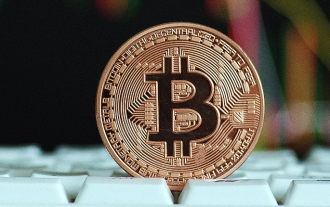
Suggestions for choosing a cryptocurrency exchange: 1. For liquidity requirements, priority is Binance, Gate.io or OKX, because of its order depth and strong volatility resistance. 2. Compliance and security, Coinbase, Kraken and Gemini have strict regulatory endorsement. 3. Innovative functions, KuCoin's soft staking and Bybit's derivative design are suitable for advanced users.
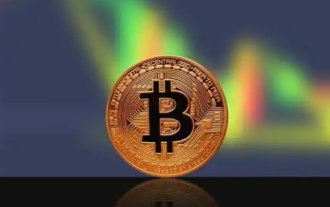
Cryptocurrency data platforms suitable for beginners include CoinMarketCap and non-small trumpet. 1. CoinMarketCap provides global real-time price, market value, and trading volume rankings for novice and basic analysis needs. 2. The non-small quotation provides a Chinese-friendly interface, suitable for Chinese users to quickly screen low-risk potential projects.
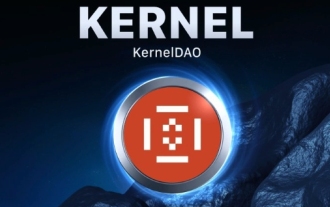
In the bustling world of cryptocurrencies, new opportunities always emerge. At present, KernelDAO (KERNEL) airdrop activity is attracting much attention and attracting the attention of many investors. So, what is the origin of this project? What benefits can BNB Holder get from it? Don't worry, the following will reveal it one by one for you.
