


Segment features can be learned by labeling a single frame of video, achieving fully supervised performance! Huake wins new SOTA for sequential behavior detection
How to find interesting clips from a video? Temporal Action Localization (TAL) is a common method.
After using video content for modeling, you can freely search within the entire video. The joint team of Huazhong University of Science and Technology and the University of Michigan has recently brought new progress to this technology-In the past, modeling in TAL was at the fragment or even instance level; Now only one frame ofin the video can achieve , and the effect is comparable to that of full supervision.
- For the "golf swing" behavior, HR-Pro effectively distinguishes between behavior and context Segments, alleviating LACP's intractable False Positive predictions;
- For the discus throwing behavior, HR-Pro detects more complete segments than LACP, which has lower activation values on non-discriminative action segments .
(The left side is the result before instance-level integrity learning, and the right side is the result after learning. The horizontal and vertical axes represent time and reliability scores respectively.)
fragment-level discriminative learningandinstance-level complete Sexual learning.
Phase 1: Segment-level discriminative learning
The research team introduces reliability-aware segment-level discriminative learning, proposes to store reliable prototypes for each category, and uses them within the video to and video-to-video methods to propagate high-confidence cues from these prototypes to other clips.
Fragment-level reliable prototype construction
In order to build reliable prototypes at the fragment level, the team created an online updated prototype memory to store reliable prototypes of various behaviors mc (where c = 1, 2, …, C) in order to be able to utilize the feature information of the entire data set.
The research team chose to initialize the prototype with point-labeled segment features:
Next, the researchers used pseudo-labeled behavioral segment features to update each Category prototypes are specifically expressed as follows:
Fragment-level reliability awareness optimization
In order to combine the feature information of fragment-level reliable prototypes Passed to other fragments, the research team designed a Reliabilty-aware Attention Block (RAB) to inject reliable information from the prototype into other fragments through cross-attention, thereby enhancing the robustness of fragment features and Increased focus on less discriminative segments.
In order to learn more discriminative fragment features, the team also constructed a reliability-aware fragment comparison loss:
Phase 2: Instance-level integrity learning
In order to fully explore the temporal structure of instance-level behaviors and optimize the proposed score ranking, the team introduced instance-level action integrity learning.
This approach aims to refine the confidence scores and bounds of proposals through instance-level feature learning, guided by reliable instance prototypes.
Instance-level reliable prototype construction
In order to utilize the instance-level prior information of point annotation during the training process, the team proposed a proposal generation method based on point annotation Used to generate proposals with different Reliabilities.
According to their reliability scores and relative point annotated timing positions, these proposals can be divided into two types:
- ##Reliable Proposals (Reliable Proposals, RP ): For each point in each category, the proposal contains this point and has the highest reliability;
- Positive Proposals (Positive Proposals, PP): All the rest Candidate Proposal.
Instance-level reliability-aware optimization
To predict the completeness score of each proposal, the research team inputs the proposal features of sensitive boundaries into the score prediction head φs:
# In short, HR-Pro can achieve great results with only a few annotations. It reduces the cost of obtaining tags and at the same time has strong generalization capabilities, providing favorable conditions for actual deployment applications.
According to this, the author predicts that HR-Pro will have broad application prospects in behavioral analysis, human-computer interaction, driving analysis and other fields.
Paper address: https://arxiv.org/abs/2308.12608
The above is the detailed content of Segment features can be learned by labeling a single frame of video, achieving fully supervised performance! Huake wins new SOTA for sequential behavior detection. For more information, please follow other related articles on the PHP Chinese website!

Hot AI Tools

Undresser.AI Undress
AI-powered app for creating realistic nude photos

AI Clothes Remover
Online AI tool for removing clothes from photos.

Undress AI Tool
Undress images for free

Clothoff.io
AI clothes remover

Video Face Swap
Swap faces in any video effortlessly with our completely free AI face swap tool!

Hot Article

Hot Tools

Notepad++7.3.1
Easy-to-use and free code editor

SublimeText3 Chinese version
Chinese version, very easy to use

Zend Studio 13.0.1
Powerful PHP integrated development environment

Dreamweaver CS6
Visual web development tools

SublimeText3 Mac version
God-level code editing software (SublimeText3)

Hot Topics
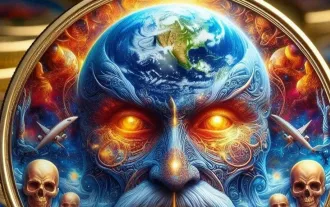
WorldCoin (WLD) stands out in the cryptocurrency market with its unique biometric verification and privacy protection mechanisms, attracting the attention of many investors. WLD has performed outstandingly among altcoins with its innovative technologies, especially in combination with OpenAI artificial intelligence technology. But how will the digital assets behave in the next few years? Let's predict the future price of WLD together. The 2025 WLD price forecast is expected to achieve significant growth in WLD in 2025. Market analysis shows that the average WLD price may reach $1.31, with a maximum of $1.36. However, in a bear market, the price may fall to around $0.55. This growth expectation is mainly due to WorldCoin2.
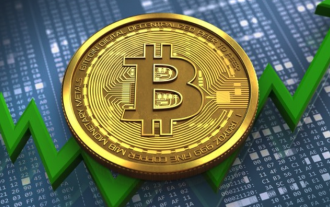
Exchanges that support cross-chain transactions: 1. Binance, 2. Uniswap, 3. SushiSwap, 4. Curve Finance, 5. Thorchain, 6. 1inch Exchange, 7. DLN Trade, these platforms support multi-chain asset transactions through various technologies.
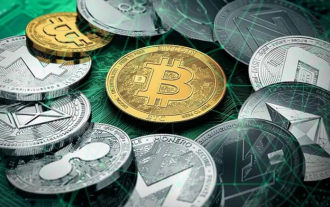
Factors of rising virtual currency prices include: 1. Increased market demand, 2. Decreased supply, 3. Stimulated positive news, 4. Optimistic market sentiment, 5. Macroeconomic environment; Decline factors include: 1. Decreased market demand, 2. Increased supply, 3. Strike of negative news, 4. Pessimistic market sentiment, 5. Macroeconomic environment.
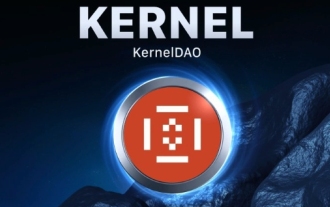
In the bustling world of cryptocurrencies, new opportunities always emerge. At present, KernelDAO (KERNEL) airdrop activity is attracting much attention and attracting the attention of many investors. So, what is the origin of this project? What benefits can BNB Holder get from it? Don't worry, the following will reveal it one by one for you.
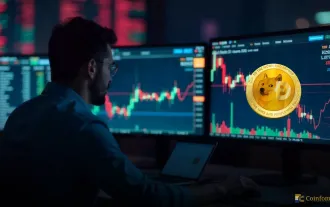
The plunge in the cryptocurrency market has caused panic among investors, and Dogecoin (Doge) has become one of the hardest hit areas. Its price fell sharply, and the total value lock-in of decentralized finance (DeFi) (TVL) also saw a significant decline. The selling wave of "Black Monday" swept the cryptocurrency market, and Dogecoin was the first to be hit. Its DeFiTVL fell to 2023 levels, and the currency price fell 23.78% in the past month. Dogecoin's DeFiTVL fell to a low of $2.72 million, mainly due to a 26.37% decline in the SOSO value index. Other major DeFi platforms, such as the boring Dao and Thorchain, TVL also dropped by 24.04% and 20, respectively.

Aavenomics is a proposal to modify the AAVE protocol token and introduce token repos, which has implemented a quorum for AAVEDAO. Marc Zeller, founder of the AAVE Project Chain (ACI), announced this on X, noting that it marks a new era for the agreement. Marc Zeller, founder of the AAVE Chain Initiative (ACI), announced on X that the Aavenomics proposal includes modifying the AAVE protocol token and introducing token repos, has achieved a quorum for AAVEDAO. According to Zeller, this marks a new era for the agreement. AaveDao members voted overwhelmingly to support the proposal, which was 100 per week on Wednesday
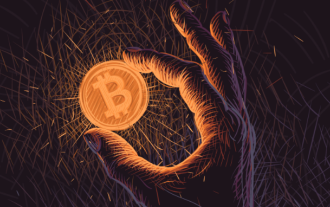
The platforms that have outstanding performance in leveraged trading, security and user experience in 2025 are: 1. OKX, suitable for high-frequency traders, providing up to 100 times leverage; 2. Binance, suitable for multi-currency traders around the world, providing 125 times high leverage; 3. Gate.io, suitable for professional derivatives players, providing 100 times leverage; 4. Bitget, suitable for novices and social traders, providing up to 100 times leverage; 5. Kraken, suitable for steady investors, providing 5 times leverage; 6. Bybit, suitable for altcoin explorers, providing 20 times leverage; 7. KuCoin, suitable for low-cost traders, providing 10 times leverage; 8. Bitfinex, suitable for senior play
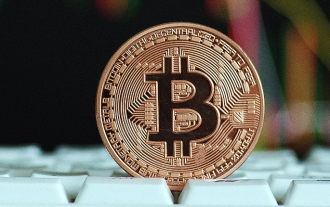
Suggestions for choosing a cryptocurrency exchange: 1. For liquidity requirements, priority is Binance, Gate.io or OKX, because of its order depth and strong volatility resistance. 2. Compliance and security, Coinbase, Kraken and Gemini have strict regulatory endorsement. 3. Innovative functions, KuCoin's soft staking and Bybit's derivative design are suitable for advanced users.
