


Google's DeepMind has developed the RoboCat AI model, which can control a variety of robots to perform a series of tasks
On June 26, Google’s DeepMind said that the company has developed an artificial intelligence model called RoboCat that can control different robot arms to perform a series of tasks. This alone isn't particularly novel, but DeepMind claims that the model is the first to be able to solve and adapt to a variety of tasks, and to do so using different, real-world robots.
RoboCat was inspired by another DeepMind AI model, Gato, which can analyze and process text, images and events. RoboCat's training data includes images and motion data of simulated and real robots, derived from other robot control models in virtual environments, human-controlled robots, and previous versions of RoboCat itself.
Alex Lee, a research scientist at DeepMind and one of the collaborators on the RoboCat team, said in an email interview with TechCrunch: "We showed that a single large model can be used on multiple real-world models. The robot physically solves diverse tasks and can quickly adapt to new tasks and entities."
IT House noted that in order to train RoboCat, DeepMind researchers first used human-controlled robotic arms, Between 100 and 1000 demonstrations of each task or robot were collected in simulated or real environments. For example, let a robotic arm pick up gears or stack building blocks. They then fine-tuned RoboCat, creating a specialized "derived" model on each task and letting it practice an average of 10,000 times. By leveraging data generated by derived models and demonstration data, researchers continue to expand RoboCat's training data set and train new versions of RoboCat.
The final version of RoboCat was trained on a total of 253 tasks and tested on 141 variations of these tasks, both in simulation and in the real world. DeepMind claims that RoboCat learned to operate different types of robotic arms after observing 1,000 human-controlled demonstrations collected over several hours. While RoboCat has been trained on four robots with two-finger arms, the model was able to adapt to a more complex arm with a three-finger gripper and twice as many controllable inputs.
Despite this, RoboCat's success rates on different tasks varied greatly in DeepMind's tests, ranging from a low of 13% to a high of 99%. This is with 1000 demonstrations in the training data; if the number of demonstrations is halved, the success rate will decrease accordingly. In some cases, though, DeepMind claims RoboCat can learn new tasks by observing just 100 demonstrations.
Alex Lee believes RoboCat might make it easier to solve new tasks. “Given a certain number of demonstrations of a new task, RoboCat can fine-tune to new tasks and self-generate more data to improve further,” he added.
Going forward, the research team aims to reduce the number of demonstrations needed to teach RoboCat to complete new tasks to less than 10.
The above is the detailed content of Google's DeepMind has developed the RoboCat AI model, which can control a variety of robots to perform a series of tasks. For more information, please follow other related articles on the PHP Chinese website!

Hot AI Tools

Undresser.AI Undress
AI-powered app for creating realistic nude photos

AI Clothes Remover
Online AI tool for removing clothes from photos.

Undress AI Tool
Undress images for free

Clothoff.io
AI clothes remover

Video Face Swap
Swap faces in any video effortlessly with our completely free AI face swap tool!

Hot Article

Hot Tools

Notepad++7.3.1
Easy-to-use and free code editor

SublimeText3 Chinese version
Chinese version, very easy to use

Zend Studio 13.0.1
Powerful PHP integrated development environment

Dreamweaver CS6
Visual web development tools

SublimeText3 Mac version
God-level code editing software (SublimeText3)

Hot Topics
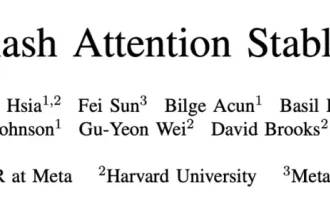
MetaFAIR teamed up with Harvard to provide a new research framework for optimizing the data bias generated when large-scale machine learning is performed. It is known that the training of large language models often takes months and uses hundreds or even thousands of GPUs. Taking the LLaMA270B model as an example, its training requires a total of 1,720,320 GPU hours. Training large models presents unique systemic challenges due to the scale and complexity of these workloads. Recently, many institutions have reported instability in the training process when training SOTA generative AI models. They usually appear in the form of loss spikes. For example, Google's PaLM model experienced up to 20 loss spikes during the training process. Numerical bias is the root cause of this training inaccuracy,
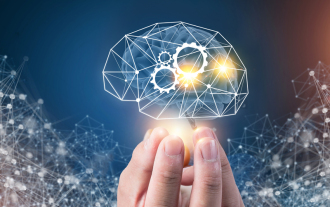
According to news on November 15, Microsoft recently launched a method called "Everything of Thought" (XOT), inspired by Google DeepMind's AlphaZero, which uses compact neural networks to enhance the reasoning capabilities of AI models. Microsoft collaborated with Georgia Institute of Technology and East China Normal University to develop this algorithm, which integrates reinforcement learning and Monte Carlo Tree Search (MCTS) capabilities to further improve the effectiveness of problem solving in complex decision-making environments. Note from this site: The Microsoft research team stated that the XOT method can expand the language model on unfamiliar problems. In Gameof24, 8-Puzzle and P
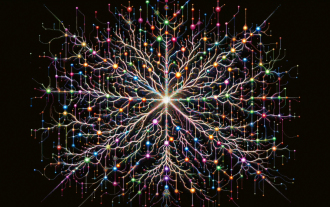
According to news on November 16, leading scientific research institutions in the industry, the US National Supercomputing Center and many leading companies in the AI field have recently jointly established the Trillion Parameter Consortium (TPC). Generated by DALL-E3 According to reports, this site has learned that the TPC Alliance is composed of scientists from laboratories, scientific research institutions, academia and industry around the world. It aims to jointly promote artificial intelligence models for scientific discovery, and pays special attention to having a The TPC Consortium is currently working to develop scalable model architectures and training strategies for mega-models with one trillion parameters or more, while organizing and curating the scientific data used for model training to optimize AI libraries for current and future exascale applications. level computing platform
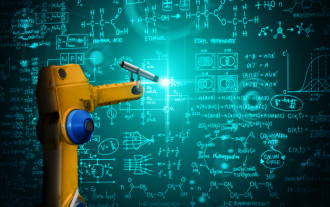
According to news on June 26, DeepMind, a subsidiary of Google, said that the company has developed an artificial intelligence model called RoboCat that can control different robot arms to perform a series of tasks. This alone isn't particularly novel, but DeepMind claims that this model is the first to be able to solve and adapt to a variety of tasks, and to do so using different, real-world robots. RoboCat is inspired by another DeepMind AI model, Gato, which can analyze and process text, images and events. RoboCat's training data includes images and motion data of simulated and real robots, which come from other robot control models in the virtual environment, human-controlled robots
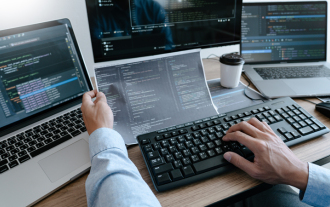
According to news on July 10, Databricks recently released the AI model SDK used by the big data analysis platform Spark. When developers write code, they can give instructions in English, and the compiler will convert the English instructions into PySpark or SQL language codes to improve developers' efficiency. ▲Image source Databricks website It is reported that Spark is an open source big data analysis tool that is downloaded more than 1 billion times a year and is used in 208 countries and regions around the world. ▲Image source Databricks website Databricks said that Microsoft’s AI code assistant GitHubCopilot is powerful, but the threshold for use is also quite high. Databricks’ SDK is relatively more universal and easier to use.
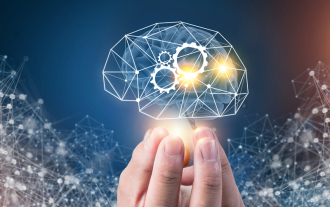
According to news on December 15, Google DeepMind recently announced a model training method called "FunSearch", which claims to be able to calculate a series of "involving the fields of mathematics and computer science" including "upper-level problems" and "boxing problems". complex issues." The content that needs to be rewritten is: ▲Source: Google DeepMind (hereinafter referred to as DeepMind) It is reported that the FunSearch model training method mainly introduces an "Evaluator" system for the AI model, and the AI model outputs a series of "creative problem-solving methods" ", and the "evaluator" is responsible for evaluating the problem-solving methods output by the model. After repeated iterations, an AI model with stronger mathematical capabilities can be trained. Google's DeepM
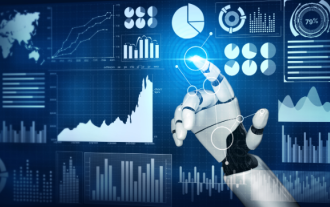
Microsoft announced its AI service terms on August 16 and announced that the terms will take effect on September 30. The main content of this update is for generative AI, especially content related to the use of relevant users and responsible development practices. Microsoft emphasizes that the official will not retain the conversation records of users chatting with Bing, nor will these chat data be used. The five key policy points used to train the AI model for Bing Enterprise Chat cover multiple areas, including prohibiting users from attempting to reverse engineer the AI model to prevent revealing underlying components; prohibiting data extraction through methods such as web scraping unless explicitly allowed; An important clause restricts users from using AI data to create or enhance other AI services. The following is a clause added by Microsoft.
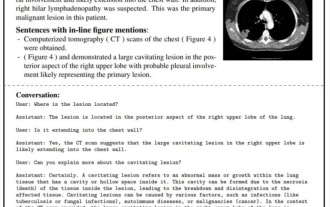
According to news on June 14, Microsoft researchers recently demonstrated the LLaVA-Med model, which is mainly used for biomedical research and can infer the pathological conditions of patients based on CT and X-ray pictures. It is reported that Microsoft researchers have cooperated with a group of hospitals and obtained a large data set corresponding to biomedical image text to train a multi-modal AI model. This data set includes chest X-ray, MRI, histology, pathology and CT images, etc., with relatively comprehensive coverage. ▲Picture source Microsoft Microsoft uses GPT-4, based on VisionTransformer and Vicuna language model, to train LLaVA-Med on eight Nvidia A100 GPUs, which contains "all pre-analysis information for each image",
