


How to implement gradient descent algorithm in Python to find local minima?
Gradient descent is an important optimization method in machine learning, used to minimize the loss function of the model. In layman's terms, it requires repeatedly changing the parameters of the model until the ideal value range that minimizes the loss function is found. The method works by taking tiny steps in the direction of the negative gradient of the loss function, or more specifically, along the path of steepest descent. The learning rate is a hyperparameter that regulates the trade-off between algorithm speed and accuracy, and it affects the size of the step size. Many machine learning methods, including linear regression, logistic regression, and neural networks, to name a few, employ gradient descent. Its main application is model training, where the goal is to minimize the difference between the expected and actual values of the target variable. In this article, we will look at implementing gradient descent in Python to find local minima.
Now it’s time to implement gradient descent in Python. Here's a basic description of how we implement it -
First, we import the necessary libraries.
Define its function and its derivatives.
Next, we will apply the gradient descent function.
After applying the function, we will set the parameters to find the local minimum,
Finally, we will plot the output.
Implementing Gradient Descent in Python
Import library
import numpy as np import matplotlib.pyplot as plt
Then we define the function f(x) and its derivative f'(x) -
def f(x): return x**2 - 4*x + 6 def df(x): return 2*x - 4
F(x) is the function that must be reduced and df is its derivative (x). The gradient descent method uses derivatives to guide itself toward the minimum by revealing the slope of the function along the way.
Then define the gradient descent function.
def gradient_descent(initial_x, learning_rate, num_iterations): x = initial_x x_history = [x] for i in range(num_iterations): gradient = df(x) x = x - learning_rate * gradient x_history.append(x) return x, x_history
The starting value of x, the learning rate and the required number of iterations are sent to the gradient descent function. To save the value of x after each iteration, it initializes x to its original value and generates an empty list. The method then performs gradient descent for the provided number of iterations, changing x in each iteration according to the equation x = x - learning rate * gradient. This function generates a list of x values for each iteration and the final value of x.
The gradient descent function can now be used to locate the local minimum of f(x) -
Example
initial_x = 0 learning_rate = 0.1 num_iterations = 50 x, x_history = gradient_descent(initial_x, learning_rate, num_iterations) print("Local minimum: {:.2f}".format(x))
Output
Local minimum: 2.00
In this figure, x is initially set to 0, the learning rate is 0.1, and 50 iterations are run. Finally, we publish the value of x, which should be close to the local minimum at x=2.
Plotting the function f(x) and the x value for each iteration allows us to see the gradient descent process in action -
Example
# Create a range of x values to plot x_vals = np.linspace(-1, 5, 100) # Plot the function f(x) plt.plot(x_vals, f(x_vals)) # Plot the values of x at each iteration plt.plot(x_history, f(np.array(x_history)), 'rx') # Label the axes and add a title plt.xlabel('x') plt.ylabel('f(x)') plt.title('Gradient Descent') # Show the plot plt.show()
Output
in conclusion
In summary, to find the local minimum of a function, Python utilizes an efficient optimization process called gradient descent. Gradient descent works by computing the derivative of a function at each step, repeatedly updating input values in the direction of steepest descent until the lowest value is reached. Implementing gradient descent in Python requires specifying the function to be optimized and its derivatives, initializing input values, and determining the learning rate and number of iterations of the algorithm. After optimization is complete, the method can be evaluated by tracing its steps to the minimum and seeing how it reaches that goal. Gradient descent is a useful technique in machine learning and optimization applications because Python can handle large data sets and complex functions.
The above is the detailed content of How to implement gradient descent algorithm in Python to find local minima?. For more information, please follow other related articles on the PHP Chinese website!

Hot AI Tools

Undresser.AI Undress
AI-powered app for creating realistic nude photos

AI Clothes Remover
Online AI tool for removing clothes from photos.

Undress AI Tool
Undress images for free

Clothoff.io
AI clothes remover

Video Face Swap
Swap faces in any video effortlessly with our completely free AI face swap tool!

Hot Article

Hot Tools

Notepad++7.3.1
Easy-to-use and free code editor

SublimeText3 Chinese version
Chinese version, very easy to use

Zend Studio 13.0.1
Powerful PHP integrated development environment

Dreamweaver CS6
Visual web development tools

SublimeText3 Mac version
God-level code editing software (SublimeText3)

Hot Topics










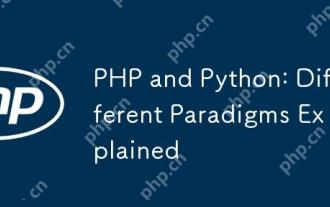
PHP is mainly procedural programming, but also supports object-oriented programming (OOP); Python supports a variety of paradigms, including OOP, functional and procedural programming. PHP is suitable for web development, and Python is suitable for a variety of applications such as data analysis and machine learning.
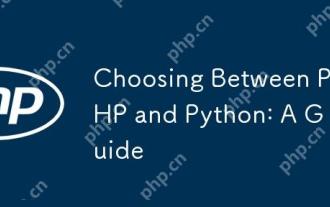
PHP is suitable for web development and rapid prototyping, and Python is suitable for data science and machine learning. 1.PHP is used for dynamic web development, with simple syntax and suitable for rapid development. 2. Python has concise syntax, is suitable for multiple fields, and has a strong library ecosystem.
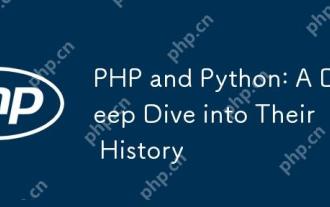
PHP originated in 1994 and was developed by RasmusLerdorf. It was originally used to track website visitors and gradually evolved into a server-side scripting language and was widely used in web development. Python was developed by Guidovan Rossum in the late 1980s and was first released in 1991. It emphasizes code readability and simplicity, and is suitable for scientific computing, data analysis and other fields.
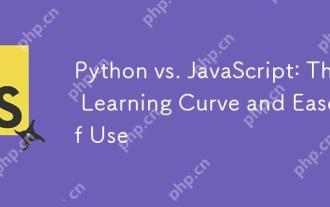
Python is more suitable for beginners, with a smooth learning curve and concise syntax; JavaScript is suitable for front-end development, with a steep learning curve and flexible syntax. 1. Python syntax is intuitive and suitable for data science and back-end development. 2. JavaScript is flexible and widely used in front-end and server-side programming.
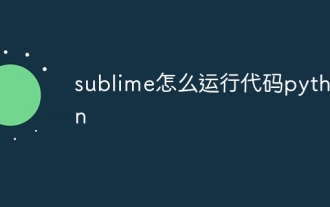
To run Python code in Sublime Text, you need to install the Python plug-in first, then create a .py file and write the code, and finally press Ctrl B to run the code, and the output will be displayed in the console.
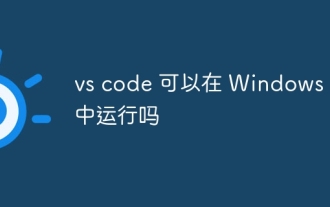
VS Code can run on Windows 8, but the experience may not be great. First make sure the system has been updated to the latest patch, then download the VS Code installation package that matches the system architecture and install it as prompted. After installation, be aware that some extensions may be incompatible with Windows 8 and need to look for alternative extensions or use newer Windows systems in a virtual machine. Install the necessary extensions to check whether they work properly. Although VS Code is feasible on Windows 8, it is recommended to upgrade to a newer Windows system for a better development experience and security.
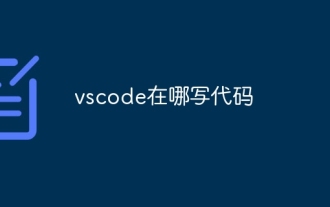
Writing code in Visual Studio Code (VSCode) is simple and easy to use. Just install VSCode, create a project, select a language, create a file, write code, save and run it. The advantages of VSCode include cross-platform, free and open source, powerful features, rich extensions, and lightweight and fast.
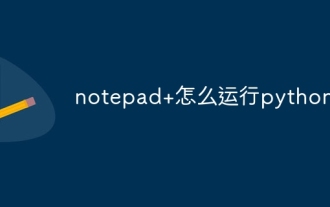
Running Python code in Notepad requires the Python executable and NppExec plug-in to be installed. After installing Python and adding PATH to it, configure the command "python" and the parameter "{CURRENT_DIRECTORY}{FILE_NAME}" in the NppExec plug-in to run Python code in Notepad through the shortcut key "F6".
