


How to improve multi-threaded concurrency efficiency in C++ big data development?
How to improve multi-threaded concurrency efficiency in C big data development?
Introduction:
In the field of modern big data, the scale and complexity of data volume Growing exponentially, the ability to process data efficiently becomes critical. In C, multi-thread concurrency is one of the important means to improve the efficiency of big data development. This article will discuss how to use multi-thread concurrency to improve the efficiency of C big data development, and give corresponding code examples.
1. Understand the basic concepts of multi-thread concurrency:
Multi-thread concurrency refers to running multiple threads at the same time, each thread performing different tasks. Multi-thread concurrency can make full use of the multi-core characteristics of the CPU and improve the running efficiency of the program. In C, multi-thread concurrency is achieved by creating and starting multiple threads.
2. Key technologies for multi-thread concurrency:
- Thread creation and startup:
In C, you can use the thread library to create and start threads. The following is a simple sample code for thread creation and startup:
#include <iostream> #include <thread> // 线程任务函数 void thread_func() { // 线程具体任务代码 std::cout << "Hello, World!" << std::endl; } int main() { // 创建线程并启动 std::thread t(thread_func); // 等待线程结束 t.join(); return 0; }
- Thread synchronization and mutual exclusion:
In multi-threaded concurrent operations, multiple threads often access the share at the same time In the case of data, a mutex lock needs to be used to ensure data consistency. The following is a simple example code using a mutex lock:
#include <iostream> #include <thread> #include <mutex> std::mutex mtx; // 全局互斥锁 // 线程任务函数 void thread_func() { std::lock_guard<std::mutex> lock(mtx); // 加锁 // 具体任务代码 std::cout << "Hello, World!" << std::endl; // 解锁 } int main() { // 创建线程并启动 std::thread t(thread_func); // 等待线程结束 t.join(); return 0; }
- Data fragmentation and fragmentation processing:
In big data scenarios, data is usually divided into multiple Fragments are processed, and different threads are responsible for processing different data fragments, thereby improving processing efficiency. The following is a simple example code for data sharding processing:
#include <iostream> #include <thread> #include <vector> #include <algorithm> const int num_threads = 4; // 线程数量 // 线程任务函数 void thread_func(int thread_id, std::vector<int>& data) { int start = thread_id * (data.size() / num_threads); int end = (thread_id == num_threads - 1) ? data.size() : (thread_id + 1) * (data.size() / num_threads); for (int i = start; i < end; ++i) { // 具体任务代码 data[i] *= 2; } } int main() { std::vector<int> data = {1, 2, 3, 4, 5, 6, 7, 8, 9, 10}; std::vector<std::thread> threads; // 创建线程并启动 for (int i = 0; i < num_threads; ++i) { threads.emplace_back(thread_func, i, std::ref(data)); } // 等待线程结束 for (int i = 0; i < num_threads; ++i) { threads[i].join(); } // 输出结果 for (int num : data) { std::cout << num << " "; } std::cout << std::endl; return 0; }
3. Summary:
By rationally utilizing multi-threaded concurrency technology, the processing efficiency of C big data development can be improved. In practical applications, in addition to the basic technologies such as thread creation and startup, thread synchronization and mutual exclusion, data sharding and shard processing introduced above, there are many other optimization techniques and strategies, which need to be selected and selected according to specific scenarios. application.
In short, effective use of multi-thread concurrency, combined with reasonable algorithms and data processing methods, can bring significant efficiency improvements to C big data development. I hope the content of this article can inspire and help big data developers.
The above is the detailed content of How to improve multi-threaded concurrency efficiency in C++ big data development?. For more information, please follow other related articles on the PHP Chinese website!

Hot AI Tools

Undresser.AI Undress
AI-powered app for creating realistic nude photos

AI Clothes Remover
Online AI tool for removing clothes from photos.

Undress AI Tool
Undress images for free

Clothoff.io
AI clothes remover

Video Face Swap
Swap faces in any video effortlessly with our completely free AI face swap tool!

Hot Article

Hot Tools

Notepad++7.3.1
Easy-to-use and free code editor

SublimeText3 Chinese version
Chinese version, very easy to use

Zend Studio 13.0.1
Powerful PHP integrated development environment

Dreamweaver CS6
Visual web development tools

SublimeText3 Mac version
God-level code editing software (SublimeText3)

Hot Topics
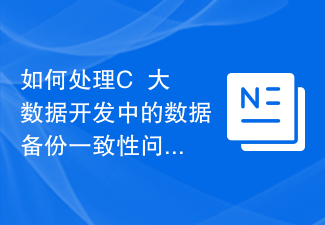
How to deal with the data backup consistency problem in C++ big data development? In C++ big data development, data backup is a very important part. In order to ensure the consistency of data backup, we need to take a series of measures to solve this problem. This article will discuss how to deal with data backup consistency issues in C++ big data development and provide corresponding code examples. Using transactions for data backup Transactions are a mechanism to ensure the consistency of data operations. In C++, we can use the transaction concept in the database to implement data backup.
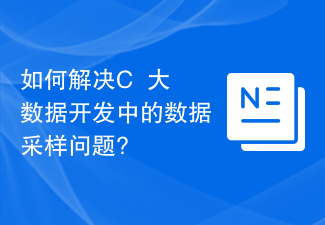
How to solve the data sampling problem in C++ big data development? In C++ big data development, the amount of data is often very large. In the process of processing these big data, a very common problem is how to sample the big data. Sampling is to select a part of sample data from a big data collection for analysis and processing, which can greatly reduce the amount of calculation and increase the processing speed. Below we will introduce several methods to solve the data sampling problem in C++ big data development, and attach code examples. 1. Simple random sampling Simple random sampling is the most common
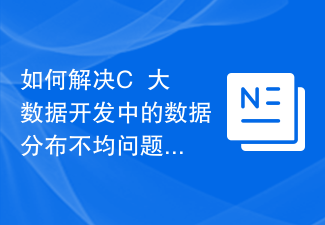
How to solve the problem of uneven data distribution in C++ big data development? In the C++ big data development process, uneven data distribution is a common problem. When the distribution of data is uneven, it will lead to inefficient data processing or even failure to complete the task. Therefore, solving the problem of uneven data distribution is the key to improving big data processing capabilities. So, how to solve the problem of uneven data distribution in C++ big data development? Some solutions are provided below, along with code examples to help readers understand and practice. Data Sharding Algorithm Data Sharding Algorithm is
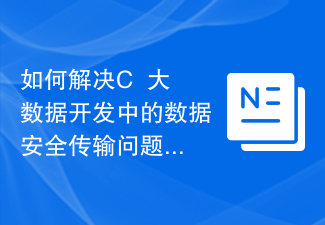
How to solve the problem of data security transmission in C++ big data development? With the rapid development of big data, data security transmission has become an issue that cannot be ignored during the development process. In C++ development, we can ensure the security of data during transmission through encryption algorithms and transmission protocols. This article will introduce how to solve the problem of data security transmission in C++ big data development and provide sample code. 1. Data encryption algorithm C++ provides a rich encryption algorithm library, such as OpenSSL, Crypto++, etc. These libraries can be used
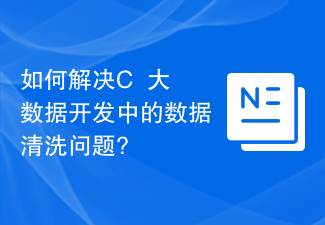
How to solve the data cleaning problem in C++ big data development? Introduction: In big data development, data cleaning is a very important step. Correct, complete, and structured data are the basis for algorithm analysis and model training. This article will introduce how to use C++ to solve data cleaning problems in big data development, and give specific implementation methods through code examples. 1. The concept of data cleaning Data cleaning refers to the preprocessing of original data to make it suitable for subsequent analysis and processing. Mainly includes the following aspects: Missing value processing: deleting or filling missing values
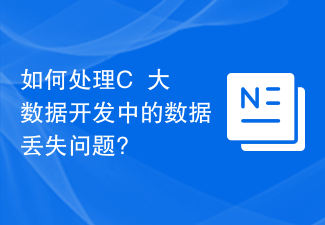
How to deal with the data loss problem in C++ big data development? With the advent of the big data era, more and more companies and developers are beginning to pay attention to big data development. As an efficient and widely used programming language, C++ has also begun to play an important role in big data processing. However, in C++ big data development, the problem of data loss often causes headaches. This article will introduce some common data loss problems and solutions, and provide relevant code examples. Sources of Data Loss Issues Data loss issues can arise from many sources, here are a few
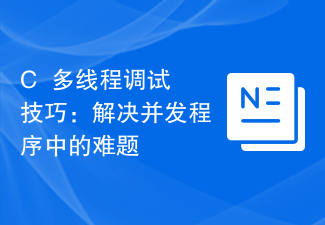
Multi-threaded programming has become more and more common in today's software development world. By using multi-threaded programming, we can better utilize the multi-core processing power of modern computers, thereby improving the performance of concurrent programs. However, multi-threaded programming also comes with some challenges, one of the biggest being debugging. In multi-threaded programs, the cause of an error can become very difficult to track and locate due to interactions and race conditions between threads. Therefore, it is very important to master some debugging skills. First, to better debug multi-threaded programs, I
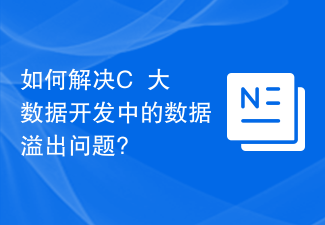
How to solve the data overflow problem in C++ big data development? In the process of C++ big data development, we often encounter the problem of data overflow. Data overflow means that when the value of data exceeds the range that its variable type can represent, it will lead to erroneous results or unpredictable program behavior. In order to solve this problem, we need to take some measures to ensure that the data does not overflow during the calculation process. 1. Choose the appropriate data type In C++, the choice of data type is very important to avoid data overflow problems. According to actual needs, we should
