


Pre-training requires no attention, and scaling to 4096 tokens is no problem, which is comparable to BERT.
Transformer, as an NLP pre-training model architecture, can effectively learn on large-scale unlabeled data. Research has proven that Transformer is the core architecture of NLP tasks since BERT.
Recent work has shown that the state space model (SSM) is a favorable competing architecture for modeling long-range sequences. SSM achieves state-of-the-art results on speech generation and Long Range Arena benchmarks, even outperforming the Transformer architecture. In addition to improving accuracy, the routing layer based on SSM will not exhibit quadratic complexity as the sequence length grows.
In this article, researchers from Cornell University, DeepMind and other institutions proposed Bidirectional Gated SSM (BiGS) for pre-training without attention. It mainly uses SSM routing is combined with an architecture based on multiplicative gating. The study found that SSM by itself performs poorly in pre-training for NLP, but when integrated into a multiplicative gated architecture, downstream accuracy improves.
Experiments show that BiGS is able to match the performance of BERT models when trained on the same data under controlled settings. With additional pretraining on longer instances, the model also maintains linear time when scaling the input sequence to 4096. Analysis shows that multiplicative gating is necessary and fixes some specific problems of SSM models on variable-length text inputs.
##Paper address: https://arxiv.org/pdf/2212.10544.pdf
Method IntroductionSSM connects the continuous input u (t) to the output y (t) through the following differential equation:
For discrete sequences, the SSM parameters are discretized, and the process can be approximated as:
Pre-training model architecture
Can SSM replace attention in pre-training? To answer this question, the study considered two different architectures, the stacked architecture (STACK) and the multiplicative gated architecture (GATED) shown in Figure 1.The stacked architecture with self-attention is equivalent to the BERT /transformer model, and the gated architecture is a bidirectional adaptation of the gated unit, which has also recently been used for unidirectional SSM. 2 sequence blocks (i.e., forward and backward SSM) with multiplicative gating are sandwiched in a feedforward layer. For a fair comparison, the size of the gated architecture is kept comparable to the stacked architecture.
Figure 1: Model variables. STACK is a standard transformer architecture, and GATED is based on gate control units. For the Routing component (dashed line), the study considers both bidirectional SSM (shown in the figure) and standard self-attention. Gated(X) represents element-wise multiplication. Pre-training Table 1 shows the main results of different pre-trained models on the GLUE benchmark. BiGS replicates BERT’s accuracy on token expansion. This result shows that SSM can replicate the accuracy of the pre-trained transformer model under such a computational budget. These results are significantly better than other non-attention-based pre-trained models. To achieve this accuracy, multiplicative gating is necessary. Without gating, the results of stacked SSM are significantly worse. To examine whether this advantage mainly comes from the use of gating, we trained an attention-based model using the GATE architecture; however, the results show that the model is actually less effective than BERT. Table 1: GLUE results. (Top) Comparison of different architectures and routing under control settings. See Figure 2 for details. (Bottom) reported comparable results for other non-attention pretrained models based on CNN, LSTM and FNet. Long-Form Task Table 2 results show that SSM can be combined with Longformer EncoderDecoder (LED) was compared to BART, however, and the results showed that it performed just as well or even better in remote tasks. Compared to the other two methods, SSM has much less pre-training data. Even though SSM does not need to approximate at these lengths, the long form is still important. Table 2: SCROLLS Encoder test results. The baseline models are both encoder-decoder models, one based on Longformer (LED) and the other based on BART. The input length is truncated. #Please see the original paper for more information. Experimental results
The above is the detailed content of Pre-training requires no attention, and scaling to 4096 tokens is no problem, which is comparable to BERT.. For more information, please follow other related articles on the PHP Chinese website!

Hot AI Tools

Undresser.AI Undress
AI-powered app for creating realistic nude photos

AI Clothes Remover
Online AI tool for removing clothes from photos.

Undress AI Tool
Undress images for free

Clothoff.io
AI clothes remover

Video Face Swap
Swap faces in any video effortlessly with our completely free AI face swap tool!

Hot Article

Hot Tools

Notepad++7.3.1
Easy-to-use and free code editor

SublimeText3 Chinese version
Chinese version, very easy to use

Zend Studio 13.0.1
Powerful PHP integrated development environment

Dreamweaver CS6
Visual web development tools

SublimeText3 Mac version
God-level code editing software (SublimeText3)

Hot Topics










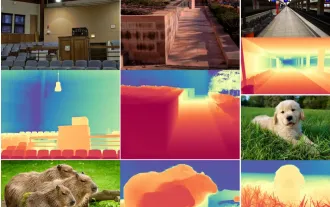
0.What does this article do? We propose DepthFM: a versatile and fast state-of-the-art generative monocular depth estimation model. In addition to traditional depth estimation tasks, DepthFM also demonstrates state-of-the-art capabilities in downstream tasks such as depth inpainting. DepthFM is efficient and can synthesize depth maps within a few inference steps. Let’s read about this work together ~ 1. Paper information title: DepthFM: FastMonocularDepthEstimationwithFlowMatching Author: MingGui, JohannesS.Fischer, UlrichPrestel, PingchuanMa, Dmytr
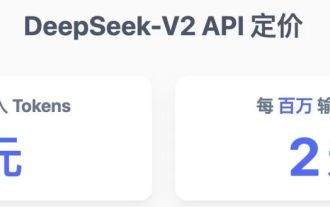
Imagine an artificial intelligence model that not only has the ability to surpass traditional computing, but also achieves more efficient performance at a lower cost. This is not science fiction, DeepSeek-V2[1], the world’s most powerful open source MoE model is here. DeepSeek-V2 is a powerful mixture of experts (MoE) language model with the characteristics of economical training and efficient inference. It consists of 236B parameters, 21B of which are used to activate each marker. Compared with DeepSeek67B, DeepSeek-V2 has stronger performance, while saving 42.5% of training costs, reducing KV cache by 93.3%, and increasing the maximum generation throughput to 5.76 times. DeepSeek is a company exploring general artificial intelligence
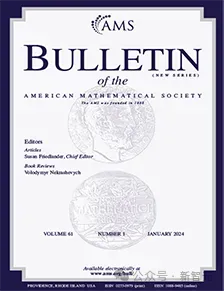
AI is indeed changing mathematics. Recently, Tao Zhexuan, who has been paying close attention to this issue, forwarded the latest issue of "Bulletin of the American Mathematical Society" (Bulletin of the American Mathematical Society). Focusing on the topic "Will machines change mathematics?", many mathematicians expressed their opinions. The whole process was full of sparks, hardcore and exciting. The author has a strong lineup, including Fields Medal winner Akshay Venkatesh, Chinese mathematician Zheng Lejun, NYU computer scientist Ernest Davis and many other well-known scholars in the industry. The world of AI has changed dramatically. You know, many of these articles were submitted a year ago.
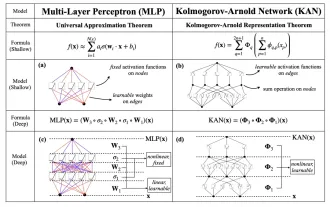
Earlier this month, researchers from MIT and other institutions proposed a very promising alternative to MLP - KAN. KAN outperforms MLP in terms of accuracy and interpretability. And it can outperform MLP running with a larger number of parameters with a very small number of parameters. For example, the authors stated that they used KAN to reproduce DeepMind's results with a smaller network and a higher degree of automation. Specifically, DeepMind's MLP has about 300,000 parameters, while KAN only has about 200 parameters. KAN has a strong mathematical foundation like MLP. MLP is based on the universal approximation theorem, while KAN is based on the Kolmogorov-Arnold representation theorem. As shown in the figure below, KAN has
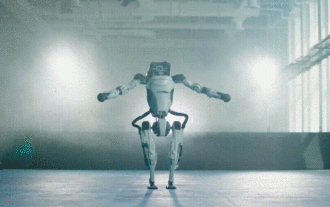
Boston Dynamics Atlas officially enters the era of electric robots! Yesterday, the hydraulic Atlas just "tearfully" withdrew from the stage of history. Today, Boston Dynamics announced that the electric Atlas is on the job. It seems that in the field of commercial humanoid robots, Boston Dynamics is determined to compete with Tesla. After the new video was released, it had already been viewed by more than one million people in just ten hours. The old people leave and new roles appear. This is a historical necessity. There is no doubt that this year is the explosive year of humanoid robots. Netizens commented: The advancement of robots has made this year's opening ceremony look like a human, and the degree of freedom is far greater than that of humans. But is this really not a horror movie? At the beginning of the video, Atlas is lying calmly on the ground, seemingly on his back. What follows is jaw-dropping
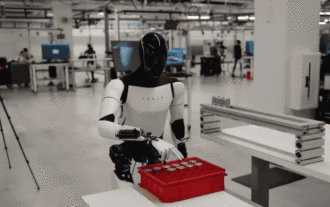
The latest video of Tesla's robot Optimus is released, and it can already work in the factory. At normal speed, it sorts batteries (Tesla's 4680 batteries) like this: The official also released what it looks like at 20x speed - on a small "workstation", picking and picking and picking: This time it is released One of the highlights of the video is that Optimus completes this work in the factory, completely autonomously, without human intervention throughout the process. And from the perspective of Optimus, it can also pick up and place the crooked battery, focusing on automatic error correction: Regarding Optimus's hand, NVIDIA scientist Jim Fan gave a high evaluation: Optimus's hand is the world's five-fingered robot. One of the most dexterous. Its hands are not only tactile
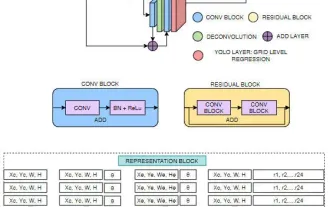
Target detection is a relatively mature problem in autonomous driving systems, among which pedestrian detection is one of the earliest algorithms to be deployed. Very comprehensive research has been carried out in most papers. However, distance perception using fisheye cameras for surround view is relatively less studied. Due to large radial distortion, standard bounding box representation is difficult to implement in fisheye cameras. To alleviate the above description, we explore extended bounding box, ellipse, and general polygon designs into polar/angular representations and define an instance segmentation mIOU metric to analyze these representations. The proposed model fisheyeDetNet with polygonal shape outperforms other models and simultaneously achieves 49.5% mAP on the Valeo fisheye camera dataset for autonomous driving
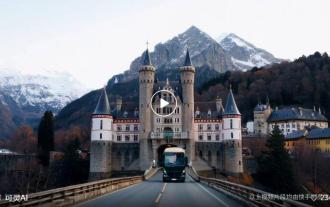
What? Is Zootopia brought into reality by domestic AI? Exposed together with the video is a new large-scale domestic video generation model called "Keling". Sora uses a similar technical route and combines a number of self-developed technological innovations to produce videos that not only have large and reasonable movements, but also simulate the characteristics of the physical world and have strong conceptual combination capabilities and imagination. According to the data, Keling supports the generation of ultra-long videos of up to 2 minutes at 30fps, with resolutions up to 1080p, and supports multiple aspect ratios. Another important point is that Keling is not a demo or video result demonstration released by the laboratory, but a product-level application launched by Kuaishou, a leading player in the short video field. Moreover, the main focus is to be pragmatic, not to write blank checks, and to go online as soon as it is released. The large model of Ke Ling is already available in Kuaiying.
