


How to create a multi-level index (MultiIndex) using Python's pandas library?
Introduction
pd.MultiIndex, an index with multiple levels. Through multi-level indexes, we can operate the data of the entire index group. This article mainly introduces 6 ways to create multi-level indexes in Pandas:
pd.MultiIndex.from_arrays(): Multi-dimensional arrays are used as parameters, high dimensions specify high-level indexes, and low dimensions specify low-level indexes. index.
pd.MultiIndex.from_tuples(): List of tuples as argument, each tuple specifying each index (high-dimensional and low-dimensional index).
pd.MultiIndex.from_product(): A list of iterable objects as parameters, created based on the Cartesian product (pairwise combination of elements) of multiple iterable object elements index.
pd.MultiIndex.from_frame: directly generated based on the existing data frame
groupby(): obtained through data grouping statistics
pivot_table(): Generate a pivot table to get
pd.MultiIndex.from_arrays()
In [1] :
import pandas as pd import numpy as np
is generated through an array, usually specifying the elements in the list:
In [2]:
# 列表元素是字符串和数字 array1 = [["xiaoming","guanyu","zhangfei"], [22,25,27] ] m1 = pd.MultiIndex.from_arrays(array1) m1
Out[2]:
MultiIndex([('xiaoming', 22), ( 'guanyu', 25), ('zhangfei', 27)], )
In [3]:
type(m1) # 查看数据类型
Use the type function to check the data type and find that it is indeed: MultiIndex
Out[3]:
pandas.core.indexes.multi.MultiIndex
is created At the same time, you can specify the name of each level:
In [4]:
# 列表元素全是字符串 array2 = [["xiaoming","guanyu","zhangfei"], ["male","male","female"] ] m2 = pd.MultiIndex.from_arrays( array2, # 指定姓名和性别 names=["name","sex"]) m2
Out[4]:
MultiIndex([('xiaoming', 'male'), ( 'guanyu', 'male'), ('zhangfei', 'female')], names=['name', 'sex'])
The following example generates an index of three levels and Specify name:
In [5]:
array3 = [["xiaoming","guanyu","zhangfei"], ["male","male","female"], [22,25,27] ] m3 = pd.MultiIndex.from_arrays( array3, names=["姓名","性别","年龄"]) m3
Out[5]:
MultiIndex([('xiaoming', 'male', 22), ( 'guanyu', 'male', 25), ('zhangfei', 'female', 27)], names=['姓名', '性别', '年龄'])
pd.MultiIndex.from_tuples()
Through tuples To generate multi-level indexes in the form:
In [6]:
# 元组的形式 array4 = (("xiaoming","guanyu","zhangfei"), (22,25,27) ) m4 = pd.MultiIndex.from_arrays(array4) m4
Out[6]:
MultiIndex([('xiaoming', 22), ( 'guanyu', 25), ('zhangfei', 27)], )
In [7]:
# 元组构成的3层索引 array5 = (("xiaoming","guanyu","zhangfei"), ("male","male","female"), (22,25,27)) m5 = pd.MultiIndex.from_arrays(array5) m5
Out [7]:
MultiIndex([('xiaoming', 'male', 22), ( 'guanyu', 'male', 25), ('zhangfei', 'female', 27)], )
Lists and tuples can be mixed.
The outermost layer is the list
All are tuples
In [8]:
array6 = [("xiaoming","guanyu","zhangfei"), ("male","male","female"), (18,35,27) ] # 指定名字 m6 = pd.MultiIndex.from_arrays(array6,names=["姓名","性别","年龄"]) m6
Out[8]:
MultiIndex([('xiaoming', 'male', 18), ( 'guanyu', 'male', 35), ('zhangfei', 'female', 27)], names=['姓名', '性别', '年龄'] # 指定名字 )
pd.MultiIndex.from_product()
Use a list of iterable objects as parameters to create an index based on the Cartesian product of multiple iterable object elements (a pairwise combination of elements).
In Python, we use the isinstance()
function to determine whether a python object is iterable:
# 导入 collections 模块的 Iterable 对比对象 from collections import Iterable
Through the above examples we summarize: Common strings, lists, sets, tuples, and dictionaries are all iterable objects
The following examples are given to illustrate:
In [18 ]:
names = ["xiaoming","guanyu","zhangfei"] numbers = [22,25] m7 = pd.MultiIndex.from_product( [names, numbers], names=["name","number"]) # 指定名字 m7
Out[18]:
MultiIndex([('xiaoming', 22), ('xiaoming', 25), ( 'guanyu', 22), ( 'guanyu', 25), ('zhangfei', 22), ('zhangfei', 25)], names=['name', 'number'])
In [19]:
# 需要展开成列表形式 strings = list("abc") lists = [1,2] m8 = pd.MultiIndex.from_product( [strings, lists], names=["alpha","number"]) m8
Out[19]:
MultiIndex([('a', 1), ('a', 2), ('b', 1), ('b', 2), ('c', 1), ('c', 2)], names=['alpha', 'number'])
In [20]:
# 使用元组形式 strings = ("a","b","c") lists = [1,2] m9 = pd.MultiIndex.from_product( [strings, lists], names=["alpha","number"]) m9
Out[20]:
MultiIndex([('a', 1), ('a', 2), ('b', 1), ('b', 2), ('c', 1), ('c', 2)], names=['alpha', 'number'])
In [21]:
# 使用range函数 strings = ("a","b","c") # 3个元素 lists = range(3) # 0,1,2 3个元素 m10 = pd.MultiIndex.from_product( [strings, lists], names=["alpha","number"]) m10
Out[21]:
MultiIndex([('a', 0), ('a', 1), ('a', 2), ('b', 0), ('b', 1), ('b', 2), ('c', 0), ('c', 1), ('c', 2)], names=['alpha', 'number'])
In [22]:
# 使用range函数 strings = ("a","b","c") list1 = range(3) # 0,1,2 list2 = ["x","y"] m11 = pd.MultiIndex.from_product( [strings, list1, list2], names=["name","l1","l2"] ) m11 # 总个数 3*3*2=18
The total number is ``332=18`:
Out[22]:
MultiIndex([('a', 0, 'x'), ('a', 0, 'y'), ('a', 1, 'x'), ('a', 1, 'y'), ('a', 2, 'x'), ('a', 2, 'y'), ('b', 0, 'x'), ('b', 0, 'y'), ('b', 1, 'x'), ('b', 1, 'y'), ('b', 2, 'x'), ('b', 2, 'y'), ('c', 0, 'x'), ('c', 0, 'y'), ('c', 1, 'x'), ('c', 1, 'y'), ('c', 2, 'x'), ('c', 2, 'y')], names=['name', 'l1', 'l2'])
pd.MultiIndex.from_frame()
By current Some DataFrames directly generate multi-level indexes:
df = pd.DataFrame({"name":["xiaoming","guanyu","zhaoyun"], "age":[23,39,34], "sex":["male","male","female"]}) df
The multi-level indexes are directly generated, and the names are the column fields of the existing data frame:
In [24]:
pd.MultiIndex.from_frame(df)
Out[24]:
MultiIndex([('xiaoming', 23, 'male'), ( 'guanyu', 39, 'male'), ( 'zhaoyun', 34, 'female')], names=['name', 'age', 'sex'])
Specify the name through the names parameter:
In [25]:
# 可以自定义名字 pd.MultiIndex.from_frame(df,names=["col1","col2","col3"])
Out[ 25]:
MultiIndex([('xiaoming', 23, 'male'), ( 'guanyu', 39, 'male'), ( 'zhaoyun', 34, 'female')], names=['col1', 'col2', 'col3'])
groupby()
is calculated through the grouping function of the groupby function:
In [26]:
df1 = pd.DataFrame({"col1":list("ababbc"), "col2":list("xxyyzz"), "number1":range(90,96), "number2":range(100,106)}) df1
Out[26] :
df2 = df1.groupby(["col1","col2"]).agg({"number1":sum, "number2":np.mean}) df2
View the index of the data:
In [28]:
df2.index
Out [28]:
MultiIndex([('a', 'x'), ('a', 'y'), ('b', 'x'), ('b', 'y'), ('b', 'z'), ('c', 'z')], names=['col1', 'col2'])
pivot_table()
Obtained through the data pivot function:
In [29]:
df3 = df1.pivot_table(values=["col1","col2"],index=["col1","col2"]) df3
In [30]:
df3.index
Out[30]:
MultiIndex([('a', 'x'), ('a', 'y'), ('b', 'x'), ('b', 'y'), ('b', 'z'), ('c', 'z')], names=['col1', 'col2'])
The above is the detailed content of How to create a multi-level index (MultiIndex) using Python's pandas library?. For more information, please follow other related articles on the PHP Chinese website!

Hot AI Tools

Undresser.AI Undress
AI-powered app for creating realistic nude photos

AI Clothes Remover
Online AI tool for removing clothes from photos.

Undress AI Tool
Undress images for free

Clothoff.io
AI clothes remover

Video Face Swap
Swap faces in any video effortlessly with our completely free AI face swap tool!

Hot Article

Hot Tools

Notepad++7.3.1
Easy-to-use and free code editor

SublimeText3 Chinese version
Chinese version, very easy to use

Zend Studio 13.0.1
Powerful PHP integrated development environment

Dreamweaver CS6
Visual web development tools

SublimeText3 Mac version
God-level code editing software (SublimeText3)

Hot Topics
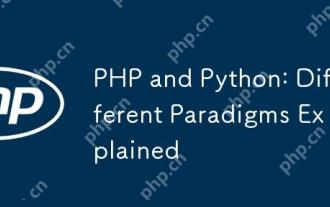
PHP is mainly procedural programming, but also supports object-oriented programming (OOP); Python supports a variety of paradigms, including OOP, functional and procedural programming. PHP is suitable for web development, and Python is suitable for a variety of applications such as data analysis and machine learning.
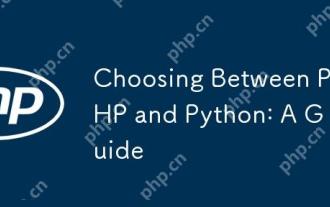
PHP is suitable for web development and rapid prototyping, and Python is suitable for data science and machine learning. 1.PHP is used for dynamic web development, with simple syntax and suitable for rapid development. 2. Python has concise syntax, is suitable for multiple fields, and has a strong library ecosystem.
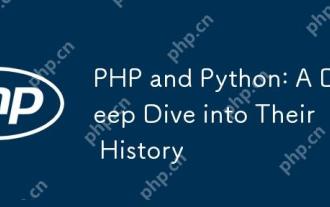
PHP originated in 1994 and was developed by RasmusLerdorf. It was originally used to track website visitors and gradually evolved into a server-side scripting language and was widely used in web development. Python was developed by Guidovan Rossum in the late 1980s and was first released in 1991. It emphasizes code readability and simplicity, and is suitable for scientific computing, data analysis and other fields.
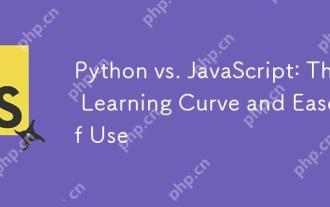
Python is more suitable for beginners, with a smooth learning curve and concise syntax; JavaScript is suitable for front-end development, with a steep learning curve and flexible syntax. 1. Python syntax is intuitive and suitable for data science and back-end development. 2. JavaScript is flexible and widely used in front-end and server-side programming.
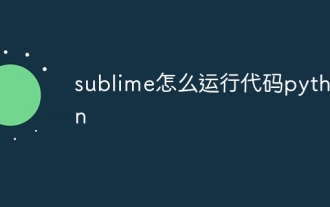
To run Python code in Sublime Text, you need to install the Python plug-in first, then create a .py file and write the code, and finally press Ctrl B to run the code, and the output will be displayed in the console.
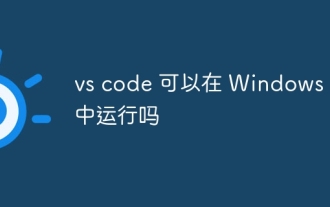
VS Code can run on Windows 8, but the experience may not be great. First make sure the system has been updated to the latest patch, then download the VS Code installation package that matches the system architecture and install it as prompted. After installation, be aware that some extensions may be incompatible with Windows 8 and need to look for alternative extensions or use newer Windows systems in a virtual machine. Install the necessary extensions to check whether they work properly. Although VS Code is feasible on Windows 8, it is recommended to upgrade to a newer Windows system for a better development experience and security.
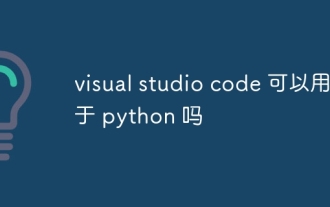
VS Code can be used to write Python and provides many features that make it an ideal tool for developing Python applications. It allows users to: install Python extensions to get functions such as code completion, syntax highlighting, and debugging. Use the debugger to track code step by step, find and fix errors. Integrate Git for version control. Use code formatting tools to maintain code consistency. Use the Linting tool to spot potential problems ahead of time.
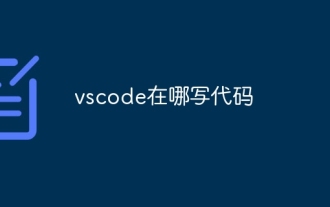
Writing code in Visual Studio Code (VSCode) is simple and easy to use. Just install VSCode, create a project, select a language, create a file, write code, save and run it. The advantages of VSCode include cross-platform, free and open source, powerful features, rich extensions, and lightweight and fast.
