


How to implement machine vision algorithms and object recognition in C++?
How to implement machine vision algorithm and object recognition in C?
Introduction:
With the continuous development and application of artificial intelligence, machine vision technology has been widely used in various fields, such as autonomous driving, security monitoring, medical imaging, etc. Among them, C, as a widely used programming language, has the characteristics of high compilation efficiency and strong flexibility, and has gradually become the preferred language for the implementation of machine vision algorithms. This article will introduce how to implement machine vision algorithms and object recognition through C, and attach code examples, hoping to provide some help to readers.
1. Implementation of machine vision algorithm
1.1 Image processing
Image processing is an important part of the machine vision algorithm, mainly including image reading, display, saving and common image processing operations (Such as image binarization, filtering, edge detection, etc.). Next, we will introduce how to use C to implement machine vision algorithms through a simple image processing example.
#include <opencv2/core/core.hpp> #include <opencv2/highgui/highgui.hpp> #include <opencv2/imgproc/imgproc.hpp> int main() { // 读取图像 cv::Mat image = cv::imread("lena.jpg", cv::IMREAD_COLOR); // 图像二值化 cv::Mat grayImage; cv::cvtColor(image, grayImage, cv::COLOR_BGR2GRAY); cv::Mat binaryImage; cv::threshold(grayImage, binaryImage, 128, 255, cv::THRESH_BINARY); // 显示图像 cv::imshow("Binary Image", binaryImage); // 保存图像 cv::imwrite("binary.jpg", binaryImage); // 等待按键退出 cv::waitKey(0); return 0; }
In this example, we used the OpenCV library to read and process images. First, we read the image named "lena.jpg" through the cv::imread
function. Then, we convert the color image into a grayscale image and perform a binarization operation on the grayscale image through the cv::threshold function. Finally, we display the binarized image through the cv::imshow
function, and use the cv::imwrite
function to save the binary image to a file named "binary.jpg" in the file.
1.2 Feature extraction and description
Feature extraction and description is one of the core tasks in machine vision algorithms. It is the process of extracting representative features from images and describing them. In this section we will use the OpenCV library to implement an example of the SIFT (Scale Invariant Feature Transform) algorithm.
#include <opencv2/core/core.hpp> #include <opencv2/highgui/highgui.hpp> #include <opencv2/features2d/features2d.hpp> int main() { // 读取图像 cv::Mat image = cv::imread("lena.jpg", cv::IMREAD_COLOR); // 使用SIFT算法检测图像中的关键点 cv::Ptr<cv::SIFT> sift = cv::SIFT::create(); std::vector<cv::KeyPoint> keypoints; sift->detect(image, keypoints); // 绘制关键点 cv::Mat keypointImage; cv::drawKeypoints(image, keypoints, keypointImage, cv::Scalar::all(-1), cv::DrawMatchesFlags::DRAW_RICH_KEYPOINTS); // 显示图像 cv::imshow("Keypoints", keypointImage); // 等待按键退出 cv::waitKey(0); return 0; }
In this example, we use the cv::SIFT
class in the OpenCV library to implement the SIFT algorithm. First, we read the image named "lena.jpg" through the cv::imread
function. Then, we created a cv::SIFT
objectsift
and used the sift->detect
function to detect key points in the image. Next, we draw the key points on the image through the cv::drawKeypoints
function, and use the cv::imshow
function to display the results.
2. Implementation of object recognition
Object recognition is one of the important applications in machine vision. It completes the object recognition task by matching objects in images with pre-trained models. . In this section we will use the DNN (deep neural network) module in the OpenCV library to implement an example of object recognition.
#include <opencv2/core/utility.hpp> #include <opencv2/core/core.hpp> #include <opencv2/dnn/dnn.hpp> #include <opencv2/highgui/highgui.hpp> #include <opencv2/imgproc/imgproc.hpp> int main() { // 加载模型及相应的配置文件 std::string model = "MobileNetSSD_deploy.caffemodel"; std::string config = "MobileNetSSD_deploy.prototxt"; cv::dnn::Net net = cv::dnn::readNetFromCaffe(config, model); // 加载图像 cv::Mat image = cv::imread("person.jpg", cv::IMREAD_COLOR); // 对图像进行预处理 cv::Mat blob = cv::dnn::blobFromImage(image, 1.0, cv::Size(300, 300), cv::Scalar(127.5, 127.5, 127.5), true, false); // 将blob输入到网络中进行推理 net.setInput(blob); // 获取检测结果 cv::Mat detection = net.forward(); // 解析检测结果 cv::Mat detectionMat(detection.size[2], detection.size[3], CV_32F, detection.ptr<float>()); for (int i = 0; i < detectionMat.rows; i++) { float confidence = detectionMat.at<float>(i, 2); if (confidence > 0.5) { int x1 = static_cast<int>(detectionMat.at<float>(i, 3) * image.cols); int y1 = static_cast<int>(detectionMat.at<float>(i, 4) * image.rows); int x2 = static_cast<int>(detectionMat.at<float>(i, 5) * image.cols); int y2 = static_cast<int>(detectionMat.at<float>(i, 6) * image.rows); // 绘制边界框 cv::rectangle(image, cv::Point(x1, y1), cv::Point(x2, y2), cv::Scalar(0, 255, 0), 2); } } // 显示结果 cv::imshow("Detection", image); // 等待按键退出 cv::waitKey(0); return 0; }
In this example, we use the cv::dnn::Net
class in the OpenCV library to load the model and configuration file, and use cv::imread
The function reads the image named "person.jpg". Next, we preprocess the image through the cv::dnn::blobFromImage
function, and then input the processed data into the network for inference. Finally, we parse the detection results and draw the detected bounding box using the cv::rectangle
function.
Conclusion:
Through the introduction of this article, we have learned how to use C to implement machine vision algorithms and object recognition. From image processing to feature extraction and description, to object recognition, the C and OpenCV libraries provide a wealth of tools and functions to help us implement machine vision algorithms efficiently. I hope this article can provide readers with some help and inspiration in implementing machine vision algorithms and object recognition in C.
The above is the detailed content of How to implement machine vision algorithms and object recognition in C++?. For more information, please follow other related articles on the PHP Chinese website!

Hot AI Tools

Undresser.AI Undress
AI-powered app for creating realistic nude photos

AI Clothes Remover
Online AI tool for removing clothes from photos.

Undress AI Tool
Undress images for free

Clothoff.io
AI clothes remover

Video Face Swap
Swap faces in any video effortlessly with our completely free AI face swap tool!

Hot Article

Hot Tools

Notepad++7.3.1
Easy-to-use and free code editor

SublimeText3 Chinese version
Chinese version, very easy to use

Zend Studio 13.0.1
Powerful PHP integrated development environment

Dreamweaver CS6
Visual web development tools

SublimeText3 Mac version
God-level code editing software (SublimeText3)

Hot Topics
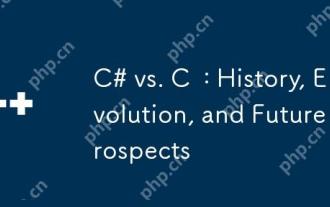
The history and evolution of C# and C are unique, and the future prospects are also different. 1.C was invented by BjarneStroustrup in 1983 to introduce object-oriented programming into the C language. Its evolution process includes multiple standardizations, such as C 11 introducing auto keywords and lambda expressions, C 20 introducing concepts and coroutines, and will focus on performance and system-level programming in the future. 2.C# was released by Microsoft in 2000. Combining the advantages of C and Java, its evolution focuses on simplicity and productivity. For example, C#2.0 introduced generics and C#5.0 introduced asynchronous programming, which will focus on developers' productivity and cloud computing in the future.
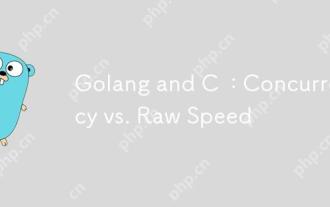
Golang is better than C in concurrency, while C is better than Golang in raw speed. 1) Golang achieves efficient concurrency through goroutine and channel, which is suitable for handling a large number of concurrent tasks. 2)C Through compiler optimization and standard library, it provides high performance close to hardware, suitable for applications that require extreme optimization.
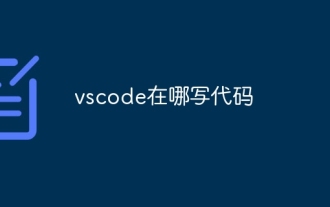
Writing code in Visual Studio Code (VSCode) is simple and easy to use. Just install VSCode, create a project, select a language, create a file, write code, save and run it. The advantages of VSCode include cross-platform, free and open source, powerful features, rich extensions, and lightweight and fast.
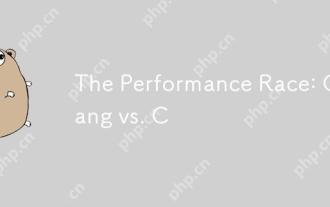
Golang and C each have their own advantages in performance competitions: 1) Golang is suitable for high concurrency and rapid development, and 2) C provides higher performance and fine-grained control. The selection should be based on project requirements and team technology stack.
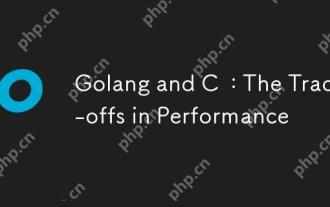
The performance differences between Golang and C are mainly reflected in memory management, compilation optimization and runtime efficiency. 1) Golang's garbage collection mechanism is convenient but may affect performance, 2) C's manual memory management and compiler optimization are more efficient in recursive computing.
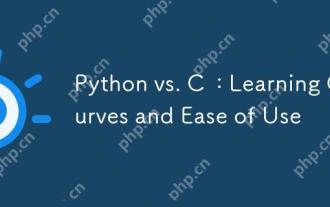
Python is easier to learn and use, while C is more powerful but complex. 1. Python syntax is concise and suitable for beginners. Dynamic typing and automatic memory management make it easy to use, but may cause runtime errors. 2.C provides low-level control and advanced features, suitable for high-performance applications, but has a high learning threshold and requires manual memory and type safety management.
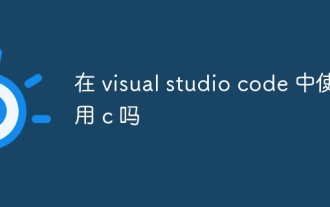
Writing C in VS Code is not only feasible, but also efficient and elegant. The key is to install the excellent C/C extension, which provides functions such as code completion, syntax highlighting, and debugging. VS Code's debugging capabilities help you quickly locate bugs, while printf output is an old-fashioned but effective debugging method. In addition, when dynamic memory allocation, the return value should be checked and memory freed to prevent memory leaks, and debugging these issues is convenient in VS Code. Although VS Code cannot directly help with performance optimization, it provides a good development environment for easy analysis of code performance. Good programming habits, readability and maintainability are also crucial. Anyway, VS Code is
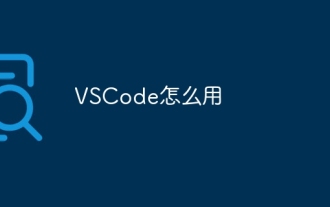
Visual Studio Code (VSCode) is a cross-platform, open source and free code editor developed by Microsoft. It is known for its lightweight, scalability and support for a wide range of programming languages. To install VSCode, please visit the official website to download and run the installer. When using VSCode, you can create new projects, edit code, debug code, navigate projects, expand VSCode, and manage settings. VSCode is available for Windows, macOS, and Linux, supports multiple programming languages and provides various extensions through Marketplace. Its advantages include lightweight, scalability, extensive language support, rich features and version
