How to implement real-time anomaly detection of data in MongoDB
How to implement real-time anomaly detection function of data in MongoDB
In recent years, the rapid development of big data has brought about a surge in data scale. In this massive amount of data, the detection of abnormal data has become increasingly important. MongoDB is one of the most popular non-relational databases and has the characteristics of high scalability and flexibility. This article will introduce how to implement real-time anomaly detection of data in MongoDB and provide specific code examples.
1. Data collection and storage
First, we need to establish a MongoDB database and create a data collection to store the data to be detected. You can use the following command to create a MongoDB collection:
use testdb db.createCollection("data")
2. Data preprocessing
Before performing anomaly detection, we need to preprocess the data, including data cleaning, data conversion, etc. In the example below, we sort all the documents in the data collection in ascending order by the timestamp field.
db.data.aggregate([ { $sort: { timestamp: 1 } } ])
3. Anomaly detection algorithm
Next, we will introduce a commonly used anomaly detection algorithm-Isolation Forest. The isolation forest algorithm is a tree-based anomaly detection algorithm. Its main idea is to isolate abnormal data in relatively small areas in the data set.
In order to use the isolation forest algorithm, we need to first install a third-party library for anomaly detection, such as scikit-learn. After the installation is complete, you can use the following code to import the relevant modules:
from sklearn.ensemble import IsolationForest
Then, we can define a function to perform the anomaly detection algorithm and save the results to a new field.
def anomaly_detection(data): # 选择要使用的特征 X = data[['feature1', 'feature2', 'feature3']] # 构建孤立森林模型 model = IsolationForest(contamination=0.1) # 拟合模型 model.fit(X) # 预测异常值 data['is_anomaly'] = model.predict(X) return data
4. Real-time anomaly detection
In order to realize the real-time anomaly detection function, we can use MongoDB's "watch" method to monitor changes in the data collection and insert new documents every time Perform anomaly detection.
while True: # 监控数据集合的变化 with db.data.watch() as stream: for change in stream: # 获取新插入的文档 new_document = change['fullDocument'] # 执行异常检测 new_document = anomaly_detection(new_document) # 更新文档 db.data.update_one({'_id': new_document['_id']}, {'$set': new_document})
The above code will continuously monitor changes in the data collection, perform anomaly detection every time a new document is inserted, and update the detection results to the document.
Summary:
This article introduces how to implement real-time anomaly detection of data in MongoDB. Through the steps of data collection and storage, data preprocessing, anomaly detection algorithms, and real-time detection, we can quickly build a simple anomaly detection system. Of course, in practical applications, the algorithm can also be optimized and adjusted according to specific needs to improve detection accuracy and efficiency.
The above is the detailed content of How to implement real-time anomaly detection of data in MongoDB. For more information, please follow other related articles on the PHP Chinese website!

Hot AI Tools

Undresser.AI Undress
AI-powered app for creating realistic nude photos

AI Clothes Remover
Online AI tool for removing clothes from photos.

Undress AI Tool
Undress images for free

Clothoff.io
AI clothes remover

Video Face Swap
Swap faces in any video effortlessly with our completely free AI face swap tool!

Hot Article

Hot Tools

Notepad++7.3.1
Easy-to-use and free code editor

SublimeText3 Chinese version
Chinese version, very easy to use

Zend Studio 13.0.1
Powerful PHP integrated development environment

Dreamweaver CS6
Visual web development tools

SublimeText3 Mac version
God-level code editing software (SublimeText3)

Hot Topics










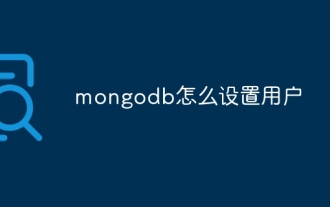
To set up a MongoDB user, follow these steps: 1. Connect to the server and create an administrator user. 2. Create a database to grant users access. 3. Use the createUser command to create a user and specify their role and database access rights. 4. Use the getUsers command to check the created user. 5. Optionally set other permissions or grant users permissions to a specific collection.
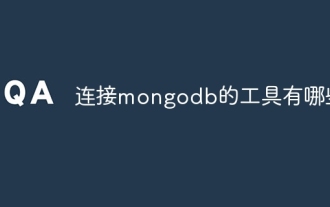
The main tools for connecting to MongoDB are: 1. MongoDB Shell, suitable for quickly viewing data and performing simple operations; 2. Programming language drivers (such as PyMongo, MongoDB Java Driver, MongoDB Node.js Driver), suitable for application development, but you need to master the usage methods; 3. GUI tools (such as Robo 3T, Compass) provide a graphical interface for beginners and quick data viewing. When selecting tools, you need to consider application scenarios and technology stacks, and pay attention to connection string configuration, permission management and performance optimization, such as using connection pools and indexes.
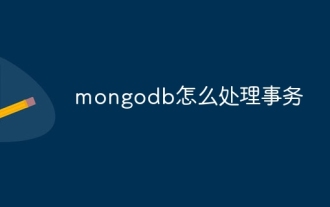
Transaction processing in MongoDB provides solutions such as multi-document transactions, snapshot isolation, and external transaction managers to achieve transaction behavior, ensure multiple operations are executed as one atomic unit, ensuring atomicity and isolation. Suitable for applications that need to ensure data integrity, prevent concurrent operational data corruption, or implement atomic updates in distributed systems. However, its transaction processing capabilities are limited and are only suitable for a single database instance. Multi-document transactions only support read and write operations. Snapshot isolation does not provide atomic guarantees. Integrating external transaction managers may also require additional development work.
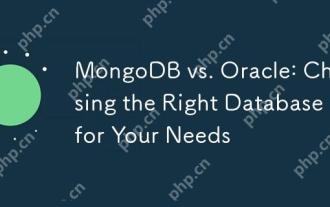
MongoDB is suitable for unstructured data and high scalability requirements, while Oracle is suitable for scenarios that require strict data consistency. 1.MongoDB flexibly stores data in different structures, suitable for social media and the Internet of Things. 2. Oracle structured data model ensures data integrity and is suitable for financial transactions. 3.MongoDB scales horizontally through shards, and Oracle scales vertically through RAC. 4.MongoDB has low maintenance costs, while Oracle has high maintenance costs but is fully supported.
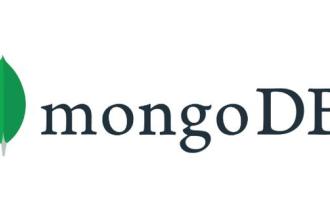
Choosing MongoDB or relational database depends on application requirements. 1. Relational databases (such as MySQL) are suitable for applications that require high data integrity and consistency and fixed data structures, such as banking systems; 2. NoSQL databases such as MongoDB are suitable for processing massive, unstructured or semi-structured data and have low requirements for data consistency, such as social media platforms. The final choice needs to weigh the pros and cons and decide based on the actual situation. There is no perfect database, only the most suitable database.
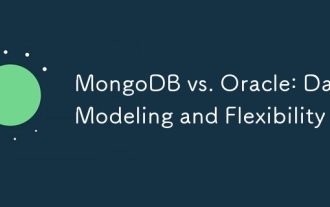
MongoDB is more suitable for processing unstructured data and rapid iteration, while Oracle is more suitable for scenarios that require strict data consistency and complex queries. 1.MongoDB's document model is flexible and suitable for handling complex data structures. 2. Oracle's relationship model is strict to ensure data consistency and complex query performance.
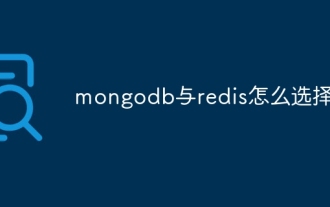
Choose MongoDB or Redis according to application requirements: MongoDB is suitable for storing complex data, and Redis is suitable for fast access to key-value pairs and caches. MongoDB uses document data models, provides persistent storage, and horizontal scalability; while Redis uses key values to perform well and cost-effectively. The final choice depends on the specific needs of the application, such as data type, performance requirements, scalability, and reliability.
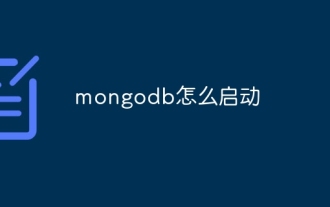
To start the MongoDB server: On a Unix system, run the mongod command. On Windows, run the mongod.exe command. Optional: Set the configuration using the --dbpath, --port, --auth, or --replSet options. Use the mongo command to verify that the connection is successful.
