


Research on methods to solve write performance problems encountered in MongoDB technology development
Research on methods to solve write performance problems encountered in MongoDB technology development
[Introduction]
With the rapid development of the Internet and mobile applications, data Volume increases exponentially. As a high-performance, non-relational database, MongoDB is widely used in various application scenarios. However, during the actual development process, we may encounter the problem of reduced writing performance, which directly affects the stability of the system and user experience. This article will analyze the write performance problems encountered in MongoDB technology development, analyze their causes, and propose some solutions, along with specific code examples.
[Problem Analysis]
In the technical development process of MongoDB, write performance problems may originate from many aspects, including hardware resource limitations, unreasonable index design, and low batch insertion efficiency. Below we will analyze these aspects.
- Hardware resource limitations
MongoDB has high requirements for hard disk and memory. If hardware resources are insufficient, writing performance will decrease. For example, slow disk speed, insufficient memory, high CPU utilization, etc. may cause write operations to slow down. - Unreasonable index design
MongoDB is a database based on document structure, and indexes play a key role in improving query performance. However, if the index design is not reasonable, the efficiency of the write operation will be reduced. For example, too many indexes will increase additional overhead during writing, making write operations slower. At the same time, unreasonable index design will also affect the performance of update and delete operations. - Low efficiency of batch insertion
In actual development, we often need to batch insert a large amount of data into MongoDB. However, there is a big difference in MongoDB's write performance between single insertion and batch insertion. Without a proper approach to bulk inserts, it can lead to write inefficiencies.
[Solution]
When solving the write performance problems encountered in MongoDB technology development, we can take the following methods:
- Hardware resource optimization
First, we need to ensure that MongoDB is running with sufficient hardware resources. You can consider upgrading your hard drive and using high-speed storage media such as SSD to increase disk read and write speeds. At the same time, memory resources are allocated reasonably to ensure that MongoDB can make full use of memory for data reading and writing operations. In addition, you can consider using a distributed architecture to store data dispersedly on multiple machines to improve write performance. - Reasonably design the index
For the problem of unreasonable index design, we can optimize it through the following methods: - Delete unnecessary indexes: evaluate the usage of existing indexes and delete them in time Unnecessary indexes reduce the overhead of write operations.
- Design a suitable composite index: According to the actual query requirements, design a suitable composite index to improve the efficiency of write operations.
- Choose the appropriate index type: MongoDB supports multiple index types, such as single-key index, multi-key index, geospatial index, etc. Choosing the appropriate index type can better meet the needs of actual application scenarios.
- Using Bulk Insertion
In order to improve the efficiency of batch insertion, we can use the Bulk Write API provided by MongoDB for batch insertion operations. This API can combine multiple insert operations into one request and send it to the server, thereby reducing network overhead and improving write performance. The following is a code example using the Bulk Write API for batch insertion:
from pymongo import MongoClient from pymongo import InsertOne def batch_insert_data(data_list): client = MongoClient("mongodb://localhost:27017") db = client["test_db"] collection = db["test_collection"] bulk_operations = [InsertOne(data) for data in data_list] collection.bulk_write(bulk_operations) if __name__ == "__main__": data_list = [{"name": "Tom", "age": 18}, {"name": "Jack", "age": 20}] batch_insert_data(data_list)
[Summary]
In view of the write performance problems encountered in the development of MongoDB technology, this article starts from the perspective of hardware resource optimization, indexing Solutions are proposed in three aspects: design optimization and batch insertion optimization, and corresponding code examples are provided. In actual development, we can choose appropriate methods to optimize performance based on specific application scenarios and data volume, thereby improving system stability and user experience.
The above is the detailed content of Research on methods to solve write performance problems encountered in MongoDB technology development. For more information, please follow other related articles on the PHP Chinese website!

Hot AI Tools

Undresser.AI Undress
AI-powered app for creating realistic nude photos

AI Clothes Remover
Online AI tool for removing clothes from photos.

Undress AI Tool
Undress images for free

Clothoff.io
AI clothes remover

Video Face Swap
Swap faces in any video effortlessly with our completely free AI face swap tool!

Hot Article

Hot Tools

Notepad++7.3.1
Easy-to-use and free code editor

SublimeText3 Chinese version
Chinese version, very easy to use

Zend Studio 13.0.1
Powerful PHP integrated development environment

Dreamweaver CS6
Visual web development tools

SublimeText3 Mac version
God-level code editing software (SublimeText3)

Hot Topics
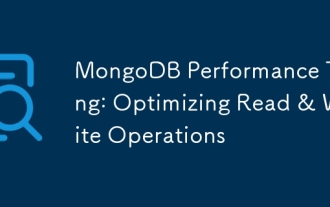
The core strategies of MongoDB performance tuning include: 1) creating and using indexes, 2) optimizing queries, and 3) adjusting hardware configuration. Through these methods, the read and write performance of the database can be significantly improved, response time, and throughput can be improved, thereby optimizing the user experience.
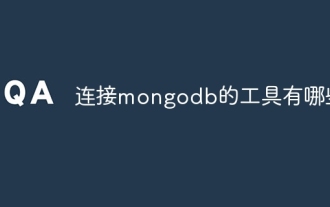
The main tools for connecting to MongoDB are: 1. MongoDB Shell, suitable for quickly viewing data and performing simple operations; 2. Programming language drivers (such as PyMongo, MongoDB Java Driver, MongoDB Node.js Driver), suitable for application development, but you need to master the usage methods; 3. GUI tools (such as Robo 3T, Compass) provide a graphical interface for beginners and quick data viewing. When selecting tools, you need to consider application scenarios and technology stacks, and pay attention to connection string configuration, permission management and performance optimization, such as using connection pools and indexes.
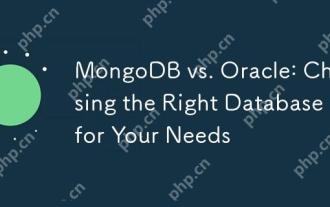
MongoDB is suitable for unstructured data and high scalability requirements, while Oracle is suitable for scenarios that require strict data consistency. 1.MongoDB flexibly stores data in different structures, suitable for social media and the Internet of Things. 2. Oracle structured data model ensures data integrity and is suitable for financial transactions. 3.MongoDB scales horizontally through shards, and Oracle scales vertically through RAC. 4.MongoDB has low maintenance costs, while Oracle has high maintenance costs but is fully supported.
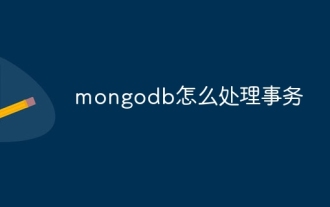
Transaction processing in MongoDB provides solutions such as multi-document transactions, snapshot isolation, and external transaction managers to achieve transaction behavior, ensure multiple operations are executed as one atomic unit, ensuring atomicity and isolation. Suitable for applications that need to ensure data integrity, prevent concurrent operational data corruption, or implement atomic updates in distributed systems. However, its transaction processing capabilities are limited and are only suitable for a single database instance. Multi-document transactions only support read and write operations. Snapshot isolation does not provide atomic guarantees. Integrating external transaction managers may also require additional development work.
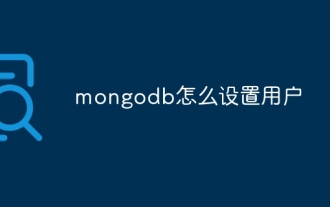
To set up a MongoDB user, follow these steps: 1. Connect to the server and create an administrator user. 2. Create a database to grant users access. 3. Use the createUser command to create a user and specify their role and database access rights. 4. Use the getUsers command to check the created user. 5. Optionally set other permissions or grant users permissions to a specific collection.
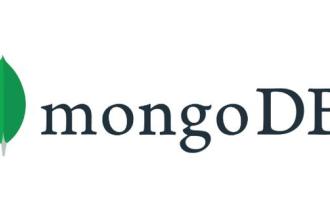
Choosing MongoDB or relational database depends on application requirements. 1. Relational databases (such as MySQL) are suitable for applications that require high data integrity and consistency and fixed data structures, such as banking systems; 2. NoSQL databases such as MongoDB are suitable for processing massive, unstructured or semi-structured data and have low requirements for data consistency, such as social media platforms. The final choice needs to weigh the pros and cons and decide based on the actual situation. There is no perfect database, only the most suitable database.
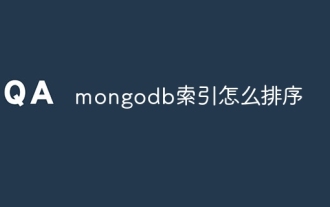
Sorting index is a type of MongoDB index that allows sorting documents in a collection by specific fields. Creating a sort index allows you to quickly sort query results without additional sorting operations. Advantages include quick sorting, override queries, and on-demand sorting. The syntax is db.collection.createIndex({ field: <sort order> }), where <sort order> is 1 (ascending order) or -1 (descending order). You can also create multi-field sorting indexes that sort multiple fields.
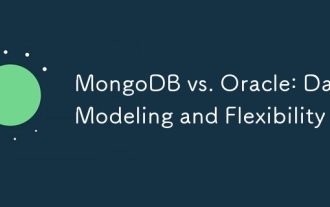
MongoDB is more suitable for processing unstructured data and rapid iteration, while Oracle is more suitable for scenarios that require strict data consistency and complex queries. 1.MongoDB's document model is flexible and suitable for handling complex data structures. 2. Oracle's relationship model is strict to ensure data consistency and complex query performance.
