


Introducing eight free and open source large model solutions because ChatGPT and Bard are too expensive.
1.LLaMA
The LLaMA project contains a set of basic language models, ranging in size from 7 billion to 65 billion parameters. These models are trained on millions of tokens, and it is trained entirely on publicly available datasets. As a result, LLaMA-13B surpassed GPT-3 (175B), while LLaMA-65B performed similarly to the best models such as Chinchilla-70B and PaLM-540B.
Image from LLaMA
Source:
- Research paper: “LLaMA: Open and Efficient Foundation Language Models (arxiv. org)" [https://arxiv.org/abs/2302.13971]
- GitHub: facebookresearch/llama [https://github.com/facebookresearch/llama]
- Demo: Baize Lora 7B [https://huggingface.co/spaces/project-baize/Baize-7B]
2.Alpaca
Stanford's Alpaca claims it can compete with ChatGPT and anyone can copy it for less than $600. Alpaca 7B is fine-tuned from the LLaMA 7B model on a 52K instruction follow demonstration.
Training content | Pictures from Stanford University CRFM
Resources:
- Blog: Stanford University CRFM. [https://crfm.stanford.edu/2023/03/13/alpaca.html]
- GitHub:tatsu-lab/stanford_alpaca [https://github.com/tatsu-lab/stanford_alpaca]
- Demo: Alpaca-LoRA (the official demo has been lost, this is a rendition of the Alpaca model) [https://huggingface.co/spaces/tloen/alpaca-lora]
3.Vicuna
Vicuna is fine-tuned based on the LLaMA model on user shared conversations collected from ShareGPT. The Vicuna-13B model has reached more than 90% of the quality of OpenAI ChatGPT and Google Bard. It also outperformed the LLaMA and Stanford Alpaca models 90% of the time. The cost to train a Vicuna is approximately $300.
Image from Vicuna
Source:
- Blog post: “Vicuna: An Open-Source Chatbot Impressing GPT-4 with 90%* ChatGPT Quality” [https://vicuna.lmsys.org/]
- GitHub: lm-sys/FastChat [https://github.com/lm-sys/FastChat#fine-tuning ]
- Demo: FastChat (lmsys.org) [https://chat.lmsys.org/]
4.OpenChatKit
OpenChatKit: An open source alternative to ChatGPT, a complete toolkit for creating chatbots. It provides large language models for training users' own instruction adjustments, fine-tuned models, a scalable retrieval system for updating bot responses, and instructions for filtering bot review of questions.
Pictures from TOGETHER
It can be seen that the GPT-NeoXT-Chat-Base-20B model performs better than Basic mode GPT-NoeX.
Resources:
- Blog post: "Announcing OpenChatKit" —TOGETHER [https://www.together.xyz/blog/openchatkit]
- GitHub: togethercomputer /OpenChatKit [https://github.com/togethercomputer/OpenChatKit]
- Demo: OpenChatKit [https://huggingface.co/spaces/togethercomputer/OpenChatKit]
- Model Card: togethercomputer/ GPT-NeoXT-Chat-Base-20B [https://huggingface.co/togethercomputer/GPT-NeoXT-Chat-Base-20B]
##5.GPT4ALL
GPT4ALL is a community-driven project and is trained on a large-scale corpus of auxiliary interactions, including code, stories, descriptions, and multiple rounds of dialogue. The team provided the dataset, model weights, data management process, and training code to facilitate open source. Additionally, they released a quantized 4-bit version of the model that can be run on a laptop. You can even use a Python client to run model inference.- Technical Report: GPT4All [https://s3.amazonaws.com/static.nomic.ai/gpt4all/2023_GPT4All_Technical_Report.pdf]
- GitHub: nomic-ai/gpt4al [https:/ /github.com/nomic-ai/gpt4all]
- Demo: GPT4All (unofficial). [https://huggingface.co/spaces/rishiraj/GPT4All]
- Model card: nomic-ai/gpt4all-lora · Hugging Face [https://huggingface.co/nomic-ai/gpt4all-lora ]
6.Raven RWKV
Raven RWKV 7B is an open source chat robot that is driven by the RWKV language model and generates The results are similar to ChatGPT. This model uses RNN, which can match the transformer in terms of quality and scalability, while being faster and saving VRAM. Raven is fine-tuned on Stanford Alpaca, code-alpaca, and more datasets.
Image from Raven RWKV 7B
Source:
- GitHub: BlinkDL/ChatRWKV [https://github.com /BlinkDL/ChatRWKV]
- Demo: Raven RWKV 7B [https://huggingface.co/spaces/BlinkDL/Raven-RWKV-7B]
- Model Card: BlinkDL/rwkv-4- raven [https://huggingface.co/BlinkDL/rwkv-4-raven]
7.OPT
OPT: The Open Pre-trained Transformer language model is not as powerful as ChatGPT, but it shows excellent capabilities in zero-shot and few-shot learning and stereotype bias analysis. It can also be integrated with Alpa, Colossal-AI, CTranslate2 and FasterTransformer for better results. NOTE: The reason it makes the list is its popularity, as it has 624,710 downloads per month in the text generation category.
Image from (arxiv.org)
Source:
- Research paper: "OPT: Open Pre-trained Transformer Language Models (arxiv.org)” [https://arxiv.org/abs/2205.01068]
- GitHub: facebookresearch/metaseq [https://github.com/facebookresearch/metaseq]
- Demo: A Watermark for LLMs [https://huggingface.co/spaces/tomg-group-umd/lm-watermarking]
- Model card: facebook/opt-1.3b [https://huggingface. co/facebook/opt-1.3b]
##8.Flan-T5-XXL
Flan-T5-XXL The T5 model is fine-tuned on the data set expressed in the form of instructions. Fine-tuning of the instructions greatly improved the performance of various model classes, such as PaLM, T5 and U-PaLM. The Flan-T5-XXL model is fine-tuned on more than 1000 additional tasks, covering more languages.- Research paper: “Scaling Instruction-Fine Tuned Language Models ” [https://arxiv.org/pdf/2210.11416.pdf]
- GitHub: google-research/t5x [https://github.com/google-research/t5x]
- Demo: Chat Llm Streaming [https://huggingface.co/spaces/olivierdehaene/chat-llm-streaming]
- Model card: google/flan-t5-xxl [https://huggingface.co/google /flan-t5-xxl?text=Q: ( False or not False or False ) is? A: Let's think step by step]
##SummaryThere are many open source large models to choose from. This article involves 8 of the more popular large models.
The above is the detailed content of Introducing eight free and open source large model solutions because ChatGPT and Bard are too expensive.. For more information, please follow other related articles on the PHP Chinese website!

Hot AI Tools

Undresser.AI Undress
AI-powered app for creating realistic nude photos

AI Clothes Remover
Online AI tool for removing clothes from photos.

Undress AI Tool
Undress images for free

Clothoff.io
AI clothes remover

Video Face Swap
Swap faces in any video effortlessly with our completely free AI face swap tool!

Hot Article

Hot Tools

Notepad++7.3.1
Easy-to-use and free code editor

SublimeText3 Chinese version
Chinese version, very easy to use

Zend Studio 13.0.1
Powerful PHP integrated development environment

Dreamweaver CS6
Visual web development tools

SublimeText3 Mac version
God-level code editing software (SublimeText3)

Hot Topics
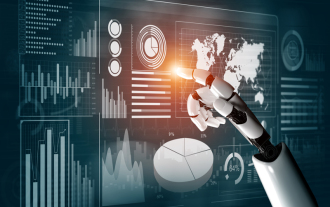
Text annotation is the work of corresponding labels or tags to specific content in text. Its main purpose is to provide additional information to the text for deeper analysis and processing, especially in the field of artificial intelligence. Text annotation is crucial for supervised machine learning tasks in artificial intelligence applications. It is used to train AI models to help more accurately understand natural language text information and improve the performance of tasks such as text classification, sentiment analysis, and language translation. Through text annotation, we can teach AI models to recognize entities in text, understand context, and make accurate predictions when new similar data appears. This article mainly recommends some better open source text annotation tools. 1.LabelStudiohttps://github.com/Hu
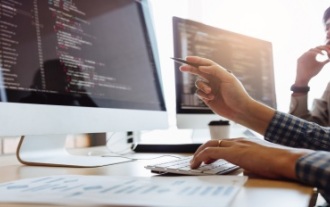
Image annotation is the process of associating labels or descriptive information with images to give deeper meaning and explanation to the image content. This process is critical to machine learning, which helps train vision models to more accurately identify individual elements in images. By adding annotations to images, the computer can understand the semantics and context behind the images, thereby improving the ability to understand and analyze the image content. Image annotation has a wide range of applications, covering many fields, such as computer vision, natural language processing, and graph vision models. It has a wide range of applications, such as assisting vehicles in identifying obstacles on the road, and helping in the detection and diagnosis of diseases through medical image recognition. . This article mainly recommends some better open source and free image annotation tools. 1.Makesens
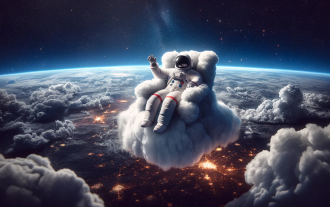
DALL-E 3 was officially introduced in September of 2023 as a vastly improved model than its predecessor. It is considered one of the best AI image generators to date, capable of creating images with intricate detail. However, at launch, it was exclus
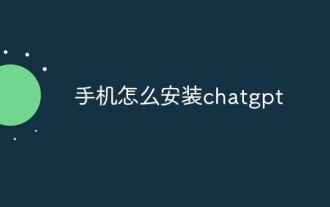
Installation steps: 1. Download the ChatGTP software from the ChatGTP official website or mobile store; 2. After opening it, in the settings interface, select the language as Chinese; 3. In the game interface, select human-machine game and set the Chinese spectrum; 4 . After starting, enter commands in the chat window to interact with the software.
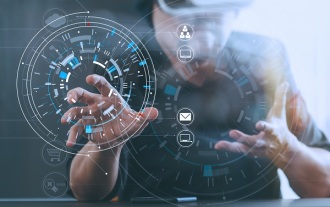
Face detection and recognition technology is already a relatively mature and widely used technology. Currently, the most widely used Internet application language is JS. Implementing face detection and recognition on the Web front-end has advantages and disadvantages compared to back-end face recognition. Advantages include reducing network interaction and real-time recognition, which greatly shortens user waiting time and improves user experience; disadvantages include: being limited by model size, the accuracy is also limited. How to use js to implement face detection on the web? In order to implement face recognition on the Web, you need to be familiar with related programming languages and technologies, such as JavaScript, HTML, CSS, WebRTC, etc. At the same time, you also need to master relevant computer vision and artificial intelligence technologies. It is worth noting that due to the design of the Web side
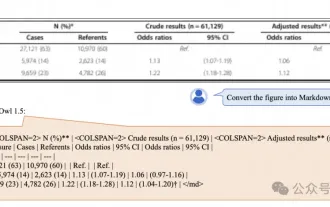
New SOTA for multimodal document understanding capabilities! Alibaba's mPLUG team released the latest open source work mPLUG-DocOwl1.5, which proposed a series of solutions to address the four major challenges of high-resolution image text recognition, general document structure understanding, instruction following, and introduction of external knowledge. Without further ado, let’s look at the effects first. One-click recognition and conversion of charts with complex structures into Markdown format: Charts of different styles are available: More detailed text recognition and positioning can also be easily handled: Detailed explanations of document understanding can also be given: You know, "Document Understanding" is currently An important scenario for the implementation of large language models. There are many products on the market to assist document reading. Some of them mainly use OCR systems for text recognition and cooperate with LLM for text processing.
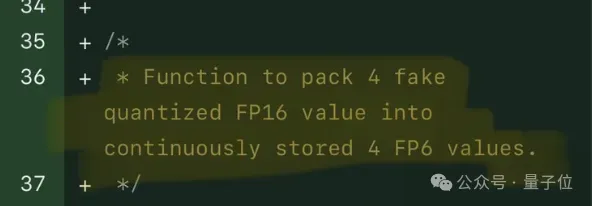
FP8 and lower floating point quantification precision are no longer the "patent" of H100! Lao Huang wanted everyone to use INT8/INT4, and the Microsoft DeepSpeed team started running FP6 on A100 without official support from NVIDIA. Test results show that the new method TC-FPx's FP6 quantization on A100 is close to or occasionally faster than INT4, and has higher accuracy than the latter. On top of this, there is also end-to-end large model support, which has been open sourced and integrated into deep learning inference frameworks such as DeepSpeed. This result also has an immediate effect on accelerating large models - under this framework, using a single card to run Llama, the throughput is 2.65 times higher than that of dual cards. one
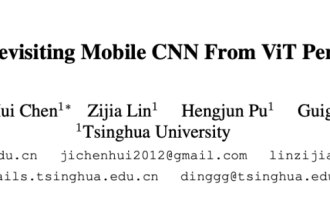
Paper address: https://arxiv.org/abs/2307.09283 Code address: https://github.com/THU-MIG/RepViTRepViT performs well in the mobile ViT architecture and shows significant advantages. Next, we explore the contributions of this study. It is mentioned in the article that lightweight ViTs generally perform better than lightweight CNNs on visual tasks, mainly due to their multi-head self-attention module (MSHA) that allows the model to learn global representations. However, the architectural differences between lightweight ViTs and lightweight CNNs have not been fully studied. In this study, the authors integrated lightweight ViTs into the effective
