


Python problems encountered in image processing and solutions
Python problems and solutions encountered in image processing
Abstract: Image processing has a wide range of applications in the fields of computer vision and graphics. Python, as a popular programming language, is also widely used in image processing. However, when we use Python in image processing, we may encounter some common problems. This article will introduce some common Python image processing problems and give solutions and corresponding code examples.
Introduction: Image processing refers to the process of editing, enhancing, analyzing and understanding images. Python provides many powerful image processing libraries, such as OpenCV, PIL and scikit-image. However, we may encounter some common problems when using these libraries.
Question 1: Reading and displaying images
When we use Python for image processing, the first problem to solve is how to read and display images. Python provides many libraries for processing images, among which OpenCV is a very popular choice. The following is a code example for using OpenCV to read and display images:
import cv2 # 读取图像 image = cv2.imread('image.jpg') # 显示图像 cv2.imshow('Image', image) cv2.waitKey(0) cv2.destroyAllWindows()
Question 2: Adjust image size
In image processing, it is often necessary to adjust the size of the image to adapt to different needs. The following is a code example that uses OpenCV to resize an image to a specified size:
import cv2 # 读取图像 image = cv2.imread('image.jpg') # 调整图像大小 resized_image = cv2.resize(image, (800, 600)) # 显示调整大小后的图像 cv2.imshow('Resized Image', resized_image) cv2.waitKey(0) cv2.destroyAllWindows()
Question 3: Image filtering
Image filtering is one of the important tasks in image processing, used to remove noise and smooth images. The following is a code example using OpenCV to filter images:
import cv2 # 读取图像 image = cv2.imread('image.jpg') # 将图像转换为灰度图 gray_image = cv2.cvtColor(image, cv2.COLOR_BGR2GRAY) # 对灰度图进行高斯滤波 blurred_image = cv2.GaussianBlur(gray_image, (5, 5), 0) # 显示滤波后的图像 cv2.imshow('Blurred Image', blurred_image) cv2.waitKey(0) cv2.destroyAllWindows()
Question 4: Image edge detection
Image edge detection is often used to extract edge information in image processing. The following is a code example of using OpenCV for image edge detection:
import cv2 # 读取图像 image = cv2.imread('image.jpg') # 将图像转换为灰度图 gray_image = cv2.cvtColor(image, cv2.COLOR_BGR2GRAY) # 对灰度图进行Canny边缘检测 edges = cv2.Canny(gray_image, 100, 200) # 显示边缘图像 cv2.imshow('Edges', edges) cv2.waitKey(0) cv2.destroyAllWindows()
Question 5: Image segmentation
Image segmentation is one of the important tasks in image processing and is used to decompose the image into several sub-regions. The following is a code example for image segmentation using scikit-image:
from skimage import segmentation import matplotlib.pyplot as plt # 读取图像 image = plt.imread('image.jpg') # 对图像进行分割 segments = segmentation.slic(image, n_segments=100) # 显示分割后的图像 plt.imshow(segments) plt.axis('off') plt.show()
Conclusion: This article introduces common Python problems in image processing and provides corresponding solutions and code examples. By learning and mastering these solutions, we can better utilize Python for image processing and achieve better results in practical applications. Image processing is a vast and complex field. I hope this article can provide some help to readers in Python programming in image processing.
The above is the detailed content of Python problems encountered in image processing and solutions. For more information, please follow other related articles on the PHP Chinese website!

Hot AI Tools

Undresser.AI Undress
AI-powered app for creating realistic nude photos

AI Clothes Remover
Online AI tool for removing clothes from photos.

Undress AI Tool
Undress images for free

Clothoff.io
AI clothes remover

Video Face Swap
Swap faces in any video effortlessly with our completely free AI face swap tool!

Hot Article

Hot Tools

Notepad++7.3.1
Easy-to-use and free code editor

SublimeText3 Chinese version
Chinese version, very easy to use

Zend Studio 13.0.1
Powerful PHP integrated development environment

Dreamweaver CS6
Visual web development tools

SublimeText3 Mac version
God-level code editing software (SublimeText3)

Hot Topics










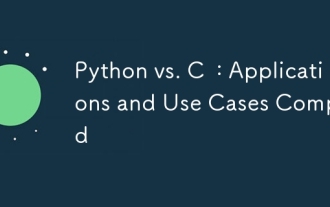
Python is suitable for data science, web development and automation tasks, while C is suitable for system programming, game development and embedded systems. Python is known for its simplicity and powerful ecosystem, while C is known for its high performance and underlying control capabilities.
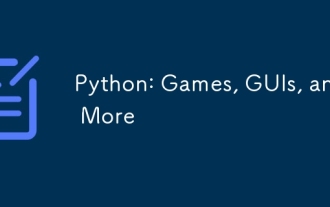
Python excels in gaming and GUI development. 1) Game development uses Pygame, providing drawing, audio and other functions, which are suitable for creating 2D games. 2) GUI development can choose Tkinter or PyQt. Tkinter is simple and easy to use, PyQt has rich functions and is suitable for professional development.
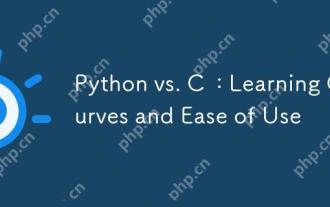
Python is easier to learn and use, while C is more powerful but complex. 1. Python syntax is concise and suitable for beginners. Dynamic typing and automatic memory management make it easy to use, but may cause runtime errors. 2.C provides low-level control and advanced features, suitable for high-performance applications, but has a high learning threshold and requires manual memory and type safety management.
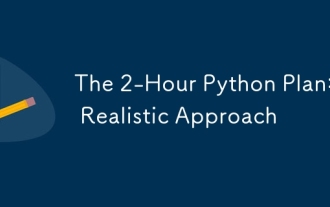
You can learn basic programming concepts and skills of Python within 2 hours. 1. Learn variables and data types, 2. Master control flow (conditional statements and loops), 3. Understand the definition and use of functions, 4. Quickly get started with Python programming through simple examples and code snippets.
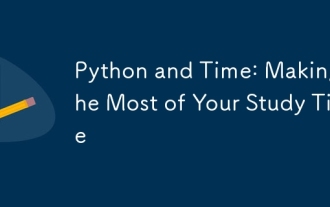
To maximize the efficiency of learning Python in a limited time, you can use Python's datetime, time, and schedule modules. 1. The datetime module is used to record and plan learning time. 2. The time module helps to set study and rest time. 3. The schedule module automatically arranges weekly learning tasks.
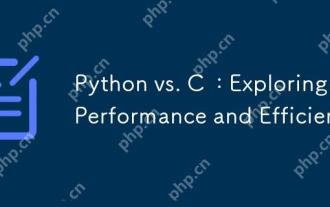
Python is better than C in development efficiency, but C is higher in execution performance. 1. Python's concise syntax and rich libraries improve development efficiency. 2.C's compilation-type characteristics and hardware control improve execution performance. When making a choice, you need to weigh the development speed and execution efficiency based on project needs.
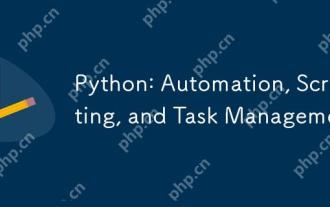
Python excels in automation, scripting, and task management. 1) Automation: File backup is realized through standard libraries such as os and shutil. 2) Script writing: Use the psutil library to monitor system resources. 3) Task management: Use the schedule library to schedule tasks. Python's ease of use and rich library support makes it the preferred tool in these areas.
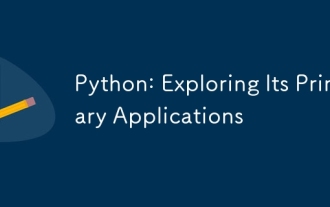
Python is widely used in the fields of web development, data science, machine learning, automation and scripting. 1) In web development, Django and Flask frameworks simplify the development process. 2) In the fields of data science and machine learning, NumPy, Pandas, Scikit-learn and TensorFlow libraries provide strong support. 3) In terms of automation and scripting, Python is suitable for tasks such as automated testing and system management.
