A guide to performance testing in Python web development
Python is a very popular programming language used for developing web applications. As Python continues to develop, more and more people are using it to build high-performance web applications. In this process, performance testing has also become a very important link.
Performance testing is to evaluate the performance of a web application or its individual components. It is used to measure the response speed, resource consumption and throughput of web applications under what load. In Python web development, there are also many tools and techniques that can be used for performance testing.
In this article, we will discuss performance testing in Python web development and provide some useful guidelines to help you understand how to perform performance testing of Python web applications.
- Understand the purpose of performance testing
Before performing performance testing of Python web applications, we need to understand the purpose of performance testing. Performance testing is not only to check how quickly an application can respond quickly under load, but also to identify performance bottlenecks and provide solutions to performance bottlenecks.
The purpose of performance testing is not only to check the performance of the application in terms of response speed, but also to evaluate its performance in some aspects, such as memory and CPU usage, network latency and throughput, etc. Therefore, before conducting performance testing, we need to clarify what aspects we want to test and what goals we want to achieve.
- Choose the right performance testing tool
In Python web development, there are many tools to choose from. Some open source tools include:
- Apache JMeter: Apache JMeter is an open source performance testing tool based on Java. It can simulate multiple load types and provides powerful charting and reporting capabilities.
- Locust: Locust is an open source load testing tool written in Python. It supports distributed deployment and provides real-time charts and metrics.
- Grinder: Grinder is a Java-based open source performance testing tool that supports distributed load testing and scripting.
- PyTest-benchmark: It is a Python-based performance testing tool that can be used with PyTest. It provides many test functions and test configurations for easy performance testing.
When choosing a performance testing tool, we need to consider some factors. First, we need to consider the type of load, whether distributed testing or real-time monitoring is required and the type of reports and required data. Secondly, we need to consider the advantages and disadvantages of different tools, such as ease of use, whether programming skills are required, whether it supports Python, etc.
- Optimizing Python Code
Before performing performance testing of Python web applications, we can consider some Python code optimization techniques. Here are some commonly used optimization techniques:
- Use Caching: In Python web applications, we can use caching to reduce database and disk accesses. Caching can help us improve response speed and reduce resource consumption.
- Code Refactoring: In Python web applications, code refactoring can help us identify performance bottlenecks and fundamentally improve code quality.
- Coroutines: In Python 3.5 and above, we can use the async/await keyword to create coroutines to improve code performance.
- Set up benchmark testing
Before conducting performance testing, we need to determine the test benchmark. The test benchmark is the performance standard for the application. We can determine the performance of the application in the load test by comparing the benchmark test results.
Before setting up a benchmark, we should measure the initial performance of the application, which can serve as the basis for the benchmark. We need to consider factors such as load patterns, user behavior, and total data volume while setting up benchmark tests.
- Perform performance testing
After setting up the benchmark test, we can start performance testing. Here are some suggestions:
- Run the test multiple times: After each run of the test, we need to record the test results and run the test multiple times to ensure the reliability of the test results.
- Rolling data: During testing, we need to roll data to avoid excessive data accumulation and uneven load. This can improve the accuracy of the test.
- Monitor system resources: During testing, we need to monitor system resources, such as memory and CPU usage, network bandwidth, I/O operations, etc. This information can help us determine the performance bottlenecks of the application.
- Analyzing test results
After completing the performance test, we need to analyze the test results. We need to compare test results with benchmark results and check if the application performs well under load testing. We can also present data through charts and reports and identify application performance bottlenecks.
- Fix performance bottlenecks
After we identify the performance bottlenecks of our application, we need to take steps to fix the problem. Methods to fix performance bottlenecks may include cache optimization, code refactoring, database optimization, etc. We need to fix the issues one by one and run performance tests again after fixes to ensure that the application's performance under load testing improves.
Summarize
Python is a very popular programming language used for developing web applications. In the Python web development process, performance testing is an indispensable part. Through the guide in this article, you can understand the key steps and techniques for Python web application performance testing, and learn how to optimize Python code, set benchmarks, run performance tests, analyze test results, and fix performance bottlenecks. Hopefully these guidelines will help you improve the performance of your Python web applications.
The above is the detailed content of A guide to performance testing in Python web development. For more information, please follow other related articles on the PHP Chinese website!

Hot AI Tools

Undresser.AI Undress
AI-powered app for creating realistic nude photos

AI Clothes Remover
Online AI tool for removing clothes from photos.

Undress AI Tool
Undress images for free

Clothoff.io
AI clothes remover

Video Face Swap
Swap faces in any video effortlessly with our completely free AI face swap tool!

Hot Article

Hot Tools

Notepad++7.3.1
Easy-to-use and free code editor

SublimeText3 Chinese version
Chinese version, very easy to use

Zend Studio 13.0.1
Powerful PHP integrated development environment

Dreamweaver CS6
Visual web development tools

SublimeText3 Mac version
God-level code editing software (SublimeText3)

Hot Topics










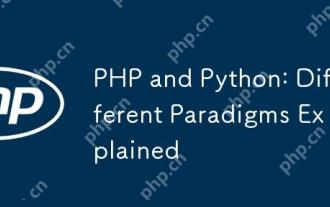
PHP is mainly procedural programming, but also supports object-oriented programming (OOP); Python supports a variety of paradigms, including OOP, functional and procedural programming. PHP is suitable for web development, and Python is suitable for a variety of applications such as data analysis and machine learning.
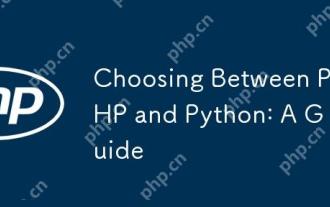
PHP is suitable for web development and rapid prototyping, and Python is suitable for data science and machine learning. 1.PHP is used for dynamic web development, with simple syntax and suitable for rapid development. 2. Python has concise syntax, is suitable for multiple fields, and has a strong library ecosystem.
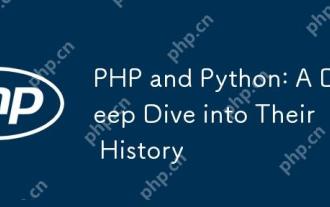
PHP originated in 1994 and was developed by RasmusLerdorf. It was originally used to track website visitors and gradually evolved into a server-side scripting language and was widely used in web development. Python was developed by Guidovan Rossum in the late 1980s and was first released in 1991. It emphasizes code readability and simplicity, and is suitable for scientific computing, data analysis and other fields.
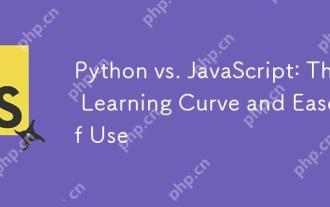
Python is more suitable for beginners, with a smooth learning curve and concise syntax; JavaScript is suitable for front-end development, with a steep learning curve and flexible syntax. 1. Python syntax is intuitive and suitable for data science and back-end development. 2. JavaScript is flexible and widely used in front-end and server-side programming.

The future trends of HTML are semantics and web components, the future trends of CSS are CSS-in-JS and CSSHoudini, and the future trends of JavaScript are WebAssembly and Serverless. 1. HTML semantics improve accessibility and SEO effects, and Web components improve development efficiency, but attention should be paid to browser compatibility. 2. CSS-in-JS enhances style management flexibility but may increase file size. CSSHoudini allows direct operation of CSS rendering. 3.WebAssembly optimizes browser application performance but has a steep learning curve, and Serverless simplifies development but requires optimization of cold start problems.
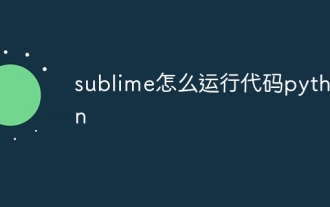
To run Python code in Sublime Text, you need to install the Python plug-in first, then create a .py file and write the code, and finally press Ctrl B to run the code, and the output will be displayed in the console.
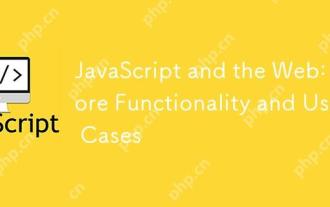
The main uses of JavaScript in web development include client interaction, form verification and asynchronous communication. 1) Dynamic content update and user interaction through DOM operations; 2) Client verification is carried out before the user submits data to improve the user experience; 3) Refreshless communication with the server is achieved through AJAX technology.

The application of React in HTML improves the efficiency and flexibility of web development through componentization and virtual DOM. 1) React componentization idea breaks down the UI into reusable units to simplify management. 2) Virtual DOM optimization performance, minimize DOM operations through diffing algorithm. 3) JSX syntax allows writing HTML in JavaScript to improve development efficiency. 4) Use the useState hook to manage state and realize dynamic content updates. 5) Optimization strategies include using React.memo and useCallback to reduce unnecessary rendering.
