Overview of Transformer technology principles
1. Foreword
Recently, AIGC (AI-Generated Content, artificial intelligence-generated content) has developed rapidly. It is not only sought after by consumers, but also attracts attention from the technology and industry circles. On September 23, 2022, Sequoia America published an article "Generative AI: A Creative New World", believing that AIGC will represent the beginning of a new round of paradigm shift. In October 2022, Stability AI released the open source model Stable Diffusion, which can automatically generate images based on text descriptions (called prompts) entered by users, namely Text-to-Image. Stable Diffusion, DALL-E 2 , Midjourney, Wenxin Yige and other AIGC models that can generate pictures have detonated the field of AI painting. AI painting has become popular, marking the penetration of artificial intelligence into the art field. The picture below shows a work with the theme of "Future Mecha" created by Baidu's "Wenxin Yige" platform.
Figure 1 AI created by Baidu’s “Wenxin Yige” platform Painting
The rapid development of the AIGC field is inseparable from the progress of deep neural networks. Specifically, the emergence of the Transform model gives the neural network more powerful global computing capabilities, reduces network training time, and improves the performance of the network model. The current AIGC domain models that perform relatively well include Attention and Transform technologies in their underlying technical architecture.
2. Development history
2.1 Deep neural network
The development of information technology represented by deep neural networks has promoted the progress and expansion of the field of artificial intelligence. In 2006, Hinton et al. used single-layer RBM autoencoding pre-training to realize deep neural network training; in 2012, the AlexNet neural network model designed by Hinton and Alex Krizhevsky achieved image recognition and classification in the ImageNet competition, becoming a new round of The starting point for the development of artificial intelligence. The currently popular deep neural network is modeled on the concepts proposed by biological neural networks. In biological neural networks, biological neurons transmit received information layer by layer, and the information from multiple neurons is aggregated to obtain the final result. Mathematical models constructed using logical neural units designed analogously to biological neural units are called artificial neural networks. In artificial neural networks, logical neural units are used to explore the hidden relationship between input data and output data. When the amount of data is small, shallow neural networks can meet the requirements of some tasks. However, as the scale of data continues to increase, Expanding, deep neural networks begin to show their unique advantages.
2.2 Attention Mechanism
The attention mechanism (Attention Mechanism) was proposed by the Bengio team in 2014 and has been widely used in depth in recent years. Various fields in learning, such as in computer vision for capturing receptive fields on images, or in NLP for locating key tokens or features. A large number of experiments have proven that models with attention mechanisms have achieved significant improvements in image classification, segmentation, tracking, enhancement, and natural language recognition, understanding, question answering, and translation.
The attention mechanism is modeled after the visual attention mechanism. The visual attention mechanism is an innate ability of the human brain. When we see a picture, we first quickly scan the picture and then focus on the target area that needs to be focused on. For example, when we look at the following pictures, our attention is easily focused on the baby's face, the title of the article, and the first sentence of the article. Just imagine, if every piece of local information is not let go, a lot of energy will be consumed, which is not conducive to the survival and evolution of human beings. Similarly, introducing similar mechanisms into deep learning networks can simplify models and speed up calculations. Essentially understood, Attention is to filter out a small amount of important information from a large amount of information, and focus on this important information, while ignoring most of the unimportant information.
##Figure 2 Schematic diagram of human attention mechanism
3. Technical details
The intelligent Transformer model abandons the traditional CNN and RNN units, and the entire network structure is entirely composed of attention mechanisms. In this chapter, we will first introduce the overall process of the Transformer model, and then introduce in detail the position encoding information and Self-Attention calculation involved.
3.1 Overview of the process
##Figure 3 Attention mechanism flow chart
As shown in the figure above, Transformer consists of two parts: Encoder module and Decoder module. Both Encoder and Decoder contain N block. Taking the translation task as an example, the workflow of Transformer is roughly as follows: Step 1: Obtain the representation vector X of each word of the input sentence. X is obtained by adding the Embedding of the word itself and the Embedding of the word position. Step 2: Pass the obtained word representation vector matrix into the Encoder module. The Encoder module uses the Attention method to calculate the input data. After N Encoder modules, the encoding information matrix of all words in the sentence can be obtained. The matrix dimensions output by each Encoder module are exactly the same as the input. Step 3: Pass the encoding information matrix output by the Encoder module to the Decoder module, and the Decoder will translate the next word i 1 based on the currently translated word i. Like the Encoder structure, the Decoder structure also uses the Attention method for calculation. During use, when translating to word i 1, the words after i 1 need to be covered by the Mask operation.3.2 Self-Attention calculation
The core of the Transform model is attention calculation, which can be expressed by the formula as3.3 Positional encoding
In addition to the Embedding of the word itself, the Transformer also needs to use the position Embedding to represent the position where the word appears in the sentence. Because Transformer does not use the structure of RNN, but uses global information, it cannot use the order information of words, and this part of information is very important for NLP or CV. Therefore, position Embedding is used in Transformer to save the relative or absolute position of the word in the sequence. Position Embedding is represented by PE, and the dimension of PE is the same as the word Embedding. PE can be obtained through training or calculated using a certain formula. The latter is used in Transformer, and the calculation formula is as follows:4. Summary
The focus of Transformer is the Self-Attention structure. Through the multi-dimensional Attention structure, the network can capture the hidden relationships between words in multiple dimensions. However, the Transformer itself cannot use the order information of the words, so it needs to be added to the input. Position Embedding is used to store the position information of the word. Compared with the recurrent neural network, the Transformer network can be trained in parallel better. Compared with the convolutional neural network, the number of operations required by the Transformer network to calculate the association between two positions does not increase with the distance, which can break through the convolutional neural network. The calculated distance is limited to the size of the receptive field. At the same time, the Transformer network can produce more interpretable models. We can examine the attention distribution from the model, and each attention head can learn to perform different tasks.
The above is the detailed content of Overview of Transformer technology principles. For more information, please follow other related articles on the PHP Chinese website!

Hot AI Tools

Undresser.AI Undress
AI-powered app for creating realistic nude photos

AI Clothes Remover
Online AI tool for removing clothes from photos.

Undress AI Tool
Undress images for free

Clothoff.io
AI clothes remover

Video Face Swap
Swap faces in any video effortlessly with our completely free AI face swap tool!

Hot Article

Hot Tools

Notepad++7.3.1
Easy-to-use and free code editor

SublimeText3 Chinese version
Chinese version, very easy to use

Zend Studio 13.0.1
Powerful PHP integrated development environment

Dreamweaver CS6
Visual web development tools

SublimeText3 Mac version
God-level code editing software (SublimeText3)

Hot Topics
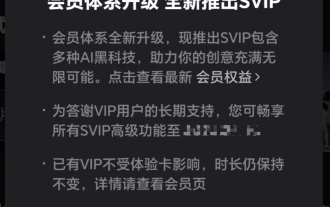
This site reported on June 27 that Jianying is a video editing software developed by FaceMeng Technology, a subsidiary of ByteDance. It relies on the Douyin platform and basically produces short video content for users of the platform. It is compatible with iOS, Android, and Windows. , MacOS and other operating systems. Jianying officially announced the upgrade of its membership system and launched a new SVIP, which includes a variety of AI black technologies, such as intelligent translation, intelligent highlighting, intelligent packaging, digital human synthesis, etc. In terms of price, the monthly fee for clipping SVIP is 79 yuan, the annual fee is 599 yuan (note on this site: equivalent to 49.9 yuan per month), the continuous monthly subscription is 59 yuan per month, and the continuous annual subscription is 499 yuan per year (equivalent to 41.6 yuan per month) . In addition, the cut official also stated that in order to improve the user experience, those who have subscribed to the original VIP
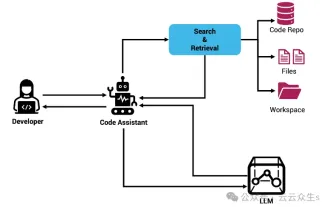
Improve developer productivity, efficiency, and accuracy by incorporating retrieval-enhanced generation and semantic memory into AI coding assistants. Translated from EnhancingAICodingAssistantswithContextUsingRAGandSEM-RAG, author JanakiramMSV. While basic AI programming assistants are naturally helpful, they often fail to provide the most relevant and correct code suggestions because they rely on a general understanding of the software language and the most common patterns of writing software. The code generated by these coding assistants is suitable for solving the problems they are responsible for solving, but often does not conform to the coding standards, conventions and styles of the individual teams. This often results in suggestions that need to be modified or refined in order for the code to be accepted into the application

To learn more about AIGC, please visit: 51CTOAI.x Community https://www.51cto.com/aigc/Translator|Jingyan Reviewer|Chonglou is different from the traditional question bank that can be seen everywhere on the Internet. These questions It requires thinking outside the box. Large Language Models (LLMs) are increasingly important in the fields of data science, generative artificial intelligence (GenAI), and artificial intelligence. These complex algorithms enhance human skills and drive efficiency and innovation in many industries, becoming the key for companies to remain competitive. LLM has a wide range of applications. It can be used in fields such as natural language processing, text generation, speech recognition and recommendation systems. By learning from large amounts of data, LLM is able to generate text
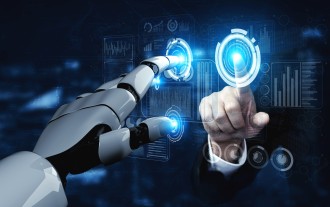
Large Language Models (LLMs) are trained on huge text databases, where they acquire large amounts of real-world knowledge. This knowledge is embedded into their parameters and can then be used when needed. The knowledge of these models is "reified" at the end of training. At the end of pre-training, the model actually stops learning. Align or fine-tune the model to learn how to leverage this knowledge and respond more naturally to user questions. But sometimes model knowledge is not enough, and although the model can access external content through RAG, it is considered beneficial to adapt the model to new domains through fine-tuning. This fine-tuning is performed using input from human annotators or other LLM creations, where the model encounters additional real-world knowledge and integrates it
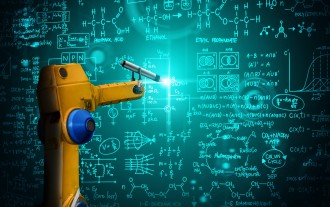
Machine learning is an important branch of artificial intelligence that gives computers the ability to learn from data and improve their capabilities without being explicitly programmed. Machine learning has a wide range of applications in various fields, from image recognition and natural language processing to recommendation systems and fraud detection, and it is changing the way we live. There are many different methods and theories in the field of machine learning, among which the five most influential methods are called the "Five Schools of Machine Learning". The five major schools are the symbolic school, the connectionist school, the evolutionary school, the Bayesian school and the analogy school. 1. Symbolism, also known as symbolism, emphasizes the use of symbols for logical reasoning and expression of knowledge. This school of thought believes that learning is a process of reverse deduction, through existing
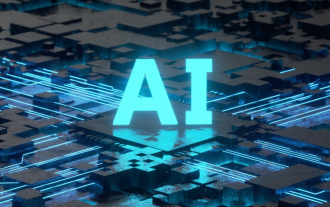
Editor |ScienceAI Question Answering (QA) data set plays a vital role in promoting natural language processing (NLP) research. High-quality QA data sets can not only be used to fine-tune models, but also effectively evaluate the capabilities of large language models (LLM), especially the ability to understand and reason about scientific knowledge. Although there are currently many scientific QA data sets covering medicine, chemistry, biology and other fields, these data sets still have some shortcomings. First, the data form is relatively simple, most of which are multiple-choice questions. They are easy to evaluate, but limit the model's answer selection range and cannot fully test the model's ability to answer scientific questions. In contrast, open-ended Q&A
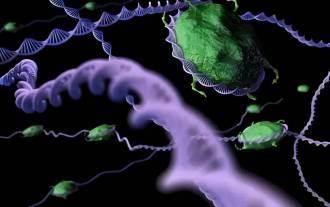
Editor | KX In the field of drug research and development, accurately and effectively predicting the binding affinity of proteins and ligands is crucial for drug screening and optimization. However, current studies do not take into account the important role of molecular surface information in protein-ligand interactions. Based on this, researchers from Xiamen University proposed a novel multi-modal feature extraction (MFE) framework, which for the first time combines information on protein surface, 3D structure and sequence, and uses a cross-attention mechanism to compare different modalities. feature alignment. Experimental results demonstrate that this method achieves state-of-the-art performance in predicting protein-ligand binding affinities. Furthermore, ablation studies demonstrate the effectiveness and necessity of protein surface information and multimodal feature alignment within this framework. Related research begins with "S
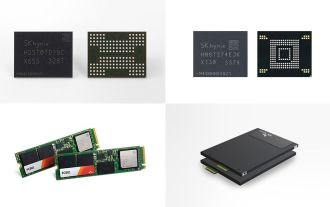
According to news from this site on August 1, SK Hynix released a blog post today (August 1), announcing that it will attend the Global Semiconductor Memory Summit FMS2024 to be held in Santa Clara, California, USA from August 6 to 8, showcasing many new technologies. generation product. Introduction to the Future Memory and Storage Summit (FutureMemoryandStorage), formerly the Flash Memory Summit (FlashMemorySummit) mainly for NAND suppliers, in the context of increasing attention to artificial intelligence technology, this year was renamed the Future Memory and Storage Summit (FutureMemoryandStorage) to invite DRAM and storage vendors and many more players. New product SK hynix launched last year
