How many AI labeling companies will Google's 'big move” kill?
Small handmade workshops are ultimately no match for factory assembly lines.
If the current generative AI is a child that is growing vigorously, then the endless stream of data is the food that feeds its growth.
Data annotation is the process of making this “food”
However, this process is really complicated and tiring.
The "annotator" who performs annotation not only needs to repeatedly identify various objects, colors, shapes, etc. in the image, but sometimes even needs to clean and preprocess the data.
With the continuous advancement of artificial intelligence technology, the limitations of manual data annotation are becoming more and more obvious. Manual data annotation is not only time-consuming and energy-consuming, but also sometimes difficult to ensure quality
In order to solve these problems, Google recently proposed a method called AI Feedback Reinforcement Learning (RLAIF), which uses large models to replace humans for preference annotation
Research results show that RLAIF can achieve equivalent improvements to human feedback reinforcement learning (RLHF) without relying on human annotation, and the winning rate of both is 50%. In addition, the study also found that both RLAIF and RLHF are superior to the baseline strategy of supervised fine-tuning (SFT)
These results show that RLAIF does not need to rely on manual annotation and is a feasible alternative to RLHF.
If this technology is really widely promoted and popularized in the future, will many companies that rely on manual "pulling boxes" for data annotation face a desperate situation?
01 Current status of data annotation
If we want to simply summarize the current status of the domestic annotation industry, it is: The workload is large, but the efficiency is not very high, and it is a thankless state.
Labeled companies are called data factories in the AI field and are usually concentrated in areas with rich human resources such as Southeast Asia, Africa, or Henan, Shanxi, and Shandong in China.
In order to reduce costs, the bosses of labeling companies will rent a space in the county to place computer equipment. Once there is an order, they will recruit part-time staff nearby to handle it. If there is no order, they will disband and take a break
To put it simply, this type of work is a bit similar to temporary decoration workers on the roadside.
At the work station, the system will randomly give the "annotator" a set of data, which usually includes several questions and several answers.
After that, the "annotator" needs to first mark what type the question belongs to, and then score and sort the answers respectively.
Previously, when people were talking about the gap between domestic large models and advanced large models such as GPT-4, they summed up the reasons for the low quality of domestic data.
Why is the data quality not high? Part of the reason lies in the "pipeline" of data annotation
Currently, there are two types of data sources for Chinese large models, one is open source data sets; the other is Chinese Internet data crawled through crawlers.
One of the main reasons why the performance of Chinese large models is not good enough is the quality of Internet data. For example, professionals generally do not use Baidu when searching for information.
Therefore, when facing some more professional and vertical data problems, such as medical care, finance, etc., it is necessary to cooperate with a professional team.
But at this time, the problem comes again: for professional teams, not only is the return period long in terms of data, but also the first movers are likely to suffer losses.
For example, a certain annotation team spent a lot of money and time to produce a lot of data, but others may just package and buy it for a small amount of money.
Faced with this "free-rider dilemma", many large domestic models have fallen into a strange situation where although there is a lot of data, the quality is not high
In this case, how do some of the leading foreign AI companies, such as OpenAI, solve this problem?
OpenAI has not given up using cheap and intensive labor to reduce costs in data annotation
For example, it was previously revealed that it had hired a large number of Kenyan workers to label toxic information at a price of US$2/hour.
However, the important difference is how to solve the problems of data quality and annotation efficiency
Specifically, the biggest difference between OpenAI and domestic companies in this regard is how to reduce the impact of "subjectivity" and "instability" of manual annotation.
02 OpenAI’s method When rewriting the content, the language needs to be rewritten into Chinese, and the original sentence does not need to appear
In order to reduce the "subjectivity" and "instability" of such human annotators, OpenAI roughly adopts two main strategies:
1. Combination of artificial feedback and reinforcement learning;
When re-writing, the original content needs to be converted to Chinese. Here’s what it looks like after rewriting: First, let's talk about labeling. The biggest difference between OpenAI's artificial feedback and domestic ones is that it mainly sorts or scores the behavior of the intelligent system, rather than modifying or labeling its output.
The behavior of an intelligent system refers to a series of actions or decisions taken by the intelligent system according to its own goals and strategies in a complex environmentSuch as playing games, controlling robots, talking to people, etc.
Generally speaking, the behavior of intelligent systems is often difficult to judge in terms of "right" or "wrong", but rather needs to be evaluated in terms of preference or satisfaction
This kind of evaluation system based on "preference" or "satisfaction" does not require modification or marking of specific content, thus reducing the impact of human subjectivity, knowledge level and other factors on the quality and accuracy of data annotation
2. Diversified and large-scale data source channels;
Domestic data annotation sources mainly come from third-party annotation companies or self-built teams of technology companies. These teams are mostly composed of undergraduates and lack sufficient professionalism and experience, making it difficult to provide high-quality and efficient feedback.
OpenAI cooperates with a number of data companies and institutions, such as Scale AI, Appen, Lionbridge AI, etc., not only using open source data sets and Internet crawlers to obtain data, but also committed to obtaining more diverse and high-quality data
The annotation methods of these data companies and institutions are more "automated" and "intelligent" than their domestic counterparts
At the same time, Snorkel can also use a variety of signals such as rules, models, and knowledge bases to add labels to data without manually labeling each data point directly. This can greatly reduce the cost and time of manual annotation.
In this way, the free-riding dilemma of "first movers will suffer" has also been eliminated by strong technical and industry barriers.
Comparison between standardization and small workshops
It can be seen thatAI automatic labeling technology will really eliminate only those labeling companies that are still using purely manual labeling.
Although data annotation sounds like a "labor-intensive" industry, once you delve into the details, you will find that pursuing high-quality data is not an easy task.Represented by Scale AI, a unicorn with overseas data annotation, Scale AI not only uses cheap human resources from Africa and other places, but also recruits dozens of PhDs to deal with professional data in various industries.
The greatest value that Scale AI provides to large model companies such as OpenAI is the quality of data annotation
To ensure data quality to the greatest extent, in addition to the use of AI-assisted annotation mentioned above, Another major innovation of Scale AI is a unified data platform.
These platforms include Scale Audit, Scale Analytics, ScaleData Quality, etc. Through these platforms, customers can monitor and analyze various indicators in the annotation process, verify and optimize the annotation data, and evaluate the accuracy, consistency and completeness of the annotation.
It can be said that such standardized and unified tools and processes have become the key factors to distinguish "assembly line factories" and "handmade workshops" in labeling companies.
In this regard, most domestic annotation companies are still using "manual review" to review the quality of data annotation. Only a few giants such as Baidu have introduced more advanced management and evaluation tools, such as EasyData Intelligence Data service platform.
If there are no specialized tools to monitor and analyze annotation results and indicators, then in terms of key data auditing, data quality control can only rely on manual experience, and this method can still only reach a workshop-style level
Therefore, more and more Chinese companies, such as Baidu, My Neighbor Totoro Data, etc., are beginning to use machine learning and artificial intelligence technologies to improve the efficiency and quality of data annotation and realize a model of human-machine collaboration
From this perspective, the emergence of artificial intelligence labeling does not mean the end of domestic labeling companies, but the end of the traditional inefficient, cheap, and labor-intensive labeling methods that lack technical content
The above is the detailed content of How many AI labeling companies will Google's 'big move” kill?. For more information, please follow other related articles on the PHP Chinese website!

Hot AI Tools

Undresser.AI Undress
AI-powered app for creating realistic nude photos

AI Clothes Remover
Online AI tool for removing clothes from photos.

Undress AI Tool
Undress images for free

Clothoff.io
AI clothes remover

Video Face Swap
Swap faces in any video effortlessly with our completely free AI face swap tool!

Hot Article

Hot Tools

Notepad++7.3.1
Easy-to-use and free code editor

SublimeText3 Chinese version
Chinese version, very easy to use

Zend Studio 13.0.1
Powerful PHP integrated development environment

Dreamweaver CS6
Visual web development tools

SublimeText3 Mac version
God-level code editing software (SublimeText3)

Hot Topics
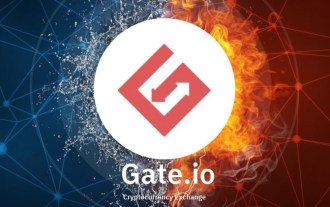
A detailed introduction to the login operation of the Sesame Open Exchange web version, including login steps and password recovery process. It also provides solutions to common problems such as login failure, unable to open the page, and unable to receive verification codes to help you log in to the platform smoothly.
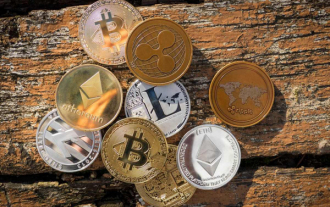
This article introduces the registration process of the Sesame Open Exchange (Gate.io) web version and the Gate trading app in detail. Whether it is web registration or app registration, you need to visit the official website or app store to download the genuine app, then fill in the user name, password, email, mobile phone number and other information, and complete email or mobile phone verification.
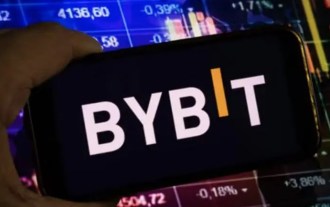
Why can’t the Bybit exchange link be directly downloaded and installed? Bybit is a cryptocurrency exchange that provides trading services to users. The exchange's mobile apps cannot be downloaded directly through AppStore or GooglePlay for the following reasons: 1. App Store policy restricts Apple and Google from having strict requirements on the types of applications allowed in the app store. Cryptocurrency exchange applications often do not meet these requirements because they involve financial services and require specific regulations and security standards. 2. Laws and regulations Compliance In many countries, activities related to cryptocurrency transactions are regulated or restricted. To comply with these regulations, Bybit Application can only be used through official websites or other authorized channels
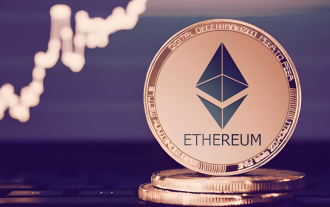
This article recommends the top ten cryptocurrency trading platforms worth paying attention to, including Binance, OKX, Gate.io, BitFlyer, KuCoin, Bybit, Coinbase Pro, Kraken, BYDFi and XBIT decentralized exchanges. These platforms have their own advantages in terms of transaction currency quantity, transaction type, security, compliance, and special features. For example, Binance is known for its largest transaction volume and abundant functions in the world, while BitFlyer attracts Asian users with its Japanese Financial Hall license and high security. Choosing a suitable platform requires comprehensive consideration based on your own trading experience, risk tolerance and investment preferences. Hope this article helps you find the best suit for yourself
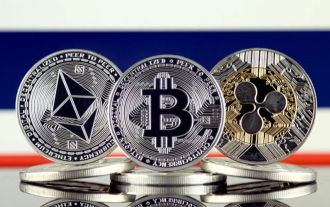
It is crucial to choose a formal channel to download the app and ensure the safety of your account.
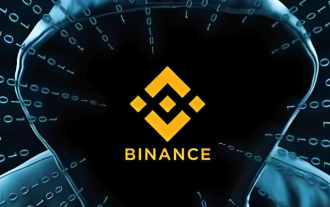
To access the latest version of Binance website login portal, just follow these simple steps. Go to the official website and click the "Login" button in the upper right corner. Select your existing login method. If you are a new user, please "Register". Enter your registered mobile number or email and password and complete authentication (such as mobile verification code or Google Authenticator). After successful verification, you can access the latest version of Binance official website login portal.
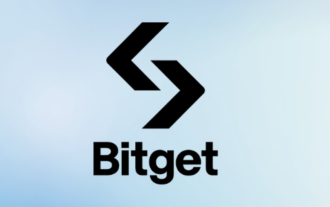
This guide provides detailed download and installation steps for the official Bitget Exchange app, suitable for Android and iOS systems. The guide integrates information from multiple authoritative sources, including the official website, the App Store, and Google Play, and emphasizes considerations during download and account management. Users can download the app from official channels, including app store, official website APK download and official website jump, and complete registration, identity verification and security settings. In addition, the guide covers frequently asked questions and considerations, such as
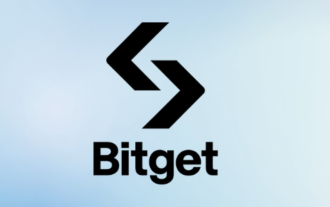
This guide provides detailed download and installation steps for the official Bitget Exchange app, suitable for Android and iOS systems. The guide integrates information from multiple authoritative sources, including the official website, the App Store, and Google Play, and emphasizes considerations during download and account management. Users can download the app from official channels, including app store, official website APK download and official website jump, and complete registration, identity verification and security settings. In addition, the guide covers frequently asked questions and considerations, such as
