


How amazing is the simple speech conversion model that supports cross-language, human voice and dog barking interchange, and only uses nearest neighbors?
The voice world in which AI participates is really magical. It can not only change one person's voice to that of any other person, but also exchange voices with animals.
We know that the goal of speech conversion is to convert the source speech into the target speech while keeping the content unchanged. Recent any-to-any speech conversion methods improve naturalness and speaker similarity, but at the expense of greatly increased complexity. This means that training and inference become more expensive, making improvements difficult to evaluate and establish.
The question is, does high-quality speech conversion require complexity? In a recent paper from the University of Stellenbosch in South Africa, several researchers explored this issue.
- ##Paper address: https://arxiv.org/pdf/2305.18975.pdf
- GitHub address: https://bshall.github.io/knn-vc/
The research highlights are: They introduced K nearest neighbor speech conversion ( kNN-VC), a simple and powerful any-to-any speech conversion method. Instead of training an explicit transformation model, K-nearest neighbor regression is simply used.
Specifically, the researchers first used a self-supervised speech representation model to extract the feature sequence of the source utterance and the reference utterance, and then replaced each frame of the source representation with one in the reference. nearest neighbor to convert to the target speaker, and finally use a neural vocoder to synthesize the converted features to obtain the converted speech.
From the results, despite its simplicity, KNN-VC achieves comparable or even improved intelligibility in both subjective and objective evaluations compared to several baseline speech conversion systems Similarity to the speaker.
Let’s appreciate the effect of KNN-VC voice conversion. Looking first at human voice conversion, KNN-VC is applied to source and target speakers unseen in the LibriSpeech dataset.
Source voice00:11
##Synthetic voice 100:11
Synthetic Speech 200:11
KNN-VC also supports cross-language speech conversion, such as Spanish to German, German to Japanese, Chinese to Spanish.
Source Chinese00:08
Destination Spanish00:05
Synthetic Speech 300:08
What’s even more amazing is that KNN-VC can also combine human voices with dogs Bark swap.
Source Dog Barking00:09
Source Human Voice00:05
Synthetic Voice 400:08
##Synthetic Voice 500:05We next look at how KNN-VC runs and compares it with other jixian methods.
Method Overview and Experimental Results
The architecture diagram of kNN-VC is as shown below, following the encoder-converter-vocoder structure. First the encoder extracts self-supervised representations of the source and reference speech, then the converter maps each source frame to their nearest neighbor in the reference, and finally the vocoder generates audio waveforms based on the converted features.
The encoder uses WavLM, the converter uses K nearest neighbor regression, and the vocoder uses HiFiGAN. The only component that requires training is the vocoder.
For the WavLM encoder, the researcher only used the pre-trained WavLM-Large model and did not do any training on it in the article. For the kNN transformation model, kNN is non-parametric and does not require any training. For the HiFiGAN vocoder, the original HiFiGAN author's repo was used to vocode the WavLM features, becoming the only part that required training.
Picture
In the experiment, the researchers first compared KNN-VC with other baseline methods, using the maximum available Target data (approximately 8 minutes of audio per speaker) to test the speech conversion system.
For KNN-VC, the researcher uses all target data as the matching set. For the baseline method, they average speaker embeddings for each target utterance.
Table 1 below reports the results for intelligibility, naturalness, and speaker similarity for each model. As can be seen, kNN-VC achieves similar naturalness and clarity to the best baseline FreeVC, but with significantly improved speaker similarity. This also confirms the assertion of this article: high-quality speech conversion does not require increased complexity.
In addition, the researchers wanted to understand how much of the improvement was due to HiFi-GAN trained on pre-matched data, as well as target speaker data How much does size affect intelligibility and speaker similarity.
Figure 2 below shows the relationship between WER (smaller is better) and EER (higher is better) for two HiFi-GAN variants at different target speaker sizes.
Picture
Netizen Comments
For this "only use nearest neighbors" "'s new speech conversion method kNN-VC, some people think that the pre-trained speech model is used in the article, so using "only" is not accurate. But it is undeniable that kNN-VC is still simpler than other models.
The results also demonstrate that kNN-VC is equally effective, if not the best, compared to very complex any-to-any speech conversion methods.
Picture
Some people also said that the example of interchange of human voice and dog barking is very interesting.
picture
The above is the detailed content of How amazing is the simple speech conversion model that supports cross-language, human voice and dog barking interchange, and only uses nearest neighbors?. For more information, please follow other related articles on the PHP Chinese website!

Hot AI Tools

Undresser.AI Undress
AI-powered app for creating realistic nude photos

AI Clothes Remover
Online AI tool for removing clothes from photos.

Undress AI Tool
Undress images for free

Clothoff.io
AI clothes remover

Video Face Swap
Swap faces in any video effortlessly with our completely free AI face swap tool!

Hot Article

Hot Tools

Notepad++7.3.1
Easy-to-use and free code editor

SublimeText3 Chinese version
Chinese version, very easy to use

Zend Studio 13.0.1
Powerful PHP integrated development environment

Dreamweaver CS6
Visual web development tools

SublimeText3 Mac version
God-level code editing software (SublimeText3)

Hot Topics
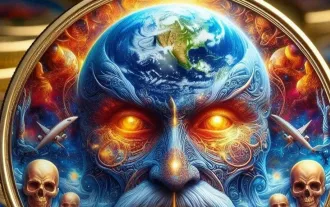
WorldCoin (WLD) stands out in the cryptocurrency market with its unique biometric verification and privacy protection mechanisms, attracting the attention of many investors. WLD has performed outstandingly among altcoins with its innovative technologies, especially in combination with OpenAI artificial intelligence technology. But how will the digital assets behave in the next few years? Let's predict the future price of WLD together. The 2025 WLD price forecast is expected to achieve significant growth in WLD in 2025. Market analysis shows that the average WLD price may reach $1.31, with a maximum of $1.36. However, in a bear market, the price may fall to around $0.55. This growth expectation is mainly due to WorldCoin2.
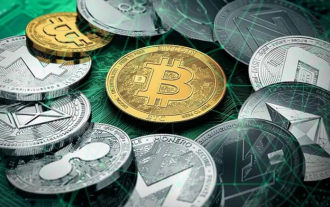
Factors of rising virtual currency prices include: 1. Increased market demand, 2. Decreased supply, 3. Stimulated positive news, 4. Optimistic market sentiment, 5. Macroeconomic environment; Decline factors include: 1. Decreased market demand, 2. Increased supply, 3. Strike of negative news, 4. Pessimistic market sentiment, 5. Macroeconomic environment.
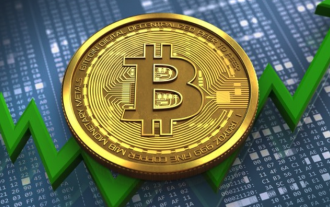
Exchanges that support cross-chain transactions: 1. Binance, 2. Uniswap, 3. SushiSwap, 4. Curve Finance, 5. Thorchain, 6. 1inch Exchange, 7. DLN Trade, these platforms support multi-chain asset transactions through various technologies.
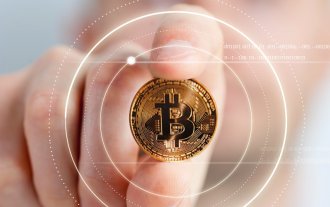
The steps to draw a Bitcoin structure analysis chart include: 1. Determine the purpose and audience of the drawing, 2. Select the right tool, 3. Design the framework and fill in the core components, 4. Refer to the existing template. Complete steps ensure that the chart is accurate and easy to understand.
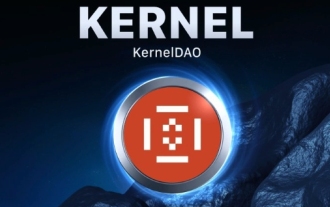
In the bustling world of cryptocurrencies, new opportunities always emerge. At present, KernelDAO (KERNEL) airdrop activity is attracting much attention and attracting the attention of many investors. So, what is the origin of this project? What benefits can BNB Holder get from it? Don't worry, the following will reveal it one by one for you.

Aavenomics is a proposal to modify the AAVE protocol token and introduce token repos, which has implemented a quorum for AAVEDAO. Marc Zeller, founder of the AAVE Project Chain (ACI), announced this on X, noting that it marks a new era for the agreement. Marc Zeller, founder of the AAVE Chain Initiative (ACI), announced on X that the Aavenomics proposal includes modifying the AAVE protocol token and introducing token repos, has achieved a quorum for AAVEDAO. According to Zeller, this marks a new era for the agreement. AaveDao members voted overwhelmingly to support the proposal, which was 100 per week on Wednesday
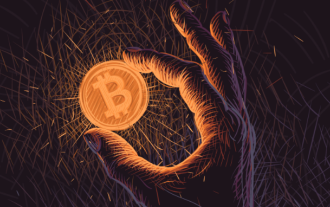
The platforms that have outstanding performance in leveraged trading, security and user experience in 2025 are: 1. OKX, suitable for high-frequency traders, providing up to 100 times leverage; 2. Binance, suitable for multi-currency traders around the world, providing 125 times high leverage; 3. Gate.io, suitable for professional derivatives players, providing 100 times leverage; 4. Bitget, suitable for novices and social traders, providing up to 100 times leverage; 5. Kraken, suitable for steady investors, providing 5 times leverage; 6. Bybit, suitable for altcoin explorers, providing 20 times leverage; 7. KuCoin, suitable for low-cost traders, providing 10 times leverage; 8. Bitfinex, suitable for senior play
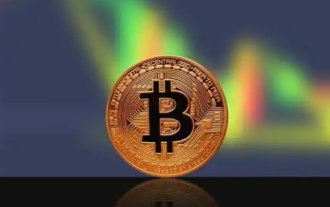
Cryptocurrency data platforms suitable for beginners include CoinMarketCap and non-small trumpet. 1. CoinMarketCap provides global real-time price, market value, and trading volume rankings for novice and basic analysis needs. 2. The non-small quotation provides a Chinese-friendly interface, suitable for Chinese users to quickly screen low-risk potential projects.
